Multiple Instance Learning with Mixed Supervision in Gleason Grading
MEDICAL IMAGE COMPUTING AND COMPUTER ASSISTED INTERVENTION, MICCAI 2022, PT VIII(2022)
摘要
With the development of computational pathology, deep learning methods for Gleason grading through whole slide images (WSIs) have excellent prospects. Since the size of WSIs is extremely large, the image label usually contains only slide-level label or limited pixel-level labels. The current mainstream approach adopts multi-instance learning to predict Gleason grades. However, some methods only considering the slide-level label ignore the limited pixel-level labels containing rich local information. Furthermore, the method of additionally considering the pixel-level labels ignores the inaccuracy of pixel-level labels. To address these problems, we propose a mixed supervision Transformer based on the multiple instance learning framework. The model utilizes both slidelevel label and instance-level labels to achieve more accurate Gleason grading at the slide level. The impact of inaccurate instance-level labels is further reduced by introducing an efficient random masking strategy in the mixed supervision training process. We achieve the state-of-the-art performance on the SICAPv2 dataset, and the visual analysis shows the accurate prediction results of instance level. The source code is available at https: //github.com/bianhaol.23/Mixed_supervision.
更多查看译文
关键词
Gleason grading, Mixed supervision, Multiple instance learning
AI 理解论文
溯源树
样例
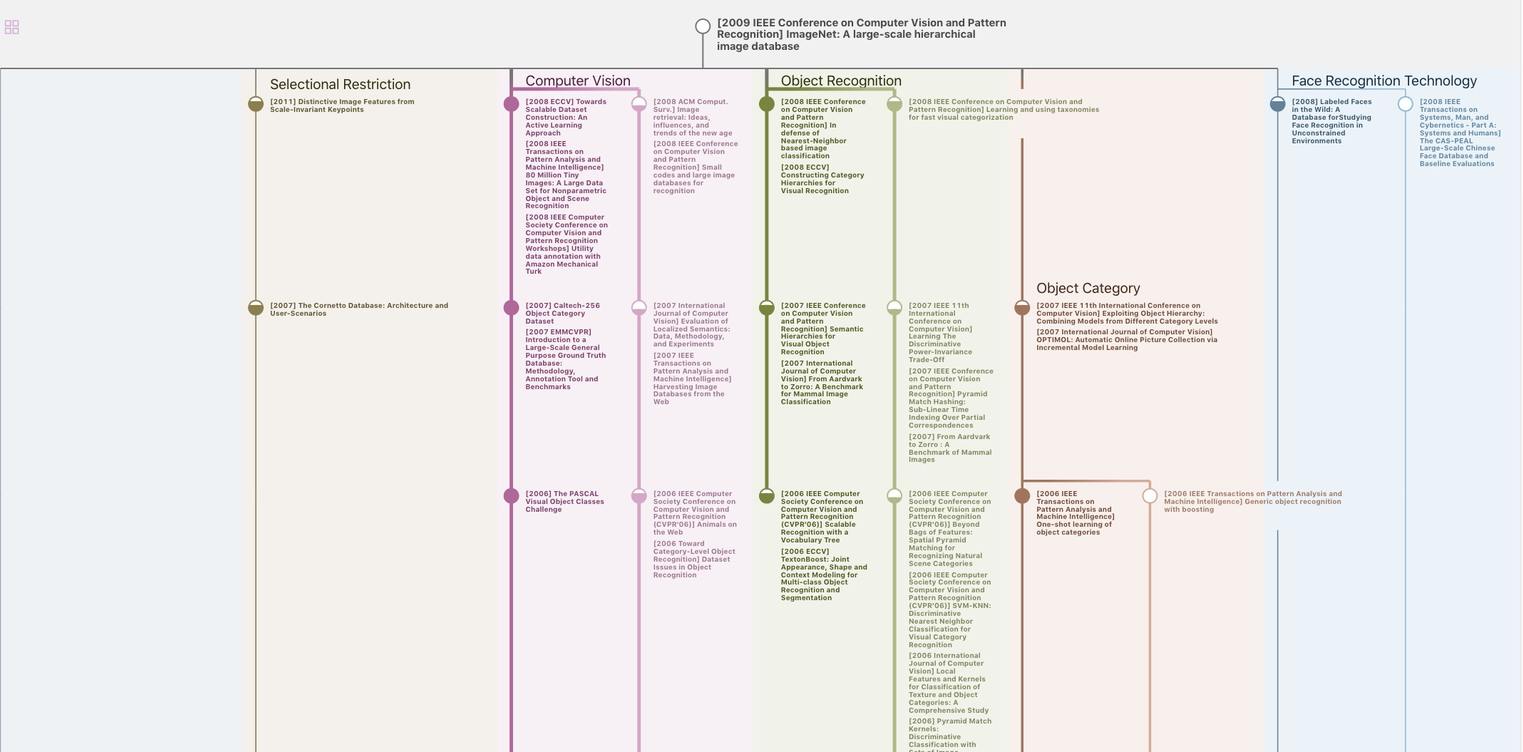
生成溯源树,研究论文发展脉络
Chat Paper
正在生成论文摘要