PPDL: Predicate Probability Distribution based Loss for Unbiased Scene Graph Generation
IEEE Conference on Computer Vision and Pattern Recognition(2022)
摘要
Scene Graph Generation (SGG) has attracted more and more attention from visual researchers in recent years, since Scene Graph (SG) is valuable in many downstream tasks due to its rich structural-semantic details. However, the ap-plication value of SG on downstream tasks is severely lim-ited by the predicate classification bias, which is caused by long-tailed data and presented as semantic bias of predicted relation predicates. Existing methods mainly reduce the prediction bias by better aggregating contexts and integrating external priori knowledge, but rarely take the semantic similarities between predicates into account. In this paper, we propose a Predicate Probability Distribution based Loss (PPDL) to train the biased SGG models and obtain unbi-ased Scene Graphs ultimately. Firstly, we propose a predi-cate probability distribution as the semantic representation of a particular predicate class. Afterwards, we re-balance the biased training loss according to the similarity between the predicted probability distribution and the estimated one, and eventually eliminate the long-tailed bias on predicate classification. Notably, the PPDL training method is model- agnostic, and extensive experiments and qualitative anal-yses on the Visual Genome dataset reveal significant per-formance improvements of our method on tail classes compared to the state-of-the-art methods.
更多查看译文
关键词
retrieval, Transfer/low-shot/long-tail learning, Vision + graphics, Visual reasoning,categorization,Scene analysis and understanding, Deep learning architectures and techniques, Machine learning, Optimization methods, Recognition: detection
AI 理解论文
溯源树
样例
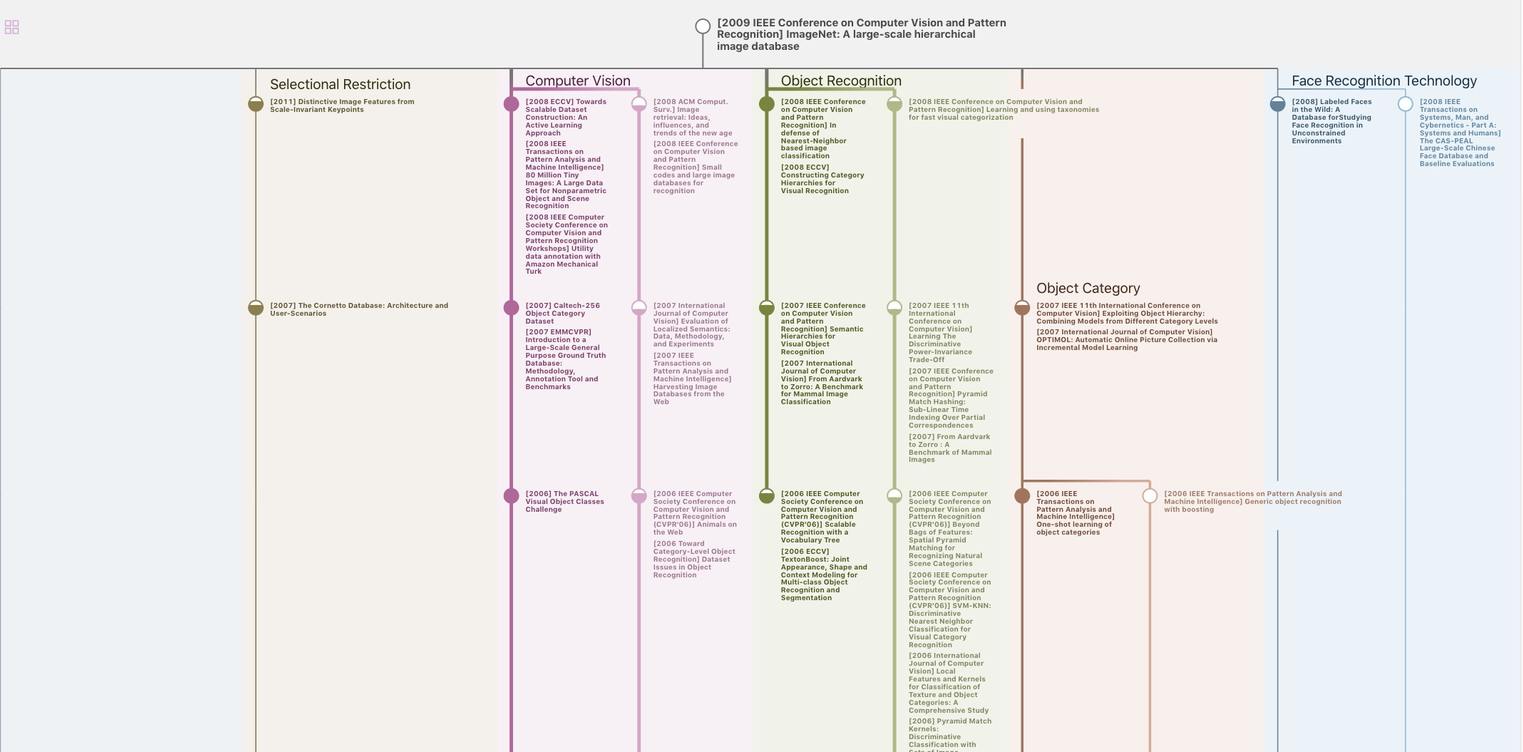
生成溯源树,研究论文发展脉络
Chat Paper
正在生成论文摘要