Turning to a Teacher for Timestamp Supervised Temporal Action Segmentation.
2022 IEEE International Conference on Multimedia and Expo (ICME)(2022)
摘要
Temporal action segmentation in videos has drawn much attention recently. Timestamp supervision is a cost-effective way for this task. To obtain more information to optimize the model, the existing method generated pseudo frame-wise labels iteratively based on the output of a segmentation model and the timestamp annotations. However, this practice may introduce noise and oscillation during the training, and lead to performance degeneration. To address this problem, we propose a new framework for timestamp supervised temporal action segmentation by introducing a teacher model parallel to the segmentation model to help stabilize the process of model optimization. The teacher model can be seen as an ensemble of the segmentation model, which helps to suppress the noise and to improve the stability of pseudo labels. We further introduce a segmentally smoothing loss, which is more focused and cohesive, to enforce the smooth transition of the predicted probabilities within action instances. The experiments on three datasets show that our method outperforms the state-of-the-art method and performs comparably against the fully-supervised methods at a much lower annotation cost.
更多查看译文
关键词
Timestamp Supervision,Temporal Action Segmentation,Mean Teacher
AI 理解论文
溯源树
样例
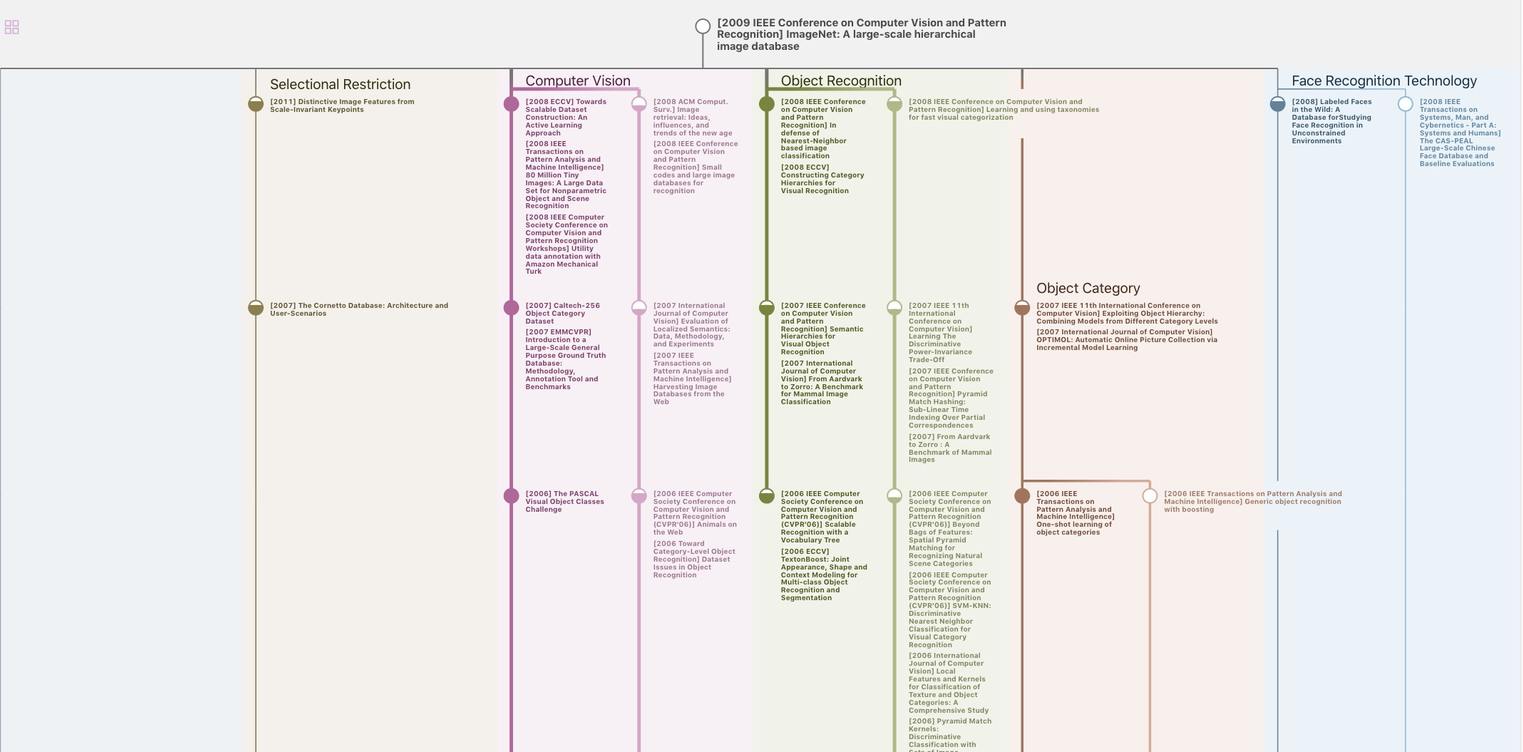
生成溯源树,研究论文发展脉络
Chat Paper
正在生成论文摘要