A nearest‐neighbour Gaussian process spatial factor model for censored, multi‐depth geochemical data
Applied statistics(2022)
摘要
We investigate the relationships between local environmental variables and the geochemical composition of the Earth in a region spanning over 26,000 km2 in the lower South Island of New Zealand. Part of the Southland–South Otago geochemical baseline survey—a pilot study pre-empting roll-out across the country—the data comprise the measurements of 59 chemical trace elements, each at two depth prescriptions, at several hundred spatial sites. We demonstrate construction of a hierarchical spatial factor model that captures inter-depth dependency; handles imputation of left-censored readings in a statistically principled manner; and exploits sparse approximations to Gaussian processes to deliver inference. The voluminous results provide a novel impression of the underlying processes and are presented graphically via simple web-based applications. These both confirm existing knowledge and provide a basis from which new research hypotheses in geochemistry might be formed.
更多查看译文
关键词
Bayesian model, geochemistry, geostatistics, multivariate outcome, spatial prediction
AI 理解论文
溯源树
样例
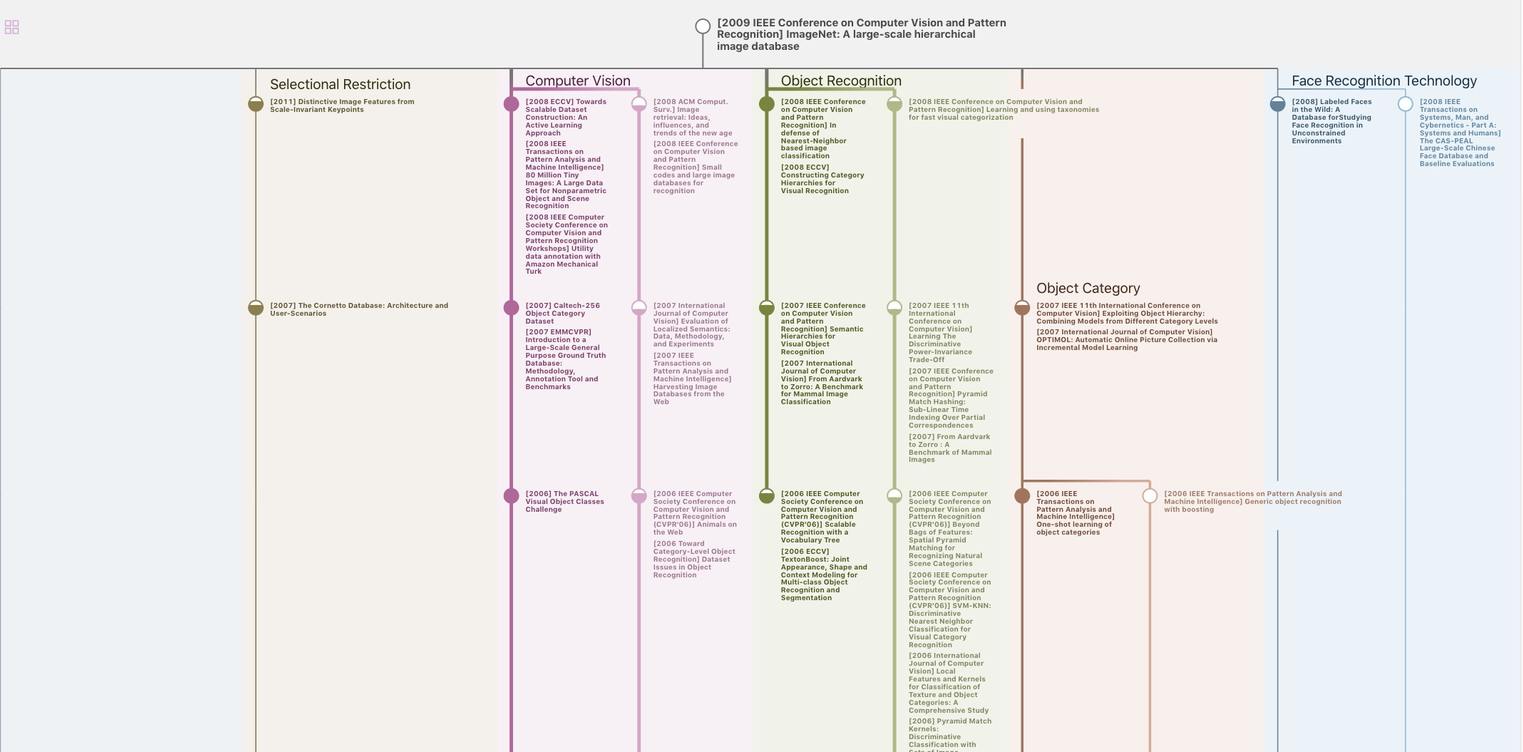
生成溯源树,研究论文发展脉络
Chat Paper
正在生成论文摘要