Prediction of Dilatory Behavior in eLearning: A Comparison of Multiple Machine Learning Models
IEEE TRANSACTIONS ON LEARNING TECHNOLOGIES(2023)
摘要
Procrastination, the irrational delay of tasks, is a common occurrence in online learning. Potential negative consequences include a higher risk of drop-outs, increased stress, and reduced mood. Due to the rise of learning management systems (LMS) and learning analytics (LA), indicators of such behavior can be detected, enabling predictions of future procrastination and other dilatory behavior. However, research focusing on such predictions is scarce. Moreover, studies involving different types of predictors and comparisons between the predictive performance of various methods are virtually nonexistent. In this article, we aim to fill these research gaps by analyzing the performance of multiple machine learning algorithms when predicting the delayed or timely submission of online assignments in a higher education setting with two categories of predictors: 1) subjective, questionnaire-based variables and 2) objective, log-data-based indicators extracted from a learning management system. The results show that models with objective predictors consistently outperform models with subjective predictors, and a combination of both variable types performs slightly better with an accuracy of 70%. For each of these three options, a different approach prevailed (gradient boosting machines for the subjective, Bayesian multilevel models for the objective, and Random Forest for the combined predictors). We conclude that careful attention should be paid to the selection of predictors and algorithms before implementing such models in learning management systems.
更多查看译文
关键词
Dilatory behavior,learning analytics (LA),machine learning (ML),predictive performance,procrastination,Delays,Predictive models,Behavioral sciences,Prediction algorithms,Task analysis,Data models,Classification algorithms
AI 理解论文
溯源树
样例
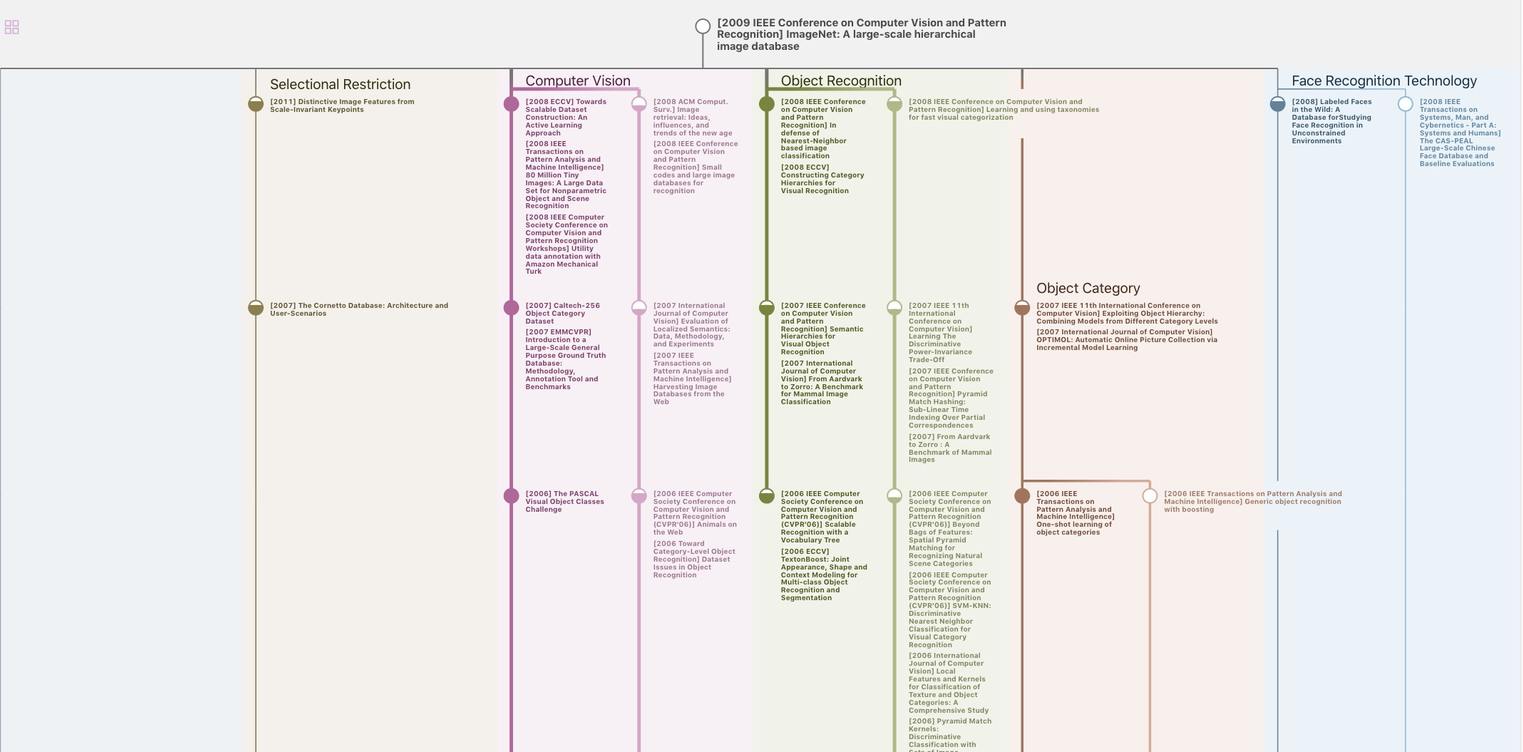
生成溯源树,研究论文发展脉络
Chat Paper
正在生成论文摘要