Nonparametric Involutive Markov Chain Monte Carlo.
International Conference on Machine Learning(2022)
摘要
A challenging problem in probabilistic programming is to develop inference algorithms that work for arbitrary programs in a universal probabilistic programming language (PPL). We present the nonparametric involutive Markov chain Monte Carlo (NP-iMCMC) algorithm as a method for constructing MCMC inference algorithms for nonparametric models expressible in universal PPLs. Building on the unifying involutive MCMC framework, and by providing a general procedure for driving state movement between dimensions, we show that NP-iMCMC can generalise numerous existing iMCMC algorithms to work on nonparametric models. We prove the correctness of the NP-iMCMC sampler. Our empirical study shows that the existing strengths of several iMCMC algorithms carry over to their nonparametric extensions. Applying our method to the recently proposed Nonparametric HMC, an instance of (Multiple Step) NP-iMCMC, we have constructed several nonparametric extensions (all of which new) that exhibit significant performance improvements.
更多查看译文
AI 理解论文
溯源树
样例
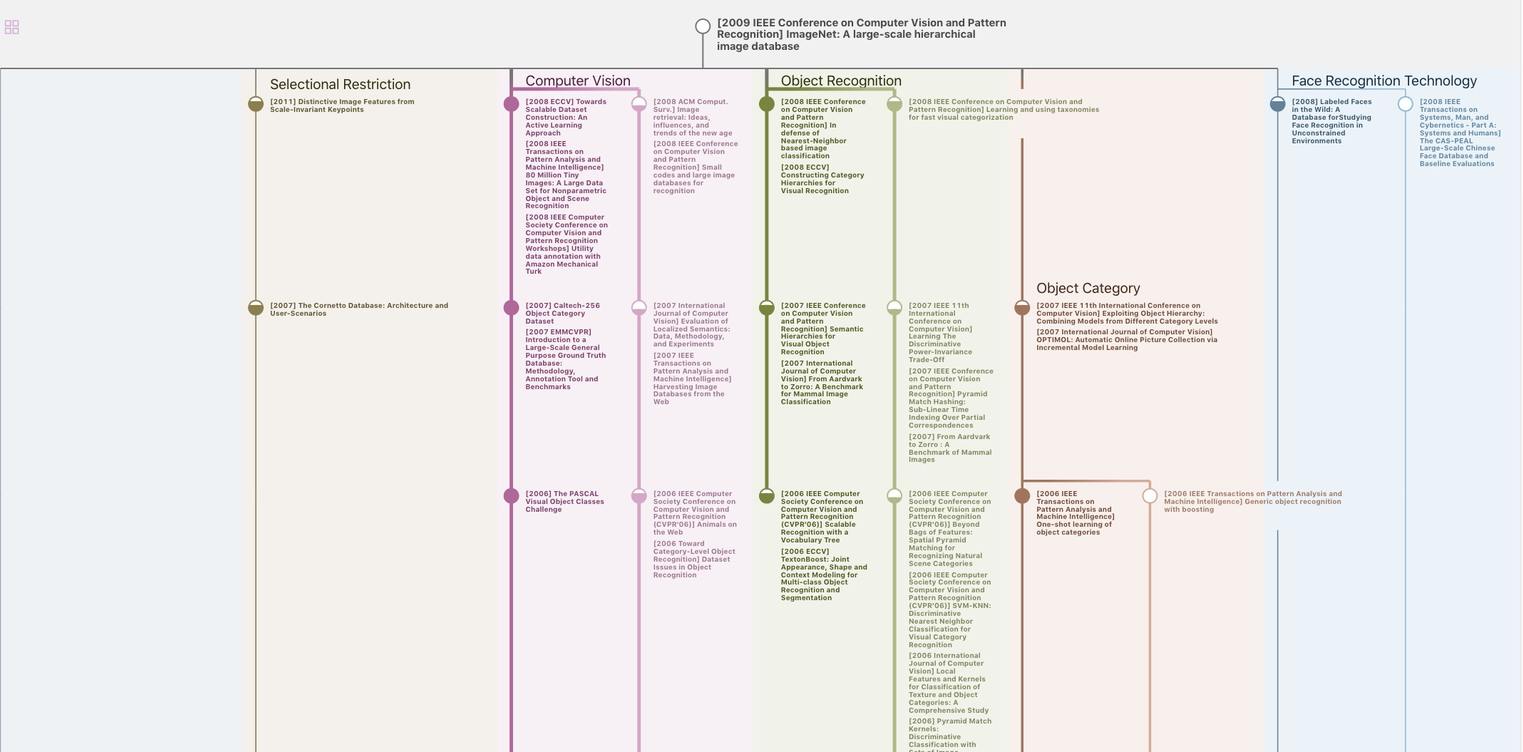
生成溯源树,研究论文发展脉络
Chat Paper
正在生成论文摘要