Development of a Machine Learning Model to Predict Non-Durable Response to Anti-TNF Therapy in Crohn's Disease Using Transcriptome Imputed from Genotypes
JOURNAL OF PERSONALIZED MEDICINE(2022)
摘要
Almost half of patients show no primary or secondary response to monoclonal anti-tumor necrosis factor alpha (anti-TNF) antibody treatment for inflammatory bowel disease (IBD). Thus, the exact mechanisms of a non-durable response (NDR) remain inadequately defined. We used our genome-wide genotype data to impute expression values as features in training machine learning models to predict a NDR. Blood samples from various IBD cohorts were used for genotyping with the Korea Biobank Array. A total of 234 patients with Crohn's disease (CD) who received their first anti-TNF therapy were enrolled. The expression profiles of 6294 genes in whole-blood tissue imputed from the genotype data were combined with clinical parameters to train a logistic model to predict the NDR. The top two and three most significant features were genetic features (DPY19L3, GSTT1, and NUCB1), not clinical features. The logistic regression of the NDR vs. DR status in our cohort by the imputed expression levels showed that the beta coefficients were positive for DPY19L3 and GSTT1, and negative for NUCB1, concordant with the known eQTL information. Machine learning models using imputed gene expression features effectively predicted NDR to anti-TNF agents in patients with CD.
更多查看译文
关键词
genotype, genetic features, anti-TNF, Crohn's disease
AI 理解论文
溯源树
样例
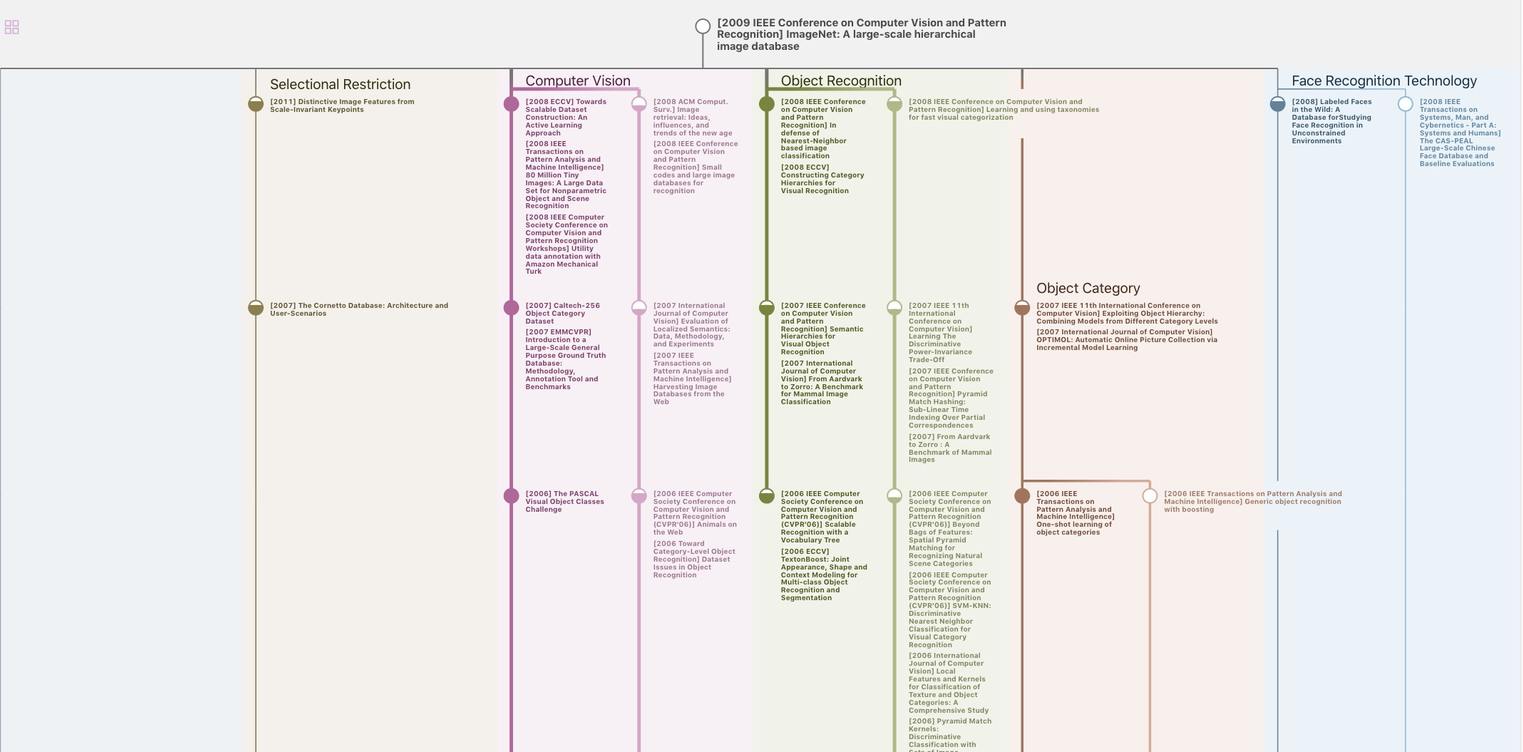
生成溯源树,研究论文发展脉络
Chat Paper
正在生成论文摘要