Personalized Meta-Learning for Domain Agnostic Learning from Demonstration
ACM/IEEE International Conference on Human-Robot Interaction(2022)
摘要
For robots to perform novel tasks in the real-world, they must be capable of learning from heterogeneous, non-expert human teachers across various domains. Yet, novice human teachers often provide suboptimal demonstrations, making it difficult for robots to successfully learn. Therefore, to effectively learn from humans, we must develop learning methods that can account for teacher suboptimality and can do so across various robotic platforms. To this end, we introduce Mutual Information Driven Meta-Learning from Demonstration (MIND MELD) [12, 13], a personalized meta-learning framework which meta-learns a mapping from suboptimal human feedback to feedback closer to optimal, conditioned on a learned personalized embedding. In a human subjects study, we demonstrate MIND MELD's ability to improve upon suboptimal demonstrations and learn meaningful, personalized embeddings. We then propose Domain Agnostic MIND MELD, which learns to transfer the personalized embedding learned in one domain to a novel domain, thereby allowing robots to learn from suboptimal humans across disparate platforms (e.g., self-driving car or in-home robot).
更多查看译文
关键词
meta-learning,personalized learning
AI 理解论文
溯源树
样例
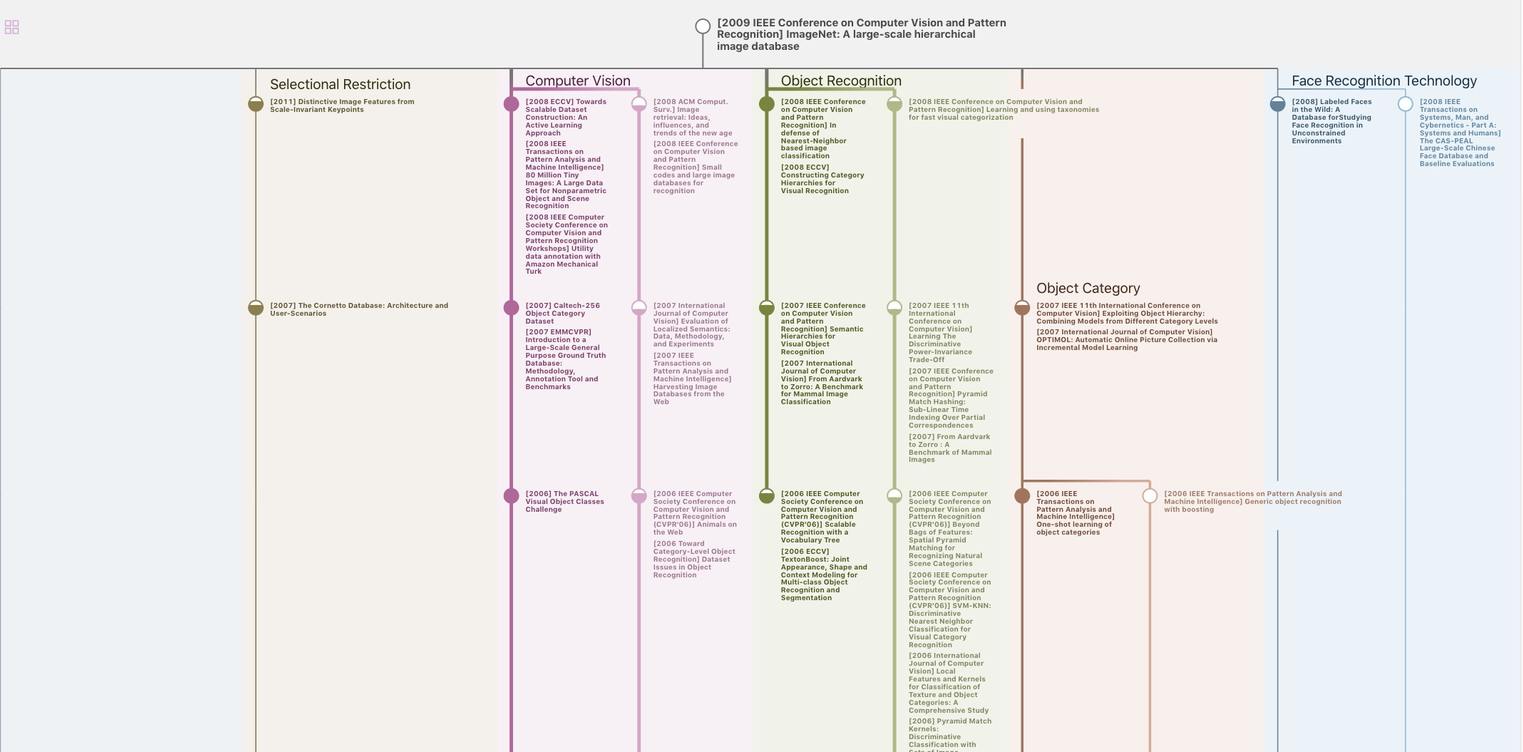
生成溯源树,研究论文发展脉络
Chat Paper
正在生成论文摘要