A Convergence Theory for SVGD in the Population Limit under Talagrand's Inequality T1.
International Conference on Machine Learning(2022)
摘要
Stein Variational Gradient Descent (SVGD) is an algorithm for sampling from a target density which is known up to a multiplicative constant. Although SVGD is a popular algorithm in practice, its theoretical study is limited to a few recent works. We study the convergence of SVGD in the population limit, (i.e., with an infinite number of particles) to sample from a non-logconcave target distribution satisfying Talagrand’s inequality T1. We first establish the convergence of the algorithm. Then, we establish a dimension-dependent complexity bound in terms of the Kernelized Stein Discrepancy (KSD). Unlike existing works, we do not assume that the KSD is bounded along the trajectory of the algorithm. Our approach relies on interpreting SVGD as a gradient descent over a space of probability measures.
更多查看译文
关键词
population limit,svgd,convergence theory,talagrand
AI 理解论文
溯源树
样例
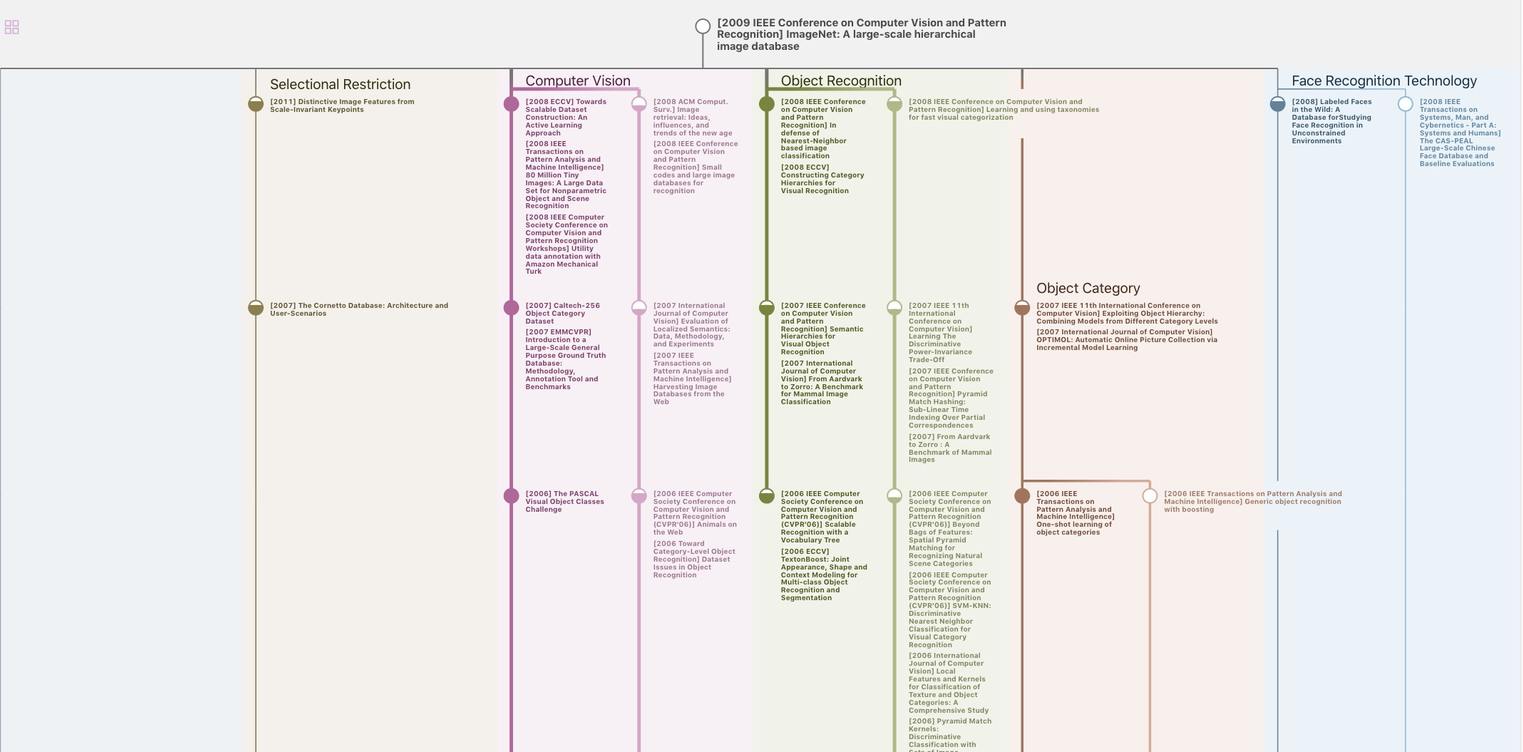
生成溯源树,研究论文发展脉络
Chat Paper
正在生成论文摘要