Ν-Flows: Conditional Neutrino Regression.
SCIPOST PHYSICS(2023)
摘要
We present & nu;-Flows, a novel method for restricting the likelihood space of neutrino kinematics in high-energy collider experiments using conditional normalising flows and deep invertible neural networks. This method allows the recovery of the full neutrino momentum which is usually left as a free parameter and permits one to sample neutrino values under a learned conditional likelihood given event observations. We demonstrate the success of & nu;-Flows in a case study by applying it to simulated semileptonic t t over bar events and show that it can lead to more accurate momentum reconstruction, particularly of the longitudinal coordinate. We also show that this has direct benefits in a downstream task of jet association, leading to an improvement of up to a factor of 1.41 compared to conventional methods.
更多查看译文
AI 理解论文
溯源树
样例
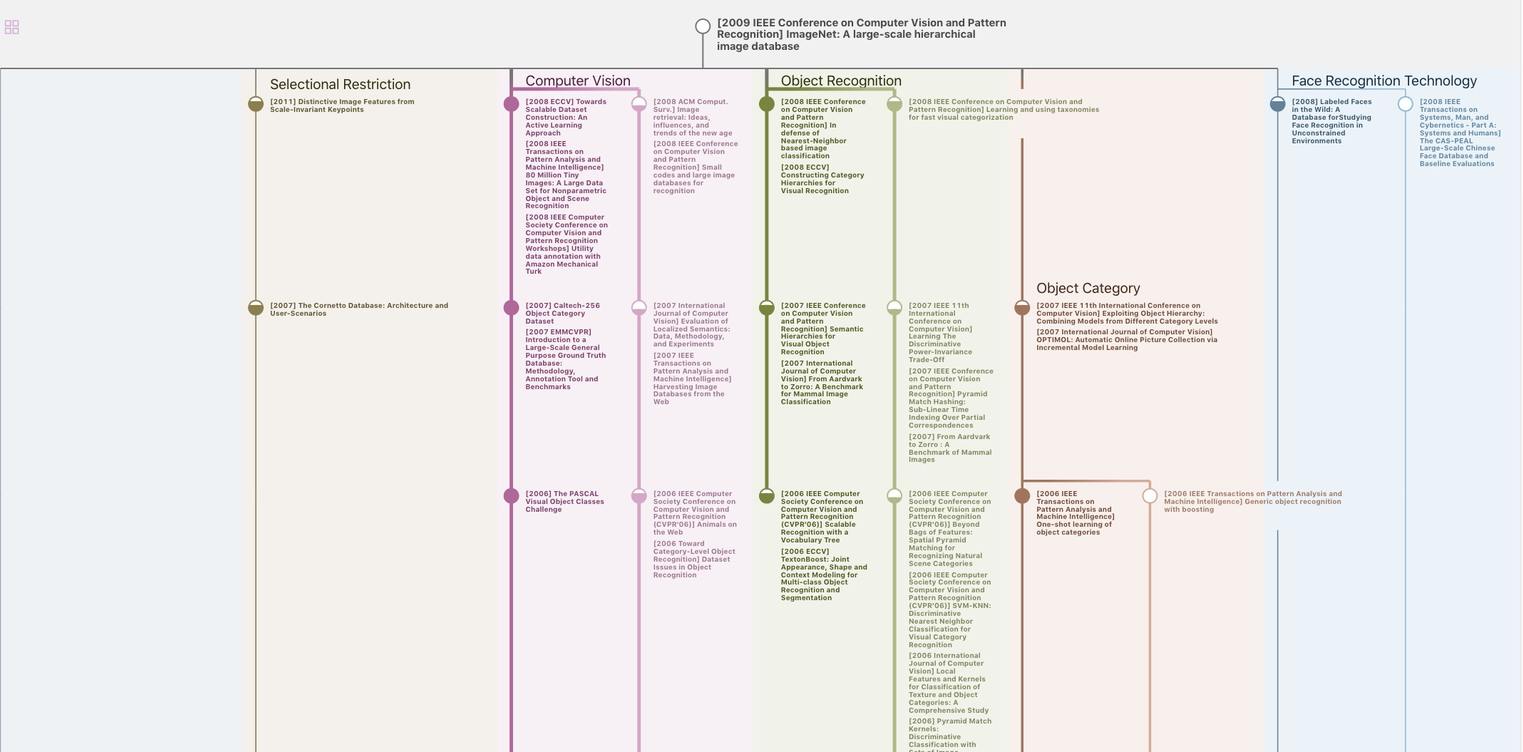
生成溯源树,研究论文发展脉络
Chat Paper
正在生成论文摘要