Quantifying Distances Between Clusters with Elliptical or Non-Elliptical Shapes
arxiv(2022)
摘要
Finite mixture models that allow for a broad range of potentially non-elliptical cluster distributions is an emerging methodological field. Such methods allow for the shape of the clusters to match the natural heterogeneity of the data, rather than forcing a series of elliptical clusters. These methods are highly relevant for clustering continuous non-normal data - a common occurrence with objective data that are now routinely captured in health research. However, interpreting and comparing such models - especially with regards to whether they produce meaningful clusters that are reasonably well separated - is non-trivial. We summarize several measures that can succinctly quantify the multivariate distance between two clusters, regardless of the cluster distribution, and suggest practical computational tools. Through a simulation study, we evaluate these measures across three scenarios that allow for clusters to differ in mean, scale, and rotation. We then demonstrate our approaches using physiological responses to emotional imagery captured as part of the Transdiagnostic Anxiety Study, a large-scale study of anxiety disorder spectrum patients and control participants. Finally, we synthesize findings to provide guidance on how to use distance measures in clustering applications.
更多查看译文
关键词
clusters,distances,non-elliptical
AI 理解论文
溯源树
样例
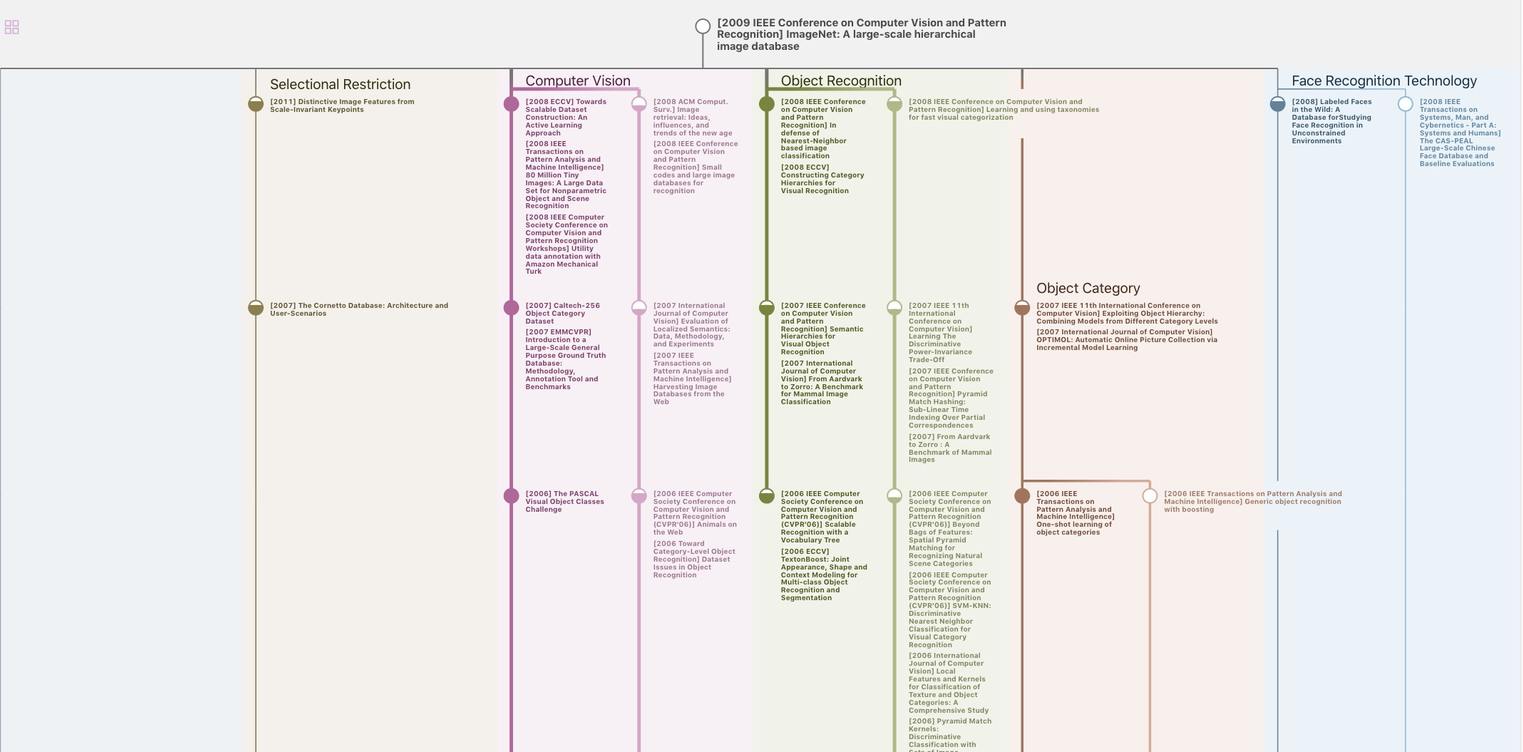
生成溯源树,研究论文发展脉络
Chat Paper
正在生成论文摘要