A Deep Learning Model Established for Evaluating Lid Margin Signs with Colour Anterior Segment Photography
Eye(2022)
摘要
Objectives To evaluate the feasibility of applying a deep learning model to identify lid margin signs from colour anterior segment photography. Methods We collected a total of 832 colour anterior segment photographs from 428 dry eye patients. Eight lid margin signs were labelled by human ophthalmologists. Eight deep learning models were constructed based on VGGNet-13 and trained to identify lid margin signs. Sensitivity, specificity, receiver operative characteristic (ROC) curves and area under the curve (AUC) were applied to evaluate the models. Results The AUC for rounding of posterior lid margin was 0.979 and was 0.977 and 0.980 for lid margin irregularity and vascularization. For hyperkeratinization, the AUC was 0.964. The AUCs for meibomian gland orifice (MGO) retroplacement and plugging were 0.963 and 0.968. For the mucocutaneous junction (MCJ) anteroplacement and retroplacement model, the AUCs were 0.950 and 0.978. The sensitivity and specificity for rounding of posterior lid margin were 0.974 and 0.921. For irregularity, the sensitivity and specificity were 0.930 and 0.938, and those for vascularization were 0.923 and 0.961. The hyperkeratinization model achieved a sensitivity and specificity of 0.889 and 0.948. The model identifying MGO plugging and retroplacement achieved a sensitivity of 0.979 and 0.909 with a specificity of 0.867 and 0.967. The sensitivity of MCJ anteroplacement and retroplacement were 0.875/0.969, with a specificity of 0.966/0.888. Conclusions The deep learning model could identify lid margin signs with high sensitivity and specificity. The study provided the potentiality of applying artificial intelligence in lid margin evaluation to assist dry eye decision-making.
更多查看译文
AI 理解论文
溯源树
样例
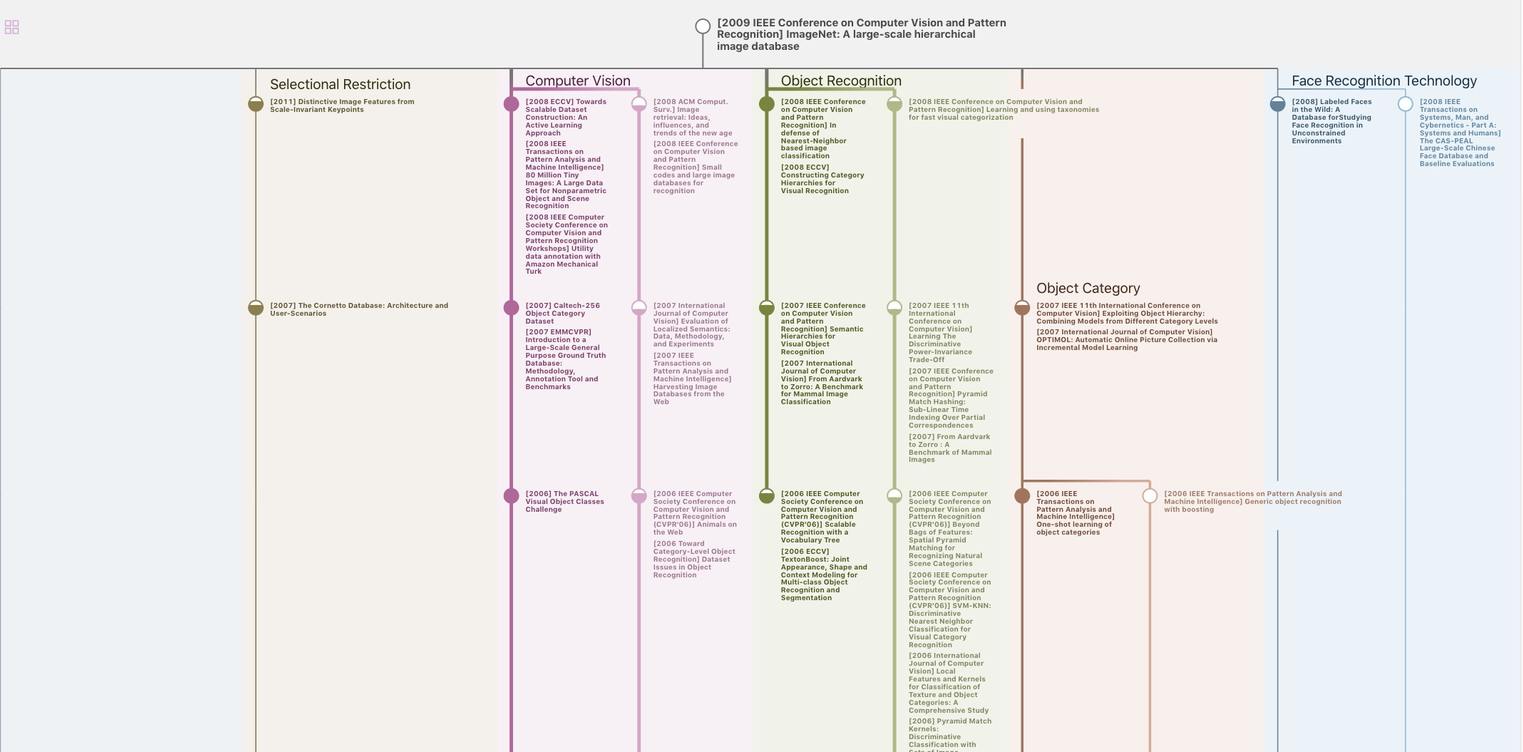
生成溯源树,研究论文发展脉络
Chat Paper
正在生成论文摘要