SLICED INVERSE REGRESSION IN METRIC SPACES
STATISTICA SINICA(2022)
摘要
In this article, we propose a general nonlinear sufficient dimension reduc-tion (SDR) framework when both the predictor and the response lie in some general metric spaces. We construct reproducing kernel Hilbert spaces with kernels that are fully determined by the distance functions of the metric spaces, and then leverage the inherent structures of these spaces to define a nonlinear SDR framework. We adapt the classical sliced inverse regression within this framework for the metric space data. Next we build an estimator based on the corresponding linear opera-tors, and show that it recovers the regression information in an unbiased manner. We derive the estimator at both the operator level and under a coordinate system, and establish its convergence rate. Lastly, we illustrate the proposed method using synthetic and real data sets that exhibit non-Euclidean geometry.
更多查看译文
关键词
Covariance operator, metric space, reproducing kernel Hilbert space, sliced inverse regression, sufficient dimension reduction
AI 理解论文
溯源树
样例
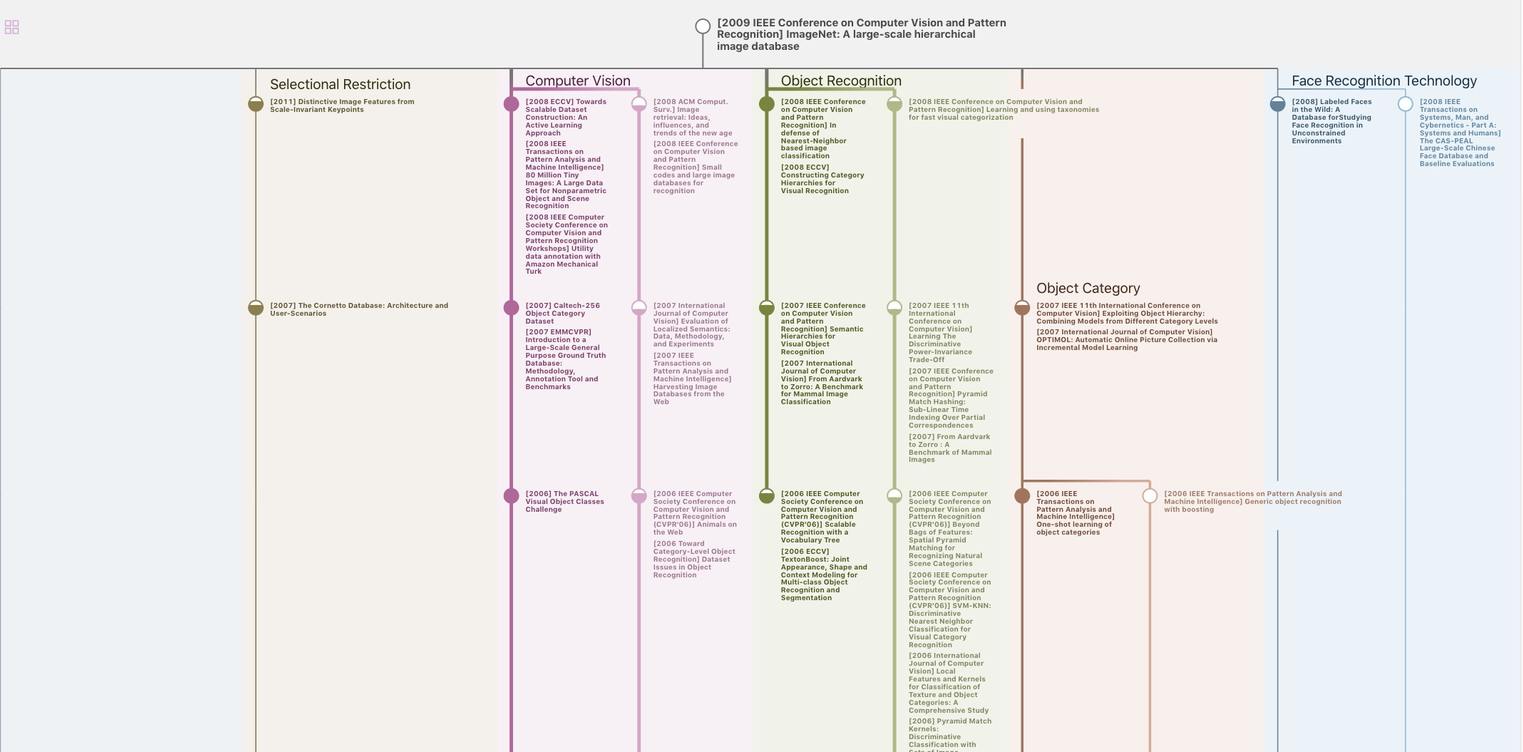
生成溯源树,研究论文发展脉络
Chat Paper
正在生成论文摘要