EFFGAN: Ensembles of Fine-Tuned Federated GANs
arxiv(2022)
Abstract
Generative adversarial networks have proven to be a powerful tool for learning complex and high-dimensional data distributions, but issues such as mode collapse have been shown to make it difficult to train them. This is an even harder problem when the data is decentralized over several clients in a federated learning setup, as problems such as client drift and non-iid data make it hard for federated averaging to converge. In this work, we study the task of how to learn a data distribution when training data is heterogeneously decentralized over clients and cannot be shared. Our goal is to sample from this distribution centrally, while the data never leaves the clients. We show using standard benchmark image datasets that existing approaches fail in this setting, experiencing so-called client drift when the local number of epochs becomes to large. We thus propose a novel approach we call EFFGAN: Ensembles of fine-tuned federated GANs. Being an ensemble of local expert generators, EFFGAN is able to learn the data distribution over all clients and mitigate client drift. It is able to train with a large number of local epochs, making it more communication efficient than previous works.
MoreTranslated text
AI Read Science
Must-Reading Tree
Example
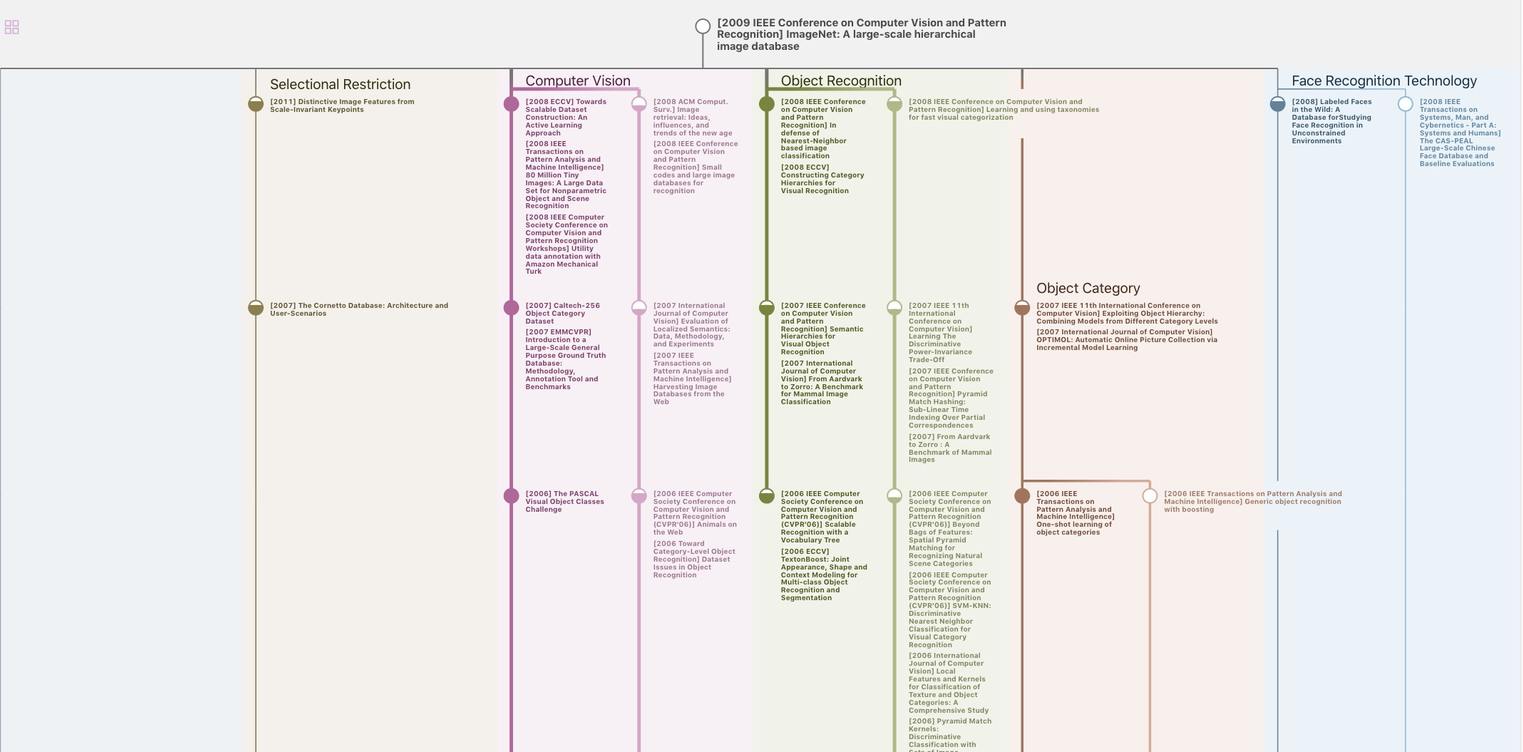
Generate MRT to find the research sequence of this paper
Chat Paper
Summary is being generated by the instructions you defined