On the Prediction Network Architecture in RNN-T for ASR
Conference of the International Speech Communication Association (INTERSPEECH)(2022)
摘要
RNN-T models have gained popularity in the literature and in commercial systems because of their competitiveness and capability of operating in online streaming mode. In this work, we conduct an extensive study comparing several prediction network architectures for both monotonic and original RNN-T models. We compare 4 types of prediction networks based on a common state-of-the-art Conformer encoder and report results obtained on Librispeech and an internal medical conversation data set. Our study covers both offline batch-mode and online streaming scenarios. In contrast to some previous works, our results show that Transformer does not always outperform LSTM when used as prediction network along with Conformer encoder. Inspired by our scoreboard, we propose a new simple prediction network architecture, N-Concat, that outperforms the others in our on-line streaming benchmark. Transformer and n-gram reduced architectures perform very similarly yet with some important distinct behaviour in terms of previous context. Overall we obtained up to 4.1 % relative WER improvement compared to our LSTM baseline, while reducing prediction network parameters by nearly an order of magnitude (8.4 times).
更多查看译文
关键词
Conformer, RNN-T, prediction network, online, MHSA, Transformer
AI 理解论文
溯源树
样例
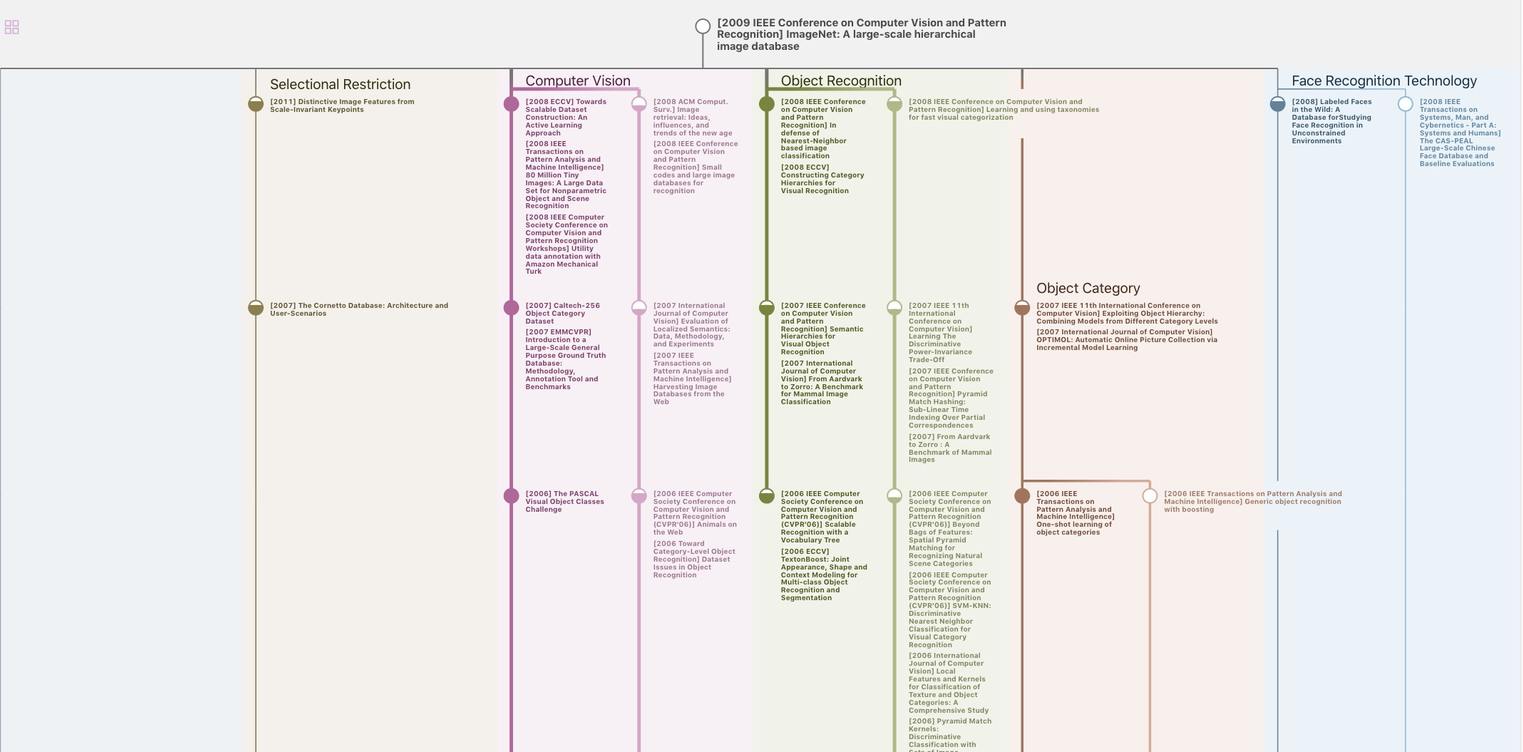
生成溯源树,研究论文发展脉络
Chat Paper
正在生成论文摘要