Fast Noninvasive Morphometric Characterization of Free Human Sperms Using Deep Learning
Microscopy and microanalysis(2022)
摘要
The selection of high-quality sperms is critical to intracytoplasmic sperm injection, which accounts for 70–80% of in vitro fertilization (IVF) treatments. So far, sperm screening is usually performed manually by clinicians. However, the performance of manual screening is limited in its objectivity, consistency, and efficiency. To overcome these limitations, we have developed a fast and noninvasive three-stage method to characterize morphology of freely swimming human sperms in bright-field microscopy images using deep learning models. Specifically, we use an object detection model to identify sperm heads, a classification model to select in-focus images, and a segmentation model to extract geometry of sperm heads and vacuoles. The models achieve an F1-score of 0.951 in sperm head detection, a z-position estimation error within ±1.5 μm in in-focus image selection, and a Dice score of 0.948 in sperm head segmentation, respectively. Customized lightweight architectures are used for the models to achieve real-time analysis of 200 frames per second. Comprehensive morphological parameters are calculated from sperm head geometry extracted by image segmentation. Overall, our method provides a reliable and efficient tool to assist clinicians in selecting high-quality sperms for successful IVF. It also demonstrates the effectiveness of deep learning in real-time analysis of live bright-field microscopy images.
更多查看译文
关键词
bright-field microscopy,deep learning,human sperm,intracytoplasmic sperm injection,sperm morphology
AI 理解论文
溯源树
样例
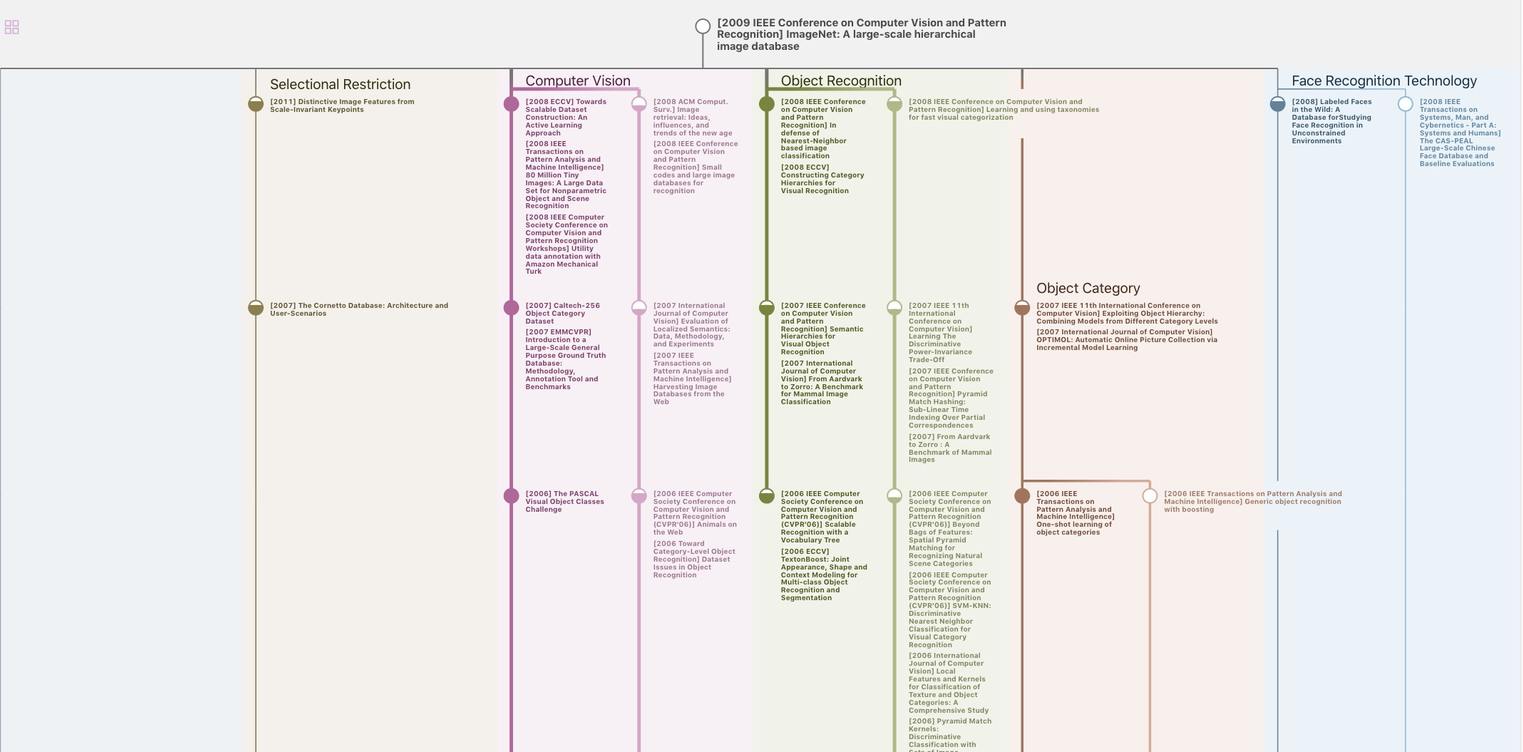
生成溯源树,研究论文发展脉络
Chat Paper
正在生成论文摘要