Regularization of NeRFs using differential geometry
arxiv(2022)
摘要
Neural radiance fields, or NeRF, represent a breakthrough in the field of novel view synthesis and 3D modeling of complex scenes from multi-view image collections. Numerous recent works have shown the importance of making NeRF models more robust, by means of regularization, in order to train with possibly inconsistent and/or very sparse data. In this work, we explore how differential geometry can provide elegant regularization tools for robustly training NeRF-like models, which are modified so as to represent continuous and infinitely differentiable functions. In particular, we present a generic framework for regularizing different types of NeRFs observations to improve the performance in challenging conditions. We also show how the same formalism can also be used to natively encourage the regularity of surfaces by means of Gaussian or mean curvatures.
更多查看译文
关键词
nerfs
AI 理解论文
溯源树
样例
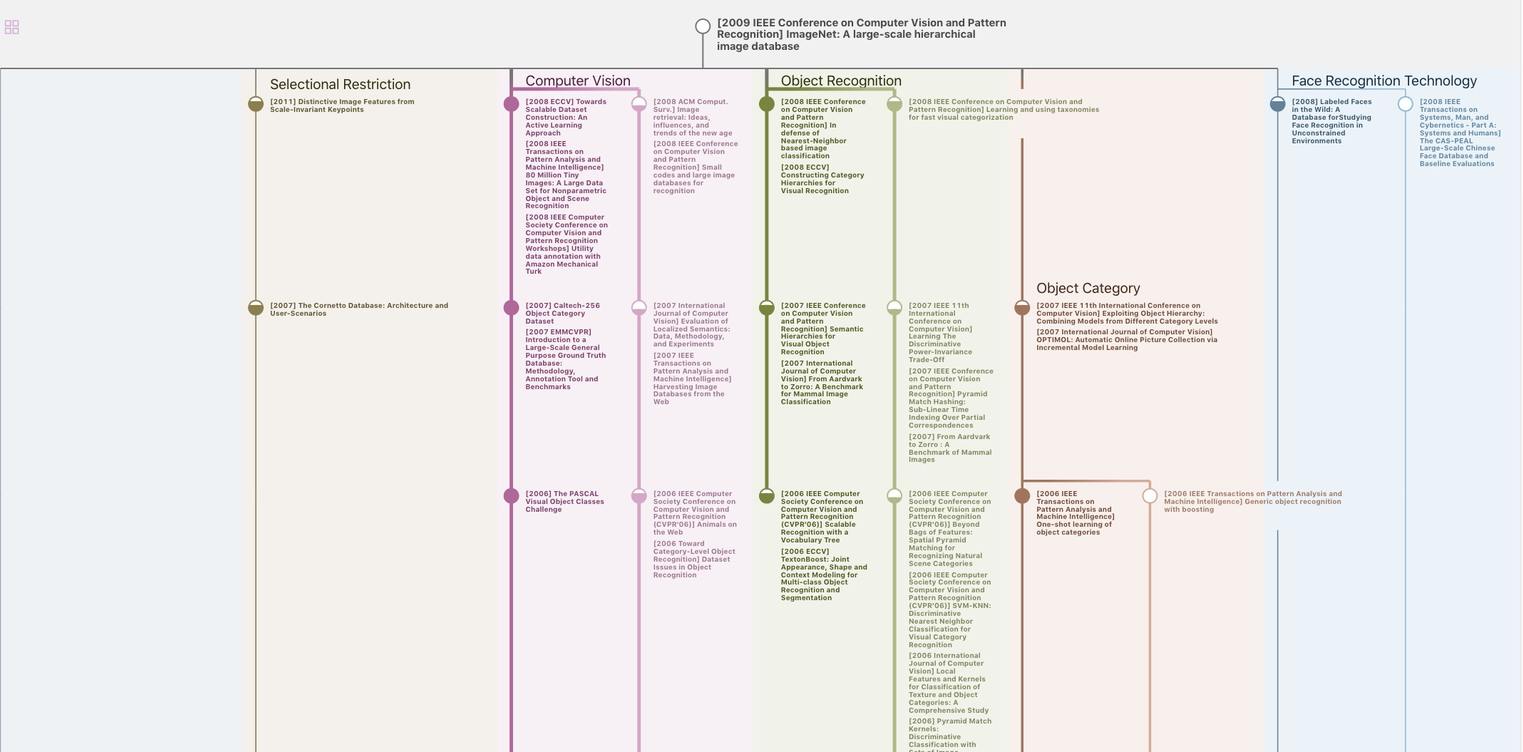
生成溯源树,研究论文发展脉络
Chat Paper
正在生成论文摘要