DLFormer: Discrete Latent Transformer for Video Inpainting
IEEE Conference on Computer Vision and Pattern Recognition(2022)
摘要
Video inpainting remains a challenging problem to fill with plausible and coherent content in unknown areas in video frames despite the prevalence of data-driven methods. Although various transformer-based architectures yield promising result for this task, they still suffer from hallucinating blurry contents and long-term spatial-temporal inconsistency. While noticing the capability of discrete representation for complex reasoning and predictive learning, we propose a novel Discrete Latent Transformer (DLFormer) to reformulate video inpainting tasks into the discrete latent space rather the previous continuous feature space. Specifically, we first learn a unique compact discrete codebook and the corresponding autoencoder to represent the target video. Built upon these representative discrete codes obtained from the entire target video, the subsequent discrete latent transformer is capable to infer proper codes for unknown areas under a self-attention mechanism, and thus produces fine-grained content with long-term spatial-temporal consistency. Moreover, we further explicitly enforce the short-term consistency to relieve temporal visual jitters via a temporal aggregation block among adjacent frames. We conduct comprehensive quantitative and qualitative evaluations to demonstrate that our method significantly outperforms other state-of-the-art approaches in reconstructing visually-plausible and spatial-temporal coherent content with fine-grained details. Code is available at https://github.com/JingjingRenabc/dlformer.
更多查看译文
关键词
Image and video synthesis and generation, Vision applications and systems
AI 理解论文
溯源树
样例
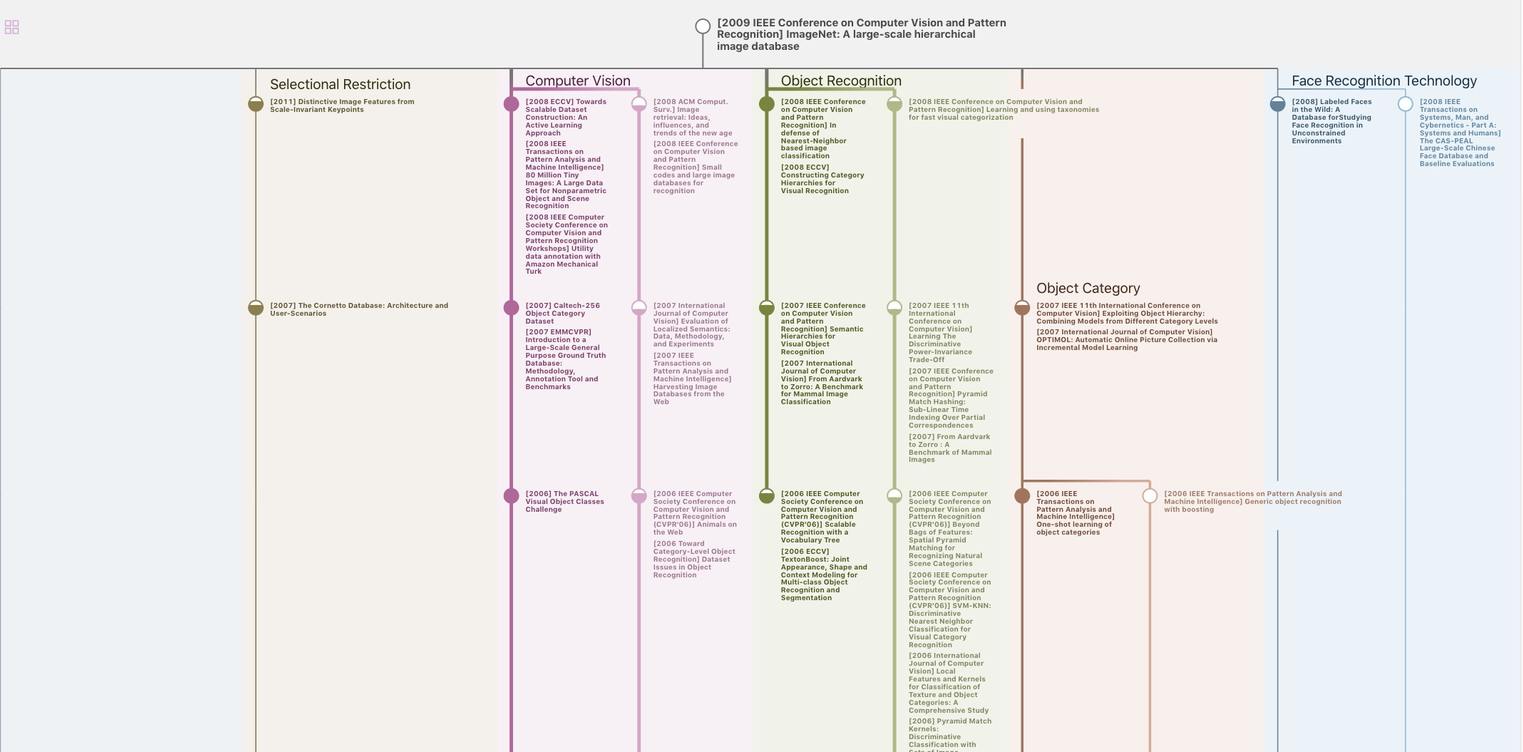
生成溯源树,研究论文发展脉络
Chat Paper
正在生成论文摘要