Digital-twin-enhanced metal tube bending forming real-time prediction method based on multi-source-input MTL
Structural and Multidisciplinary Optimization(2022)
摘要
As one of the most widely used metal tube bending methods, the rotary draw bending process enables reliable and high-precision metal tube bending forming (MTBF). The forming accuracy is seriously affected by the springback and other potential forming defects, of which the mechanism analysis is difficult to deal with. At the same time, the existing methods are mainly conducted in offline space, ignoring the real-time information in the physical world, which is unreliable and inefficient. To address this issue, a digital-twin-enhanced (DT-enhanced) metal tube bending forming real-time prediction method based on multi-source-input multi-task learning (MTL) is proposed. The new method can achieve comprehensive MTBF real-time prediction. By sharing the common feature of the multi-close domain and adopting group regularization strategy on feature sharing and accepting layers, the accuracy and efficiency of the multi-source-input MTL can be guaranteed. Enhanced by DT, the physical real-time deformation data are aligned in the image dimension by an improved Grammy Angle Field (GAF) conversion, realizing the reflection of the actual processing. Different from the traditional offline prediction methods, the new method integrates the virtual and physical data to achieve a more efficient and accurate real-time prediction result, and the DT mapping connection between virtual and physical systems can be achieved. To exclude the effects of equipment errors, the effectiveness of the proposed method is verified on the physical experiment-verified FE simulation scenarios. At the same time, the common pre-training networks are compared with the proposed method. The results show that the proposed DT-enhanced prediction method is more accurate and efficient.
更多查看译文
关键词
Metal tube bending forming (MTBF), Digital-twin (DT), Multi-source-input MTL, Group regularization strategy, GAF conversion
AI 理解论文
溯源树
样例
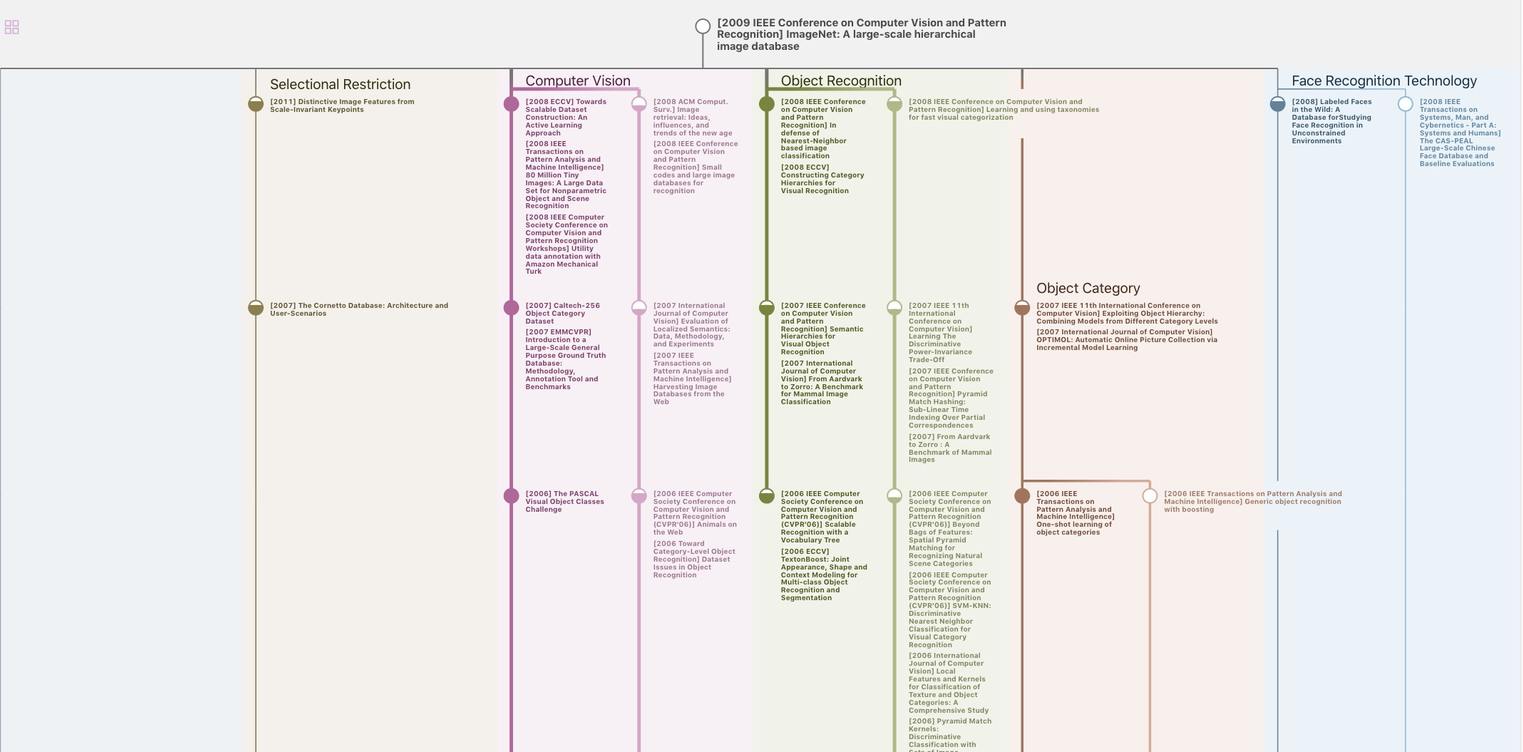
生成溯源树,研究论文发展脉络
Chat Paper
正在生成论文摘要