Learning to Memorize Feature Hallucination for One-Shot Image Generation
IEEE Conference on Computer Vision and Pattern Recognition(2022)
摘要
This paper studies the task of One-Shot image Generation (OSG), where generation network learned on base dataset should be generalizable to synthesize images of novel categories with only one available sample per novel category. Most existing methods for feature transfer in oneshot image generation only learn reusable features implicitly on pre-training tasks. Such methods would be likely to overfit pre-training tasks. In this paper, we propose a novel model to explicitly learn and memorize reusable features that can help hallucinate novel category images. To be specific, our algorithm learns to decompose image features into the Category-Related (CR) and Category-Independent(CI) features. Our model learning to memorize class-independent CI features which are further utilized by our feature hallucination component to generate target novel category images. We validate our model on several benchmarks. Extensive experiments demonstrate that our model effectively boosts the OSG performance and can generate compelling and diverse samples.
更多查看译文
关键词
Transfer/low-shot/long-tail learning, Image and video synthesis and generation
AI 理解论文
溯源树
样例
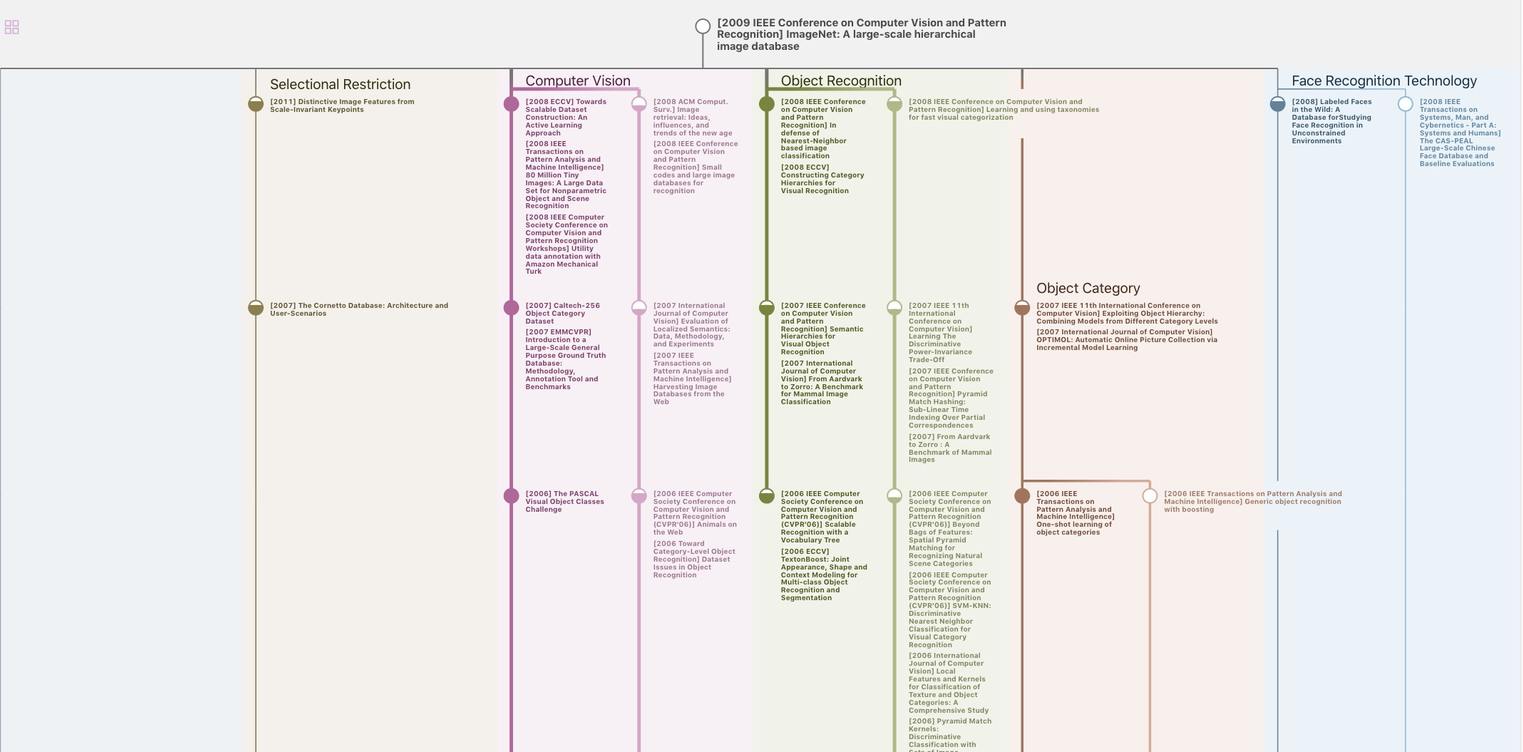
生成溯源树,研究论文发展脉络
Chat Paper
正在生成论文摘要