Prediction Errors for Penalized Regressions based on Generalized Approximate Message Passing
arxiv(2023)
摘要
We discuss the prediction accuracy of assumed statistical models in terms of prediction errors for the generalized linear model and penalized maximum likelihood methods. We derive the forms of estimators for the prediction errors, such as $C_p$ criterion, information criteria, and leave-one-out cross validation (LOOCV) error, using the generalized approximate message passing (GAMP) algorithm and replica method. These estimators coincide with each other when the number of model parameters is sufficiently small; however, there is a discrepancy between them in particular in the parameter region where the number of model parameters is larger than the data dimension. In this paper, we review the prediction errors and corresponding estimators, and discuss their differences. In the framework of GAMP, we show that the information criteria can be expressed by using the variance of the estimates. Further, we demonstrate how to approach LOOCV error from the information criteria by utilizing the expression provided by GAMP.
更多查看译文
关键词
prediction error,cross validation,information criteria,message passing
AI 理解论文
溯源树
样例
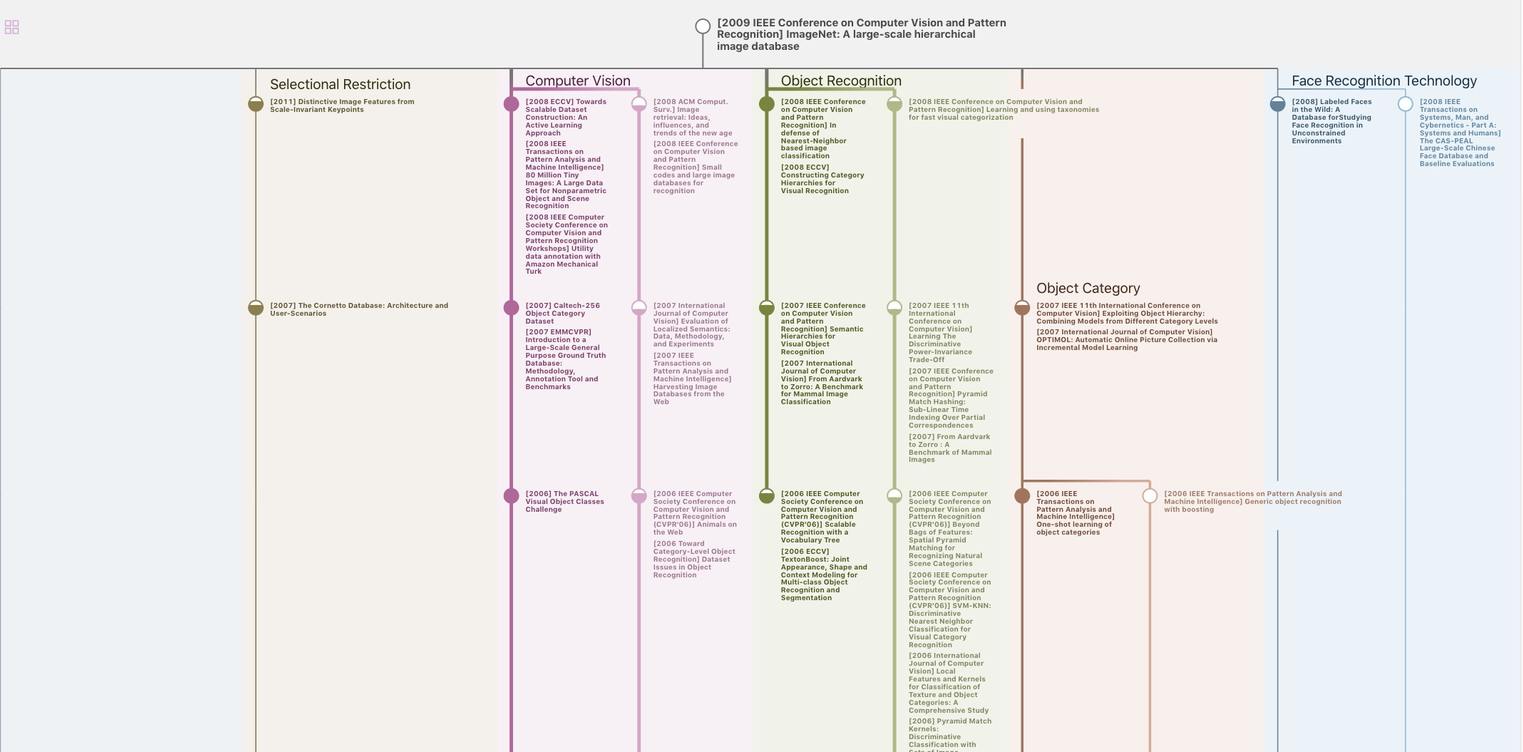
生成溯源树,研究论文发展脉络
Chat Paper
正在生成论文摘要