Cross-Domain Adaptive Teacher for Object Detection
IEEE Conference on Computer Vision and Pattern Recognition(2022)
摘要
We address the task of domain adaptation in object detection, where there is an obvious domain gap between a domain with annotations (source) and a domain of interest without annotations (target). As a popular semi-supervised learning method, the teacher-student framework (a student model is supervised by the pseudo labels from a teacher model) has also yielded a large accuracy gain in cross-domain object detection. However, it suffers from the domain shift and generates many low-quality pseudo labels (e.g., false positives), which leads to sub-optimal performance. To mitigate this problem, we propose a teacher-student framework named Adaptive Teacher (AT) which leverages domain adversarial learning and weak-strong data augmentation to address the domain gap. Specifically, we employ feature-level adversarial training in the student model, allowing features derived from the source and target domains to share similar distributions. This process ensures the student model produces domain-invariant features. Furthermore, we apply weak-strong augmentation and mutual learning between the teacher model (taking data from the target domain) and the student model (taking data from both domains). This enables the teacher model to learn the knowledge from the student model without being biased to the source domain. We show that AT demonstrates superiority over existing approaches and even Oracle (fully-supervised) models by a large margin. For example, we achieve 50.9% (49.3%) mAP on Foggy Cityscape (Cli-part1K), which is 9.2% (5.2%) and 8.2% (11.0%) higher than previous state-of-the-art and Oracle, respectively.
更多查看译文
关键词
Recognition: detection,categorization,retrieval, Representation learning, Self-& semi-& meta- & unsupervised learning
AI 理解论文
溯源树
样例
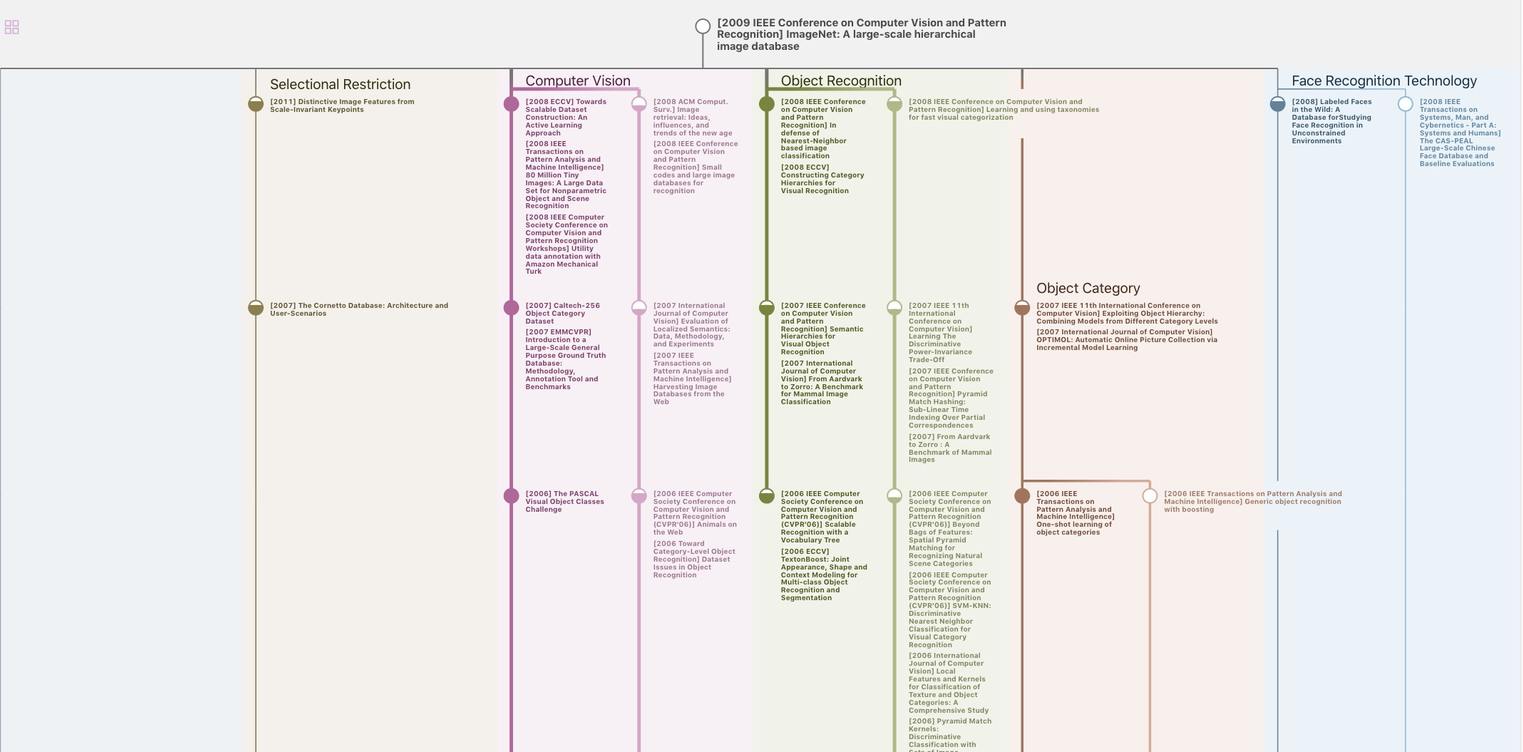
生成溯源树,研究论文发展脉络
Chat Paper
正在生成论文摘要