InfoGCN: Representation Learning for Human Skeleton-based Action Recognition
IEEE Conference on Computer Vision and Pattern Recognition(2022)
摘要
Human skeleton-based action recognition offers a valuable means to understand the intricacies of human behavior because it can handle the complex relationships between physical constraints and intention. Although several studies have focused on encoding a skeleton, less attention has been paid to embed this information into the latent representations of human action. InfoGCN proposes a learning framework for action recognition combining a novel learning objective and an encoding method. First, we design an information bottleneck-based learning objective to guide the model to learn informative but compact latent representations. To provide discriminative information for classifying action, we introduce attention-based graph convolution that captures the context-dependent intrinsic topology of human action. In addition, we present a multi-modal representation of the skeleton using the relative position of joints, designed to provide complementary spatial information for joints. InfoGcn 1 1 Code is available at github.com/stnoahl/infogcn surpasses the known state-of-the-art on multiple skeleton-based action recognition benchmarks with the accuracy of 93.0% on NTU RGB+D 60 cross-subject split, 89.8% on NTU RGB+D 120 cross-subject split, and 97.0% on NW-UCLA.
更多查看译文
关键词
Action and event recognition, Behavior analysis, Representation learning
AI 理解论文
溯源树
样例
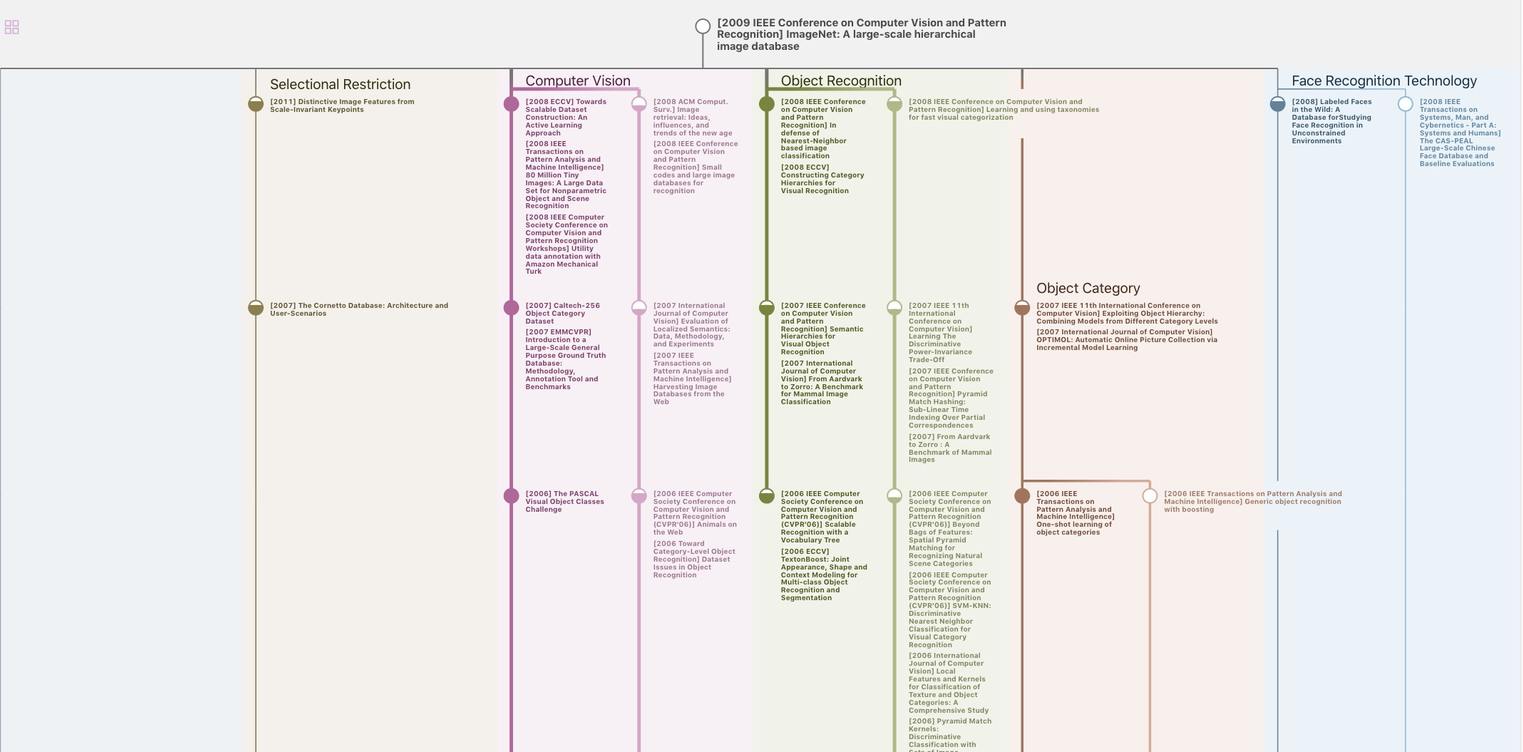
生成溯源树,研究论文发展脉络
Chat Paper
正在生成论文摘要