Criticality-Guided Deep Reinforcement Learning for Motion Planning
2021 China Automation Congress (CAC)(2021)
摘要
Real-time and efficient collision avoidance is still challenging for mobile robots running in dynamic and crowded environments. Recent research shows that deep reinforcement learning (DRL) provides a framework to plan collision-free trajectories efficiently. However, most of the current DRL-based methods focus on a fixed number of obstacles in the environments, which limits their applications. In this paper, we propose a learning-based model, Crit-LSTM-DRL, for a robot moving in environments with a variable number of obstacles. It combines an LSTM (Long Short-Term Memory) model and a value-based DRL model. Given the states of a set of obstacles, Crit-LSTM-DRL first sorts the obstacles according to their possible collision time to the robot and then feeds to the LSTM model to generate a fixed-size hidden state. Then, the value-based DRL model takes the hidden state and robot state as input to compute the value. Hence, at any time step, an action is selected that maximizes the value function defined in the DRL framework. Finally, we compare the performance of Crit-LSTM-DRL with a state-of-the-art DRL-based planning method that aims to deal with a variable number of obstacles. The simulation results show that the three models of Crit-LSTM-DRL can improve the success rate by 4%, 20.1%, and 3.8%, and reduce the collision rate by 35.5%, 75%, and 66.7%, respectively.
更多查看译文
关键词
Motion Planning,Collision Avoidance,Obstacle Criticality,Deep Reinforcement Learning
AI 理解论文
溯源树
样例
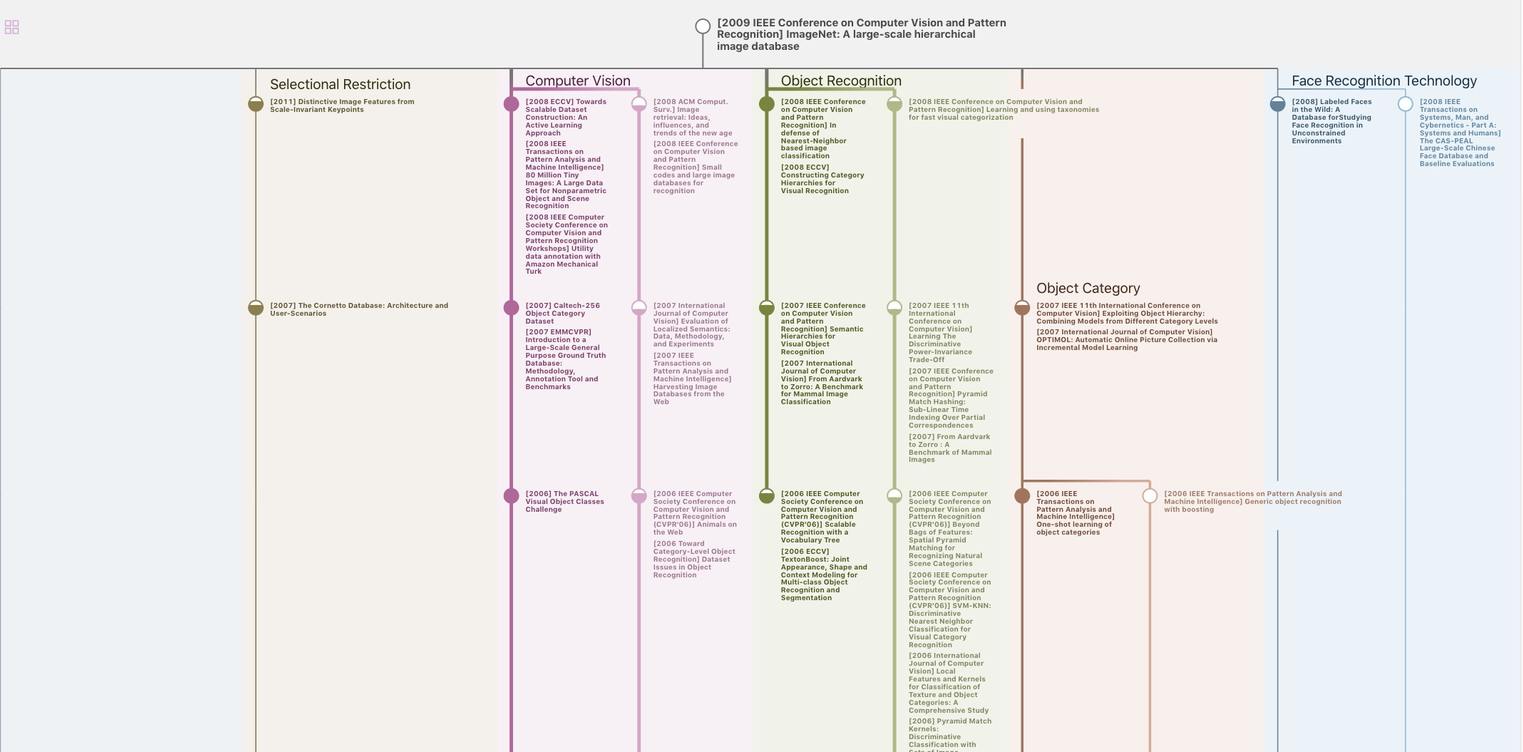
生成溯源树,研究论文发展脉络
Chat Paper
正在生成论文摘要