A Consistent and Efficient Evaluation Strategy for Attribution Methods
ICML 2022(2022)
摘要
With a variety of local feature attribution methods being proposed in recent years, follow-up work suggested several evaluation strategies. To assess the attribution quality across different attribution techniques, the most popular among these evaluation strategies in the image domain use pixel perturbations. However, recent advances discovered that different evaluation strategies produce conflicting rankings of attribution methods and can be prohibitively expensive to compute. In this work, we present an information-theoretic analysis of evaluation strategies based on pixel perturbations. Our findings reveal that the results are strongly affected by information leakage through the shape of the removed pixels as opposed to their actual values. Using our theoretical insights, we propose a novel evaluation framework termed Remove and Debias (ROAD) which offers two contributions: First, it mitigates the impact of the confounders, which entails higher consistency among evaluation strategies. Second, ROAD does not require the computationally expensive retraining step and saves up to 99% in computational costs compared to the state-of-the-art. We release our source code at https://github.com/tleemann/road_evaluation.
更多查看译文
关键词
attribution methods,efficient evaluation strategy
AI 理解论文
溯源树
样例
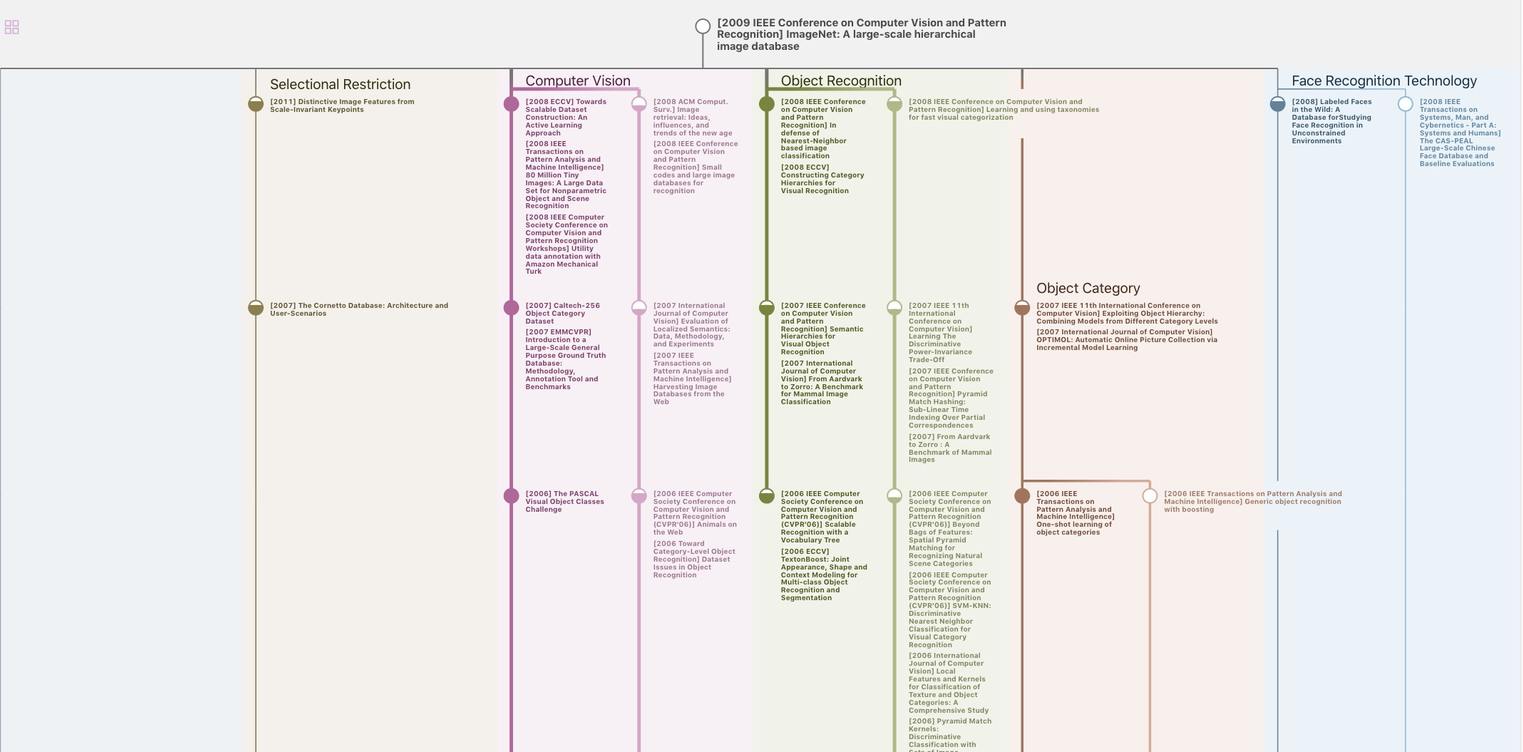
生成溯源树,研究论文发展脉络
Chat Paper
正在生成论文摘要