An Ultra-Energy-Efficient and High Accuracy ECG Classification Processor With SNN Inference Assisted by On-Chip ANN Learning
IEEE Transactions on Biomedical Circuits and Systems(2022)
摘要
The ECG classification processor is a key component in wearable intelligent ECG monitoring devices which monitor the ECG signals in real time and detect the abnormality automatically. The state-of-the-art ECG classification processors for wearable intelligent ECG monitoring devices are faced with two challenges, including ultra-low energy consumption demand and high classification accuracy demand against patient-to-patient variability. To address the above two challenges, in this work, an ultra-energy-efficient ECG classification processor with high classification accuracy is proposed. Several design techniques have been proposed, including a reconfigurable SNN/ANN inference architecture for reducing energy consumption while maintaining classification accuracy, a reconfigurable on-chip learning architecture for improving the classification accuracy against patent-to-patient variability, and a dual-purpose binary encoding scheme of ECG heartbeats for further reducing the energy consumption. Fabricated with a 28nm CMOS technology, the proposed design consumes extremely low classification energy (0.3 mu J) while achieving high classification accuracy (97.36%) against patient-to-patient variability, outperforming several state-of-the-art designs.
更多查看译文
关键词
Electrocardiography,Neurons,Computational modeling,Biological neural networks,Monitoring,Energy consumption,Power demand,ECG,processor,SNN,ANN,on-chip learning
AI 理解论文
溯源树
样例
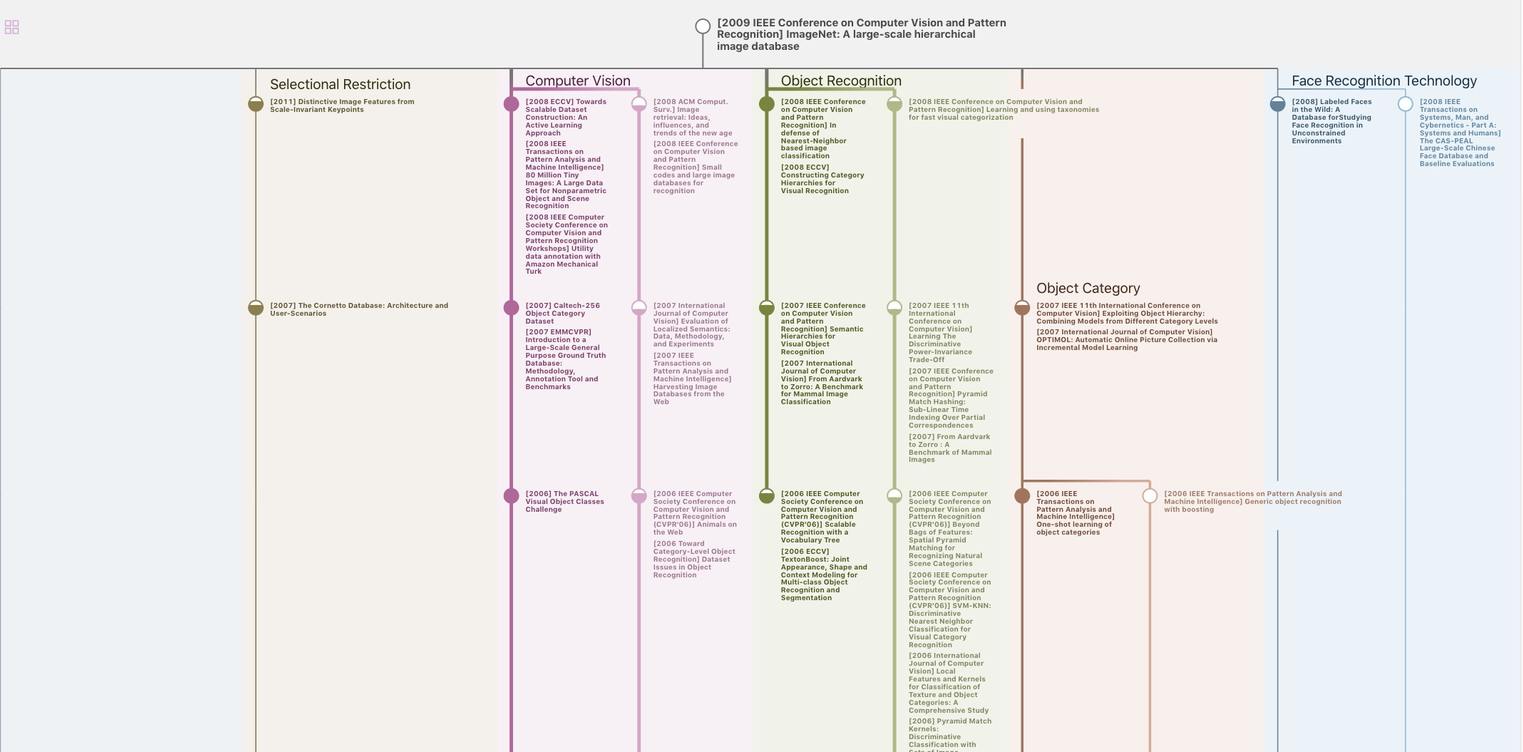
生成溯源树,研究论文发展脉络
Chat Paper
正在生成论文摘要