Cyclical Kernel Adaptive Metropolis
arxiv(2022)
摘要
We propose cKAM, cyclical Kernel Adaptive Metropolis, which incorporates a cyclical stepsize scheme to allow control for exploration and sampling. We show that on a crafted bimodal distribution, existing Adaptive Metropolis type algorithms would fail to converge to the true posterior distribution. We point out that this is because adaptive samplers estimates the local/global covariance structure using past history of the chain, which will lead to adaptive algorithms be trapped in a local mode. We demonstrate that cKAM encourages exploration of the posterior distribution and allows the sampler to escape from a local mode, while maintaining the high performance of adaptive methods.
更多查看译文
关键词
kernel
AI 理解论文
溯源树
样例
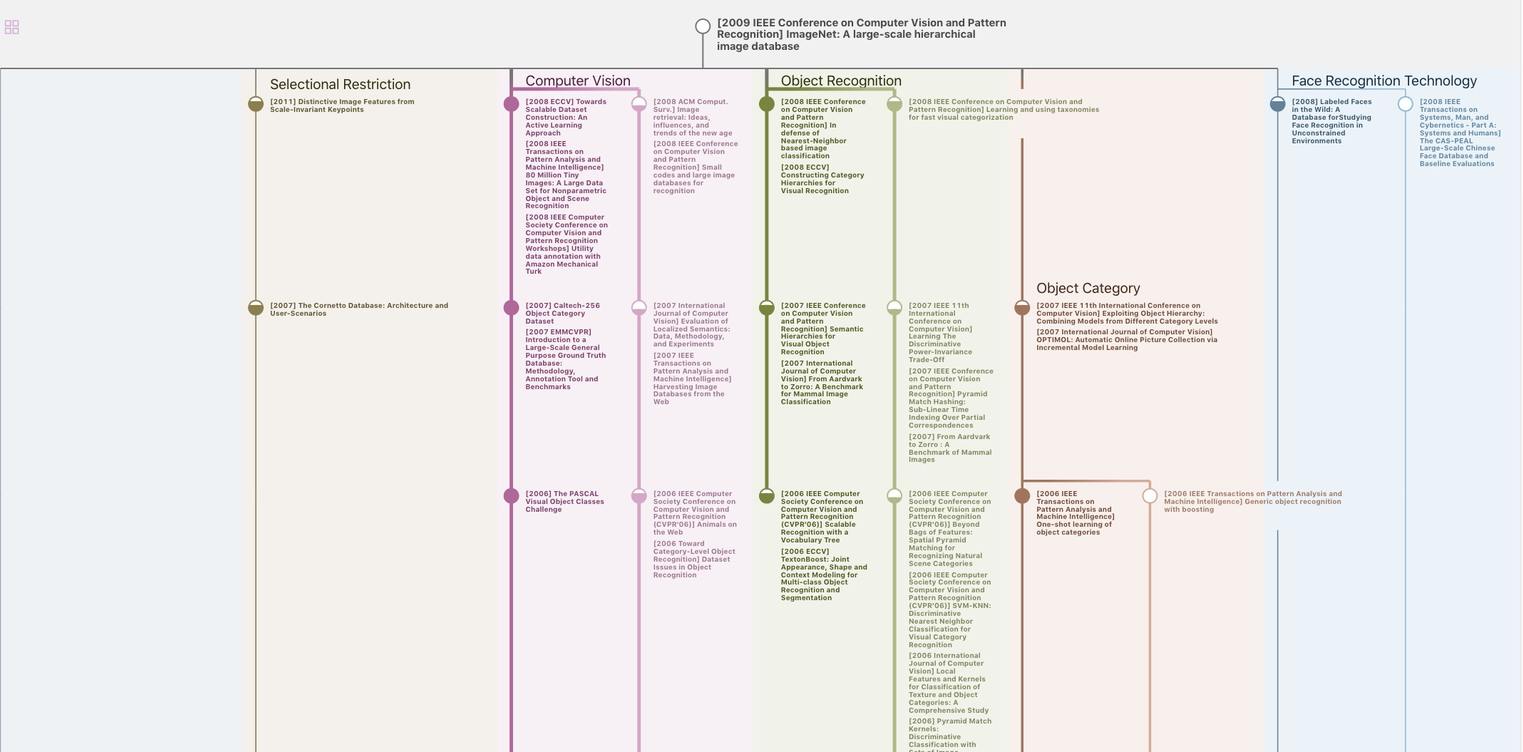
生成溯源树,研究论文发展脉络
Chat Paper
正在生成论文摘要