Optimal Estimation of Generic Dynamics by Path-Dependent Neural Jump ODEs
arxiv(2022)
摘要
This paper studies the problem of forecasting general stochastic processes using an extension of the Neural Jump ODE (NJ-ODE) framework. While NJ-ODE was the first framework to establish convergence guarantees for the prediction of irregularly observed time series, these results were limited to data stemming from It\^o-diffusions with complete observations, in particular Markov processes where all coordinates are observed simultaneously. In this work, we generalise these results to generic, possibly non-Markovian or discontinuous, stochastic processes with incomplete observations, by utilising the reconstruction properties of the signature transform. These theoretical results are supported by empirical studies, where it is shown that the path-dependent NJ-ODE outperforms the original NJ-ODE framework in the case of non-Markovian data. Moreover, we show that PD-NJ-ODE can be applied successfully to limit order book (LOB) data.
更多查看译文
关键词
generic dynamics,optimal estimation,odes,path-dependent
AI 理解论文
溯源树
样例
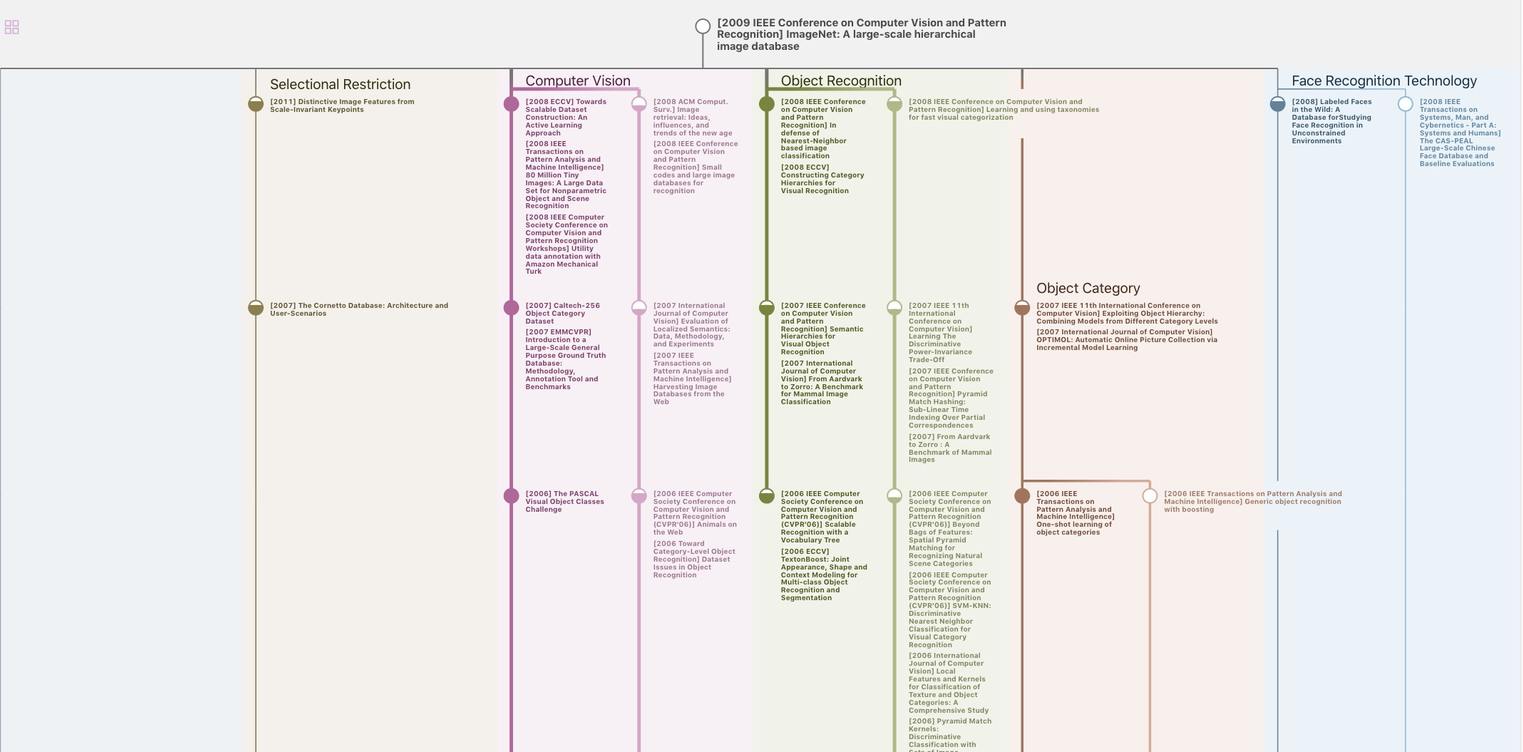
生成溯源树,研究论文发展脉络
Chat Paper
正在生成论文摘要