Task Discrepancy Maximization for Fine-grained Few-Shot Classification
IEEE Conference on Computer Vision and Pattern Recognition(2022)
摘要
Recognizing discriminative details such as eyes and beaks is important for distinguishing fine-grained classes since they have similar overall appearances. In this regard, we introduce Task Discrepancy Maximization (TDM), a simple module for fine-grained few-shot classification. Our objective is to localize the class-wise discriminative regions by highlighting channels encoding distinct information of the class. Specifically, TDM learns task-specific channel weights based on two novel components: Support Attention Module (SAM) and Query Attention Module (QAM). SAM produces a support weight to represent channel-wise discriminative power for each class. Still, since the SAM is basically only based on the labeled support sets, it can be vulnerable to bias toward such support set. Therefore, we propose QAM which complements SAM by yielding a query weight that grants more weight to object-relevant channels for a given query image. By combining these two weights, a class-wise task-specific channel weight is defined. The weights are then applied to produce task-adaptive feature maps more focusing on the discriminative details. Our experiments validate the effectiveness of TDM and its complementary benefits with prior methods in fine- grained few-shot classification.
更多查看译文
关键词
retrieval, Self-& semi-& meta- & unsupervised learning,categorization,Recognition: detection
AI 理解论文
溯源树
样例
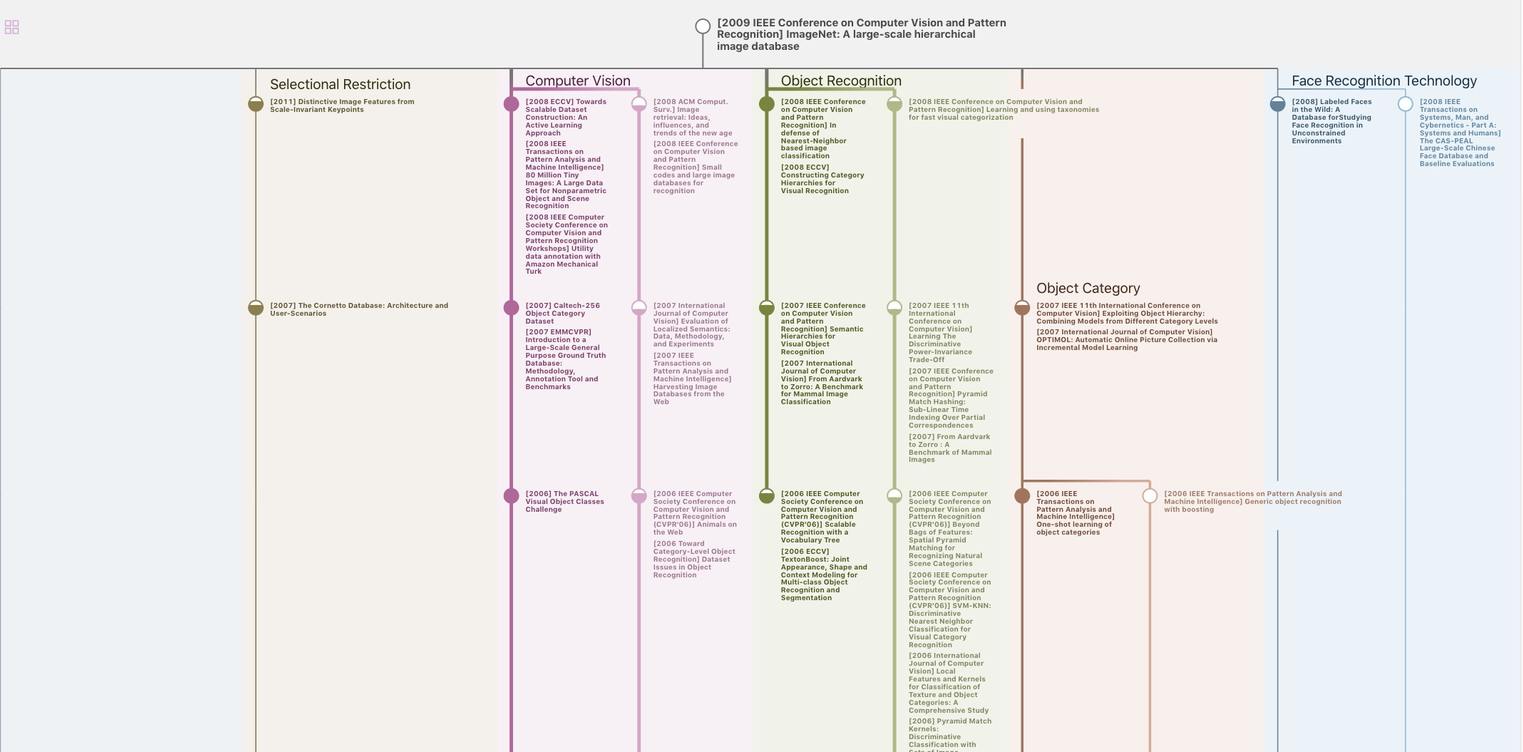
生成溯源树,研究论文发展脉络
Chat Paper
正在生成论文摘要