Implicit U-Net for Volumetric Medical Image Segmentation.
Annual Conference on Medical Image Understanding and Analysis (MIUA)(2022)
摘要
U-Net has been the go-to architecture for medical image segmentation tasks, however computational challenges arise when extending the U-Net architecture to 3D images. We propose the Implicit U-Net architecture that adapts the efficient Implicit Representation paradigm to supervised image segmentation tasks. By combining a convolutional feature extractor with an implicit localization network, our implicit U-Net has 40% less parameters than the equivalent U-Net. Moreover, we propose training and inference procedures to capitalize sparse predictions. When comparing to an equivalent fully convolutional U-Net, Implicit U-Net reduces by approximately 30% inference and training time as well as training memory footprint while achieving comparable results in our experiments with two different abdominal CT scan datasets.
更多查看译文
关键词
segmentation,u-net
AI 理解论文
溯源树
样例
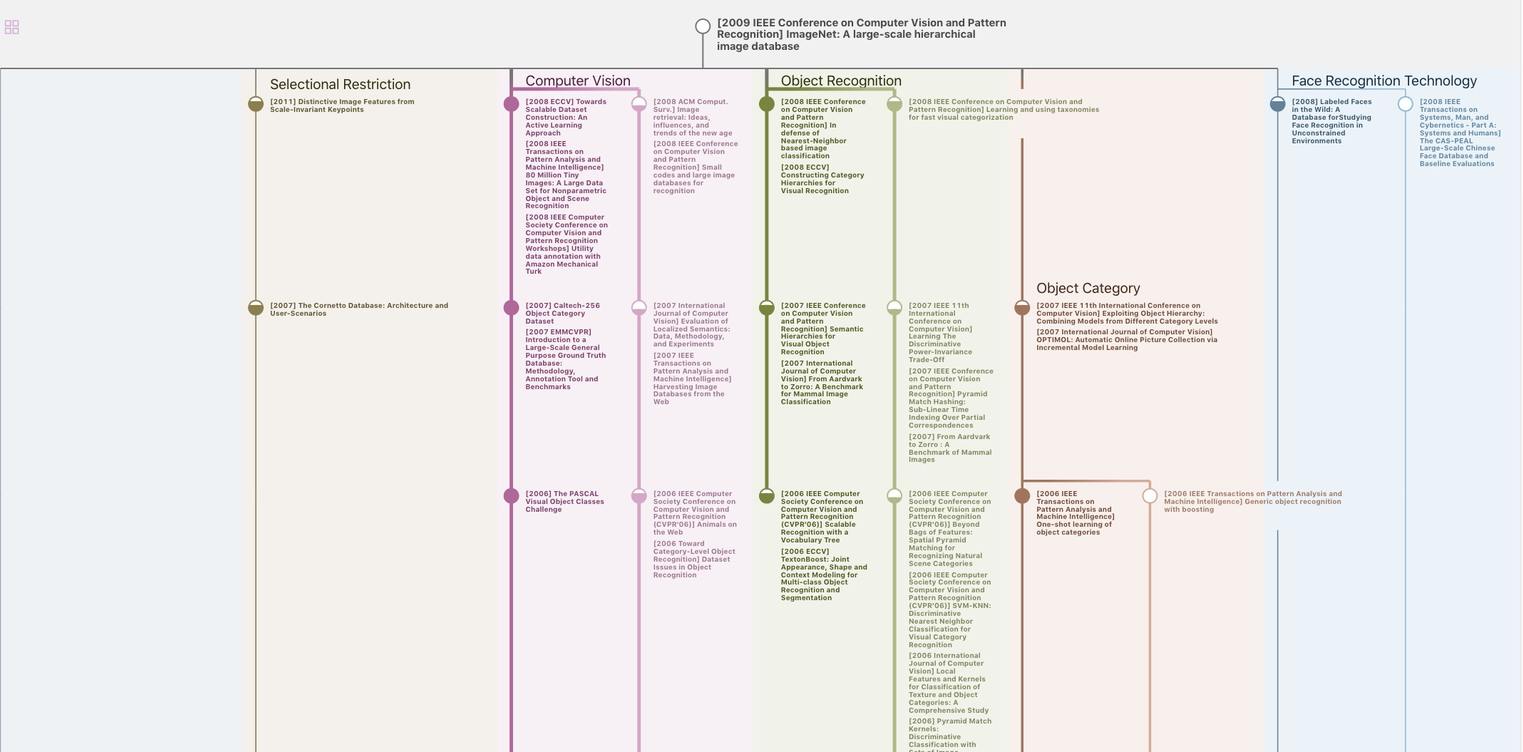
生成溯源树,研究论文发展脉络
Chat Paper
正在生成论文摘要