A lightweight ensemble discriminator for Generative Adversarial Networks
Knowledge-Based Systems(2022)
摘要
While Generative Adversarial Networks (GANs) have brought immense success in various content-generation tasks, they still face enormous challenges in generating high-quality visually realistic images because of the model collapse or instability during GAN training. One common accepted explanation for the model collapse and instability is that the learning signal provided by the discriminator to the generator become inadequate when the discriminator overconcentrates on the most discriminative difference between real and synthetic images and ignores the less discriminative parts. To this end, we propose a lightweight ensemble discriminator to evaluate the generator from multi-perspective. Borrowing the insights from ensemble learning, several auxiliary discriminators are embedded into one deep model. A novel ensemble loss function is designed to promote the complementariness within the ensemble and train the whole framework in an end-to-end manner. Extensive experiments on datasets of varying resolutions and data sizes prove significant performance improvements over the state-of-the-art GANs. The proposed method can be easily embedded into various GAN frameworks and combined with different loss functions.
更多查看译文
关键词
Generative Adversarial Network,Ensemble discriminator,Mode collapse,Instability,Image generation
AI 理解论文
溯源树
样例
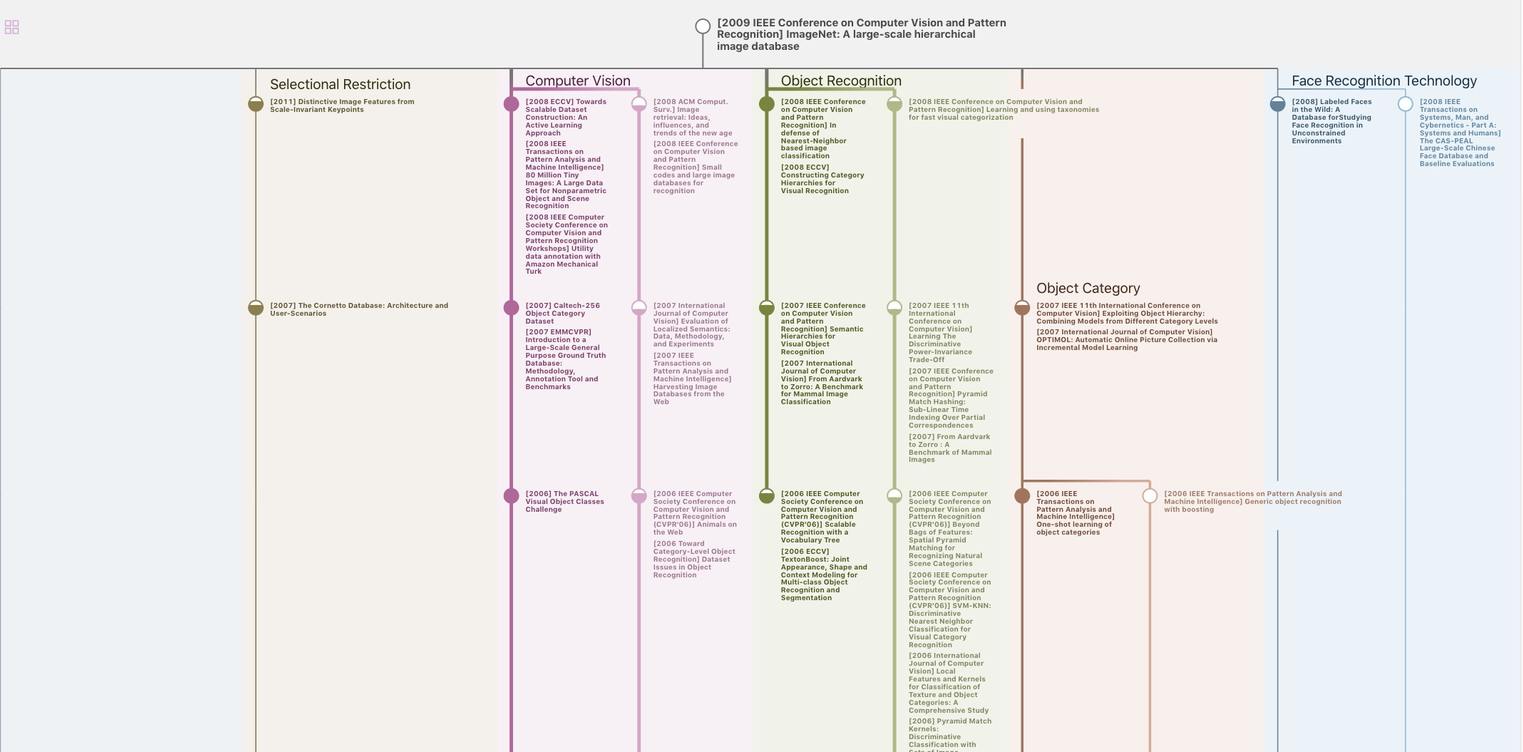
生成溯源树,研究论文发展脉络
Chat Paper
正在生成论文摘要