Automated Machine Learning for the Early Prediction of the Severity of Acute Pancreatitis in Hospitals
FRONTIERS IN CELLULAR AND INFECTION MICROBIOLOGY(2022)
摘要
BackgroundMachine learning (ML) algorithms are widely applied in building models of medicine due to their powerful studying and generalizing ability. This study aims to explore different ML models for early identification of severe acute pancreatitis (SAP) among patients hospitalized for acute pancreatitis. MethodsThis retrospective study enrolled patients with acute pancreatitis (AP) from multiple centers. Data from the First Affiliated Hospital and Changshu No. 1 Hospital of Soochow University were adopted for training and internal validation, and data from the Second Affiliated Hospital of Soochow University were adopted for external validation from January 2017 to December 2021. The diagnosis of AP and SAP was based on the 2012 revised Atlanta classification of acute pancreatitis. Models were built using traditional logistic regression (LR) and automated machine learning (AutoML) analysis with five types of algorithms. The performance of models was evaluated by the receiver operating characteristic (ROC) curve, the calibration curve, and the decision curve analysis (DCA) based on LR and feature importance, SHapley Additive exPlanation (SHAP) Plot, and Local Interpretable Model Agnostic Explanation (LIME) based on AutoML. ResultsA total of 1,012 patients were included in this study to develop the AutoML models in the training/validation dataset. An independent dataset of 212 patients was used to test the models. The model developed by the gradient boost machine (GBM) outperformed other models with an area under the ROC curve (AUC) of 0.937 in the validation set and an AUC of 0.945 in the test set. Furthermore, the GBM model achieved the highest sensitivity value of 0.583 among these AutoML models. The model developed by eXtreme Gradient Boosting (XGBoost) achieved the highest specificity value of 0.980 and the highest accuracy of 0.958 in the test set. ConclusionsThe AutoML model based on the GBM algorithm for early prediction of SAP showed evident clinical practicability.
更多查看译文
关键词
automated machine learning,logistic regression analysis,severe acute pancreatitis,predictive models,artificial intelligence
AI 理解论文
溯源树
样例
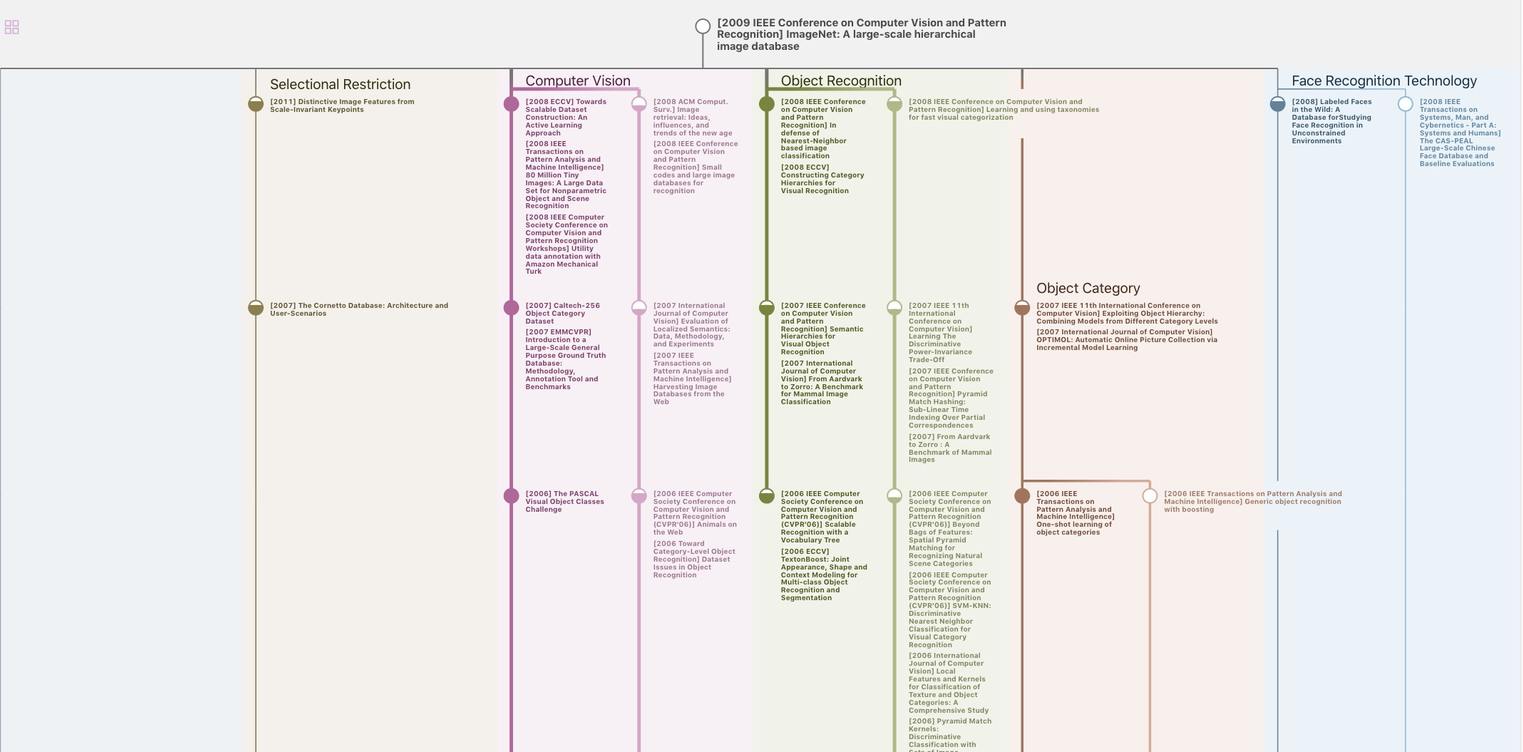
生成溯源树,研究论文发展脉络
Chat Paper
正在生成论文摘要