Minimax Optimal Deep Neural Network Classifiers Under Smooth Decision Boundary
arxiv(2022)
摘要
Deep learning has gained huge empirical successes in large-scale classification problems. In contrast, there is a lack of statistical understanding about deep learning methods, particularly in the minimax optimality perspective. For instance, in the classical smooth decision boundary setting, existing deep neural network (DNN) approaches are rate-suboptimal, and it remains elusive how to construct minimax optimal DNN classifiers. Moreover, it is interesting to explore whether DNN classifiers can circumvent the curse of dimensionality in handling high-dimensional data. The contributions of this paper are two-fold. First, based on a localized margin framework, we discover the source of suboptimality of existing DNN approaches. Motivated by this, we propose a new deep learning classifier using a divide-and-conquer technique: DNN classifiers are constructed on each local region and then aggregated to a global one. We further propose a localized version of the classical Tsybakov's noise condition, under which statistical optimality of our new classifier is established. Second, we show that DNN classifiers can adapt to low-dimensional data structures and circumvent the curse of dimensionality in the sense that the minimax rate only depends on the effective dimension, potentially much smaller than the actual data dimension. Numerical experiments are conducted on simulated data to corroborate our theoretical results.
更多查看译文
关键词
smooth decision
AI 理解论文
溯源树
样例
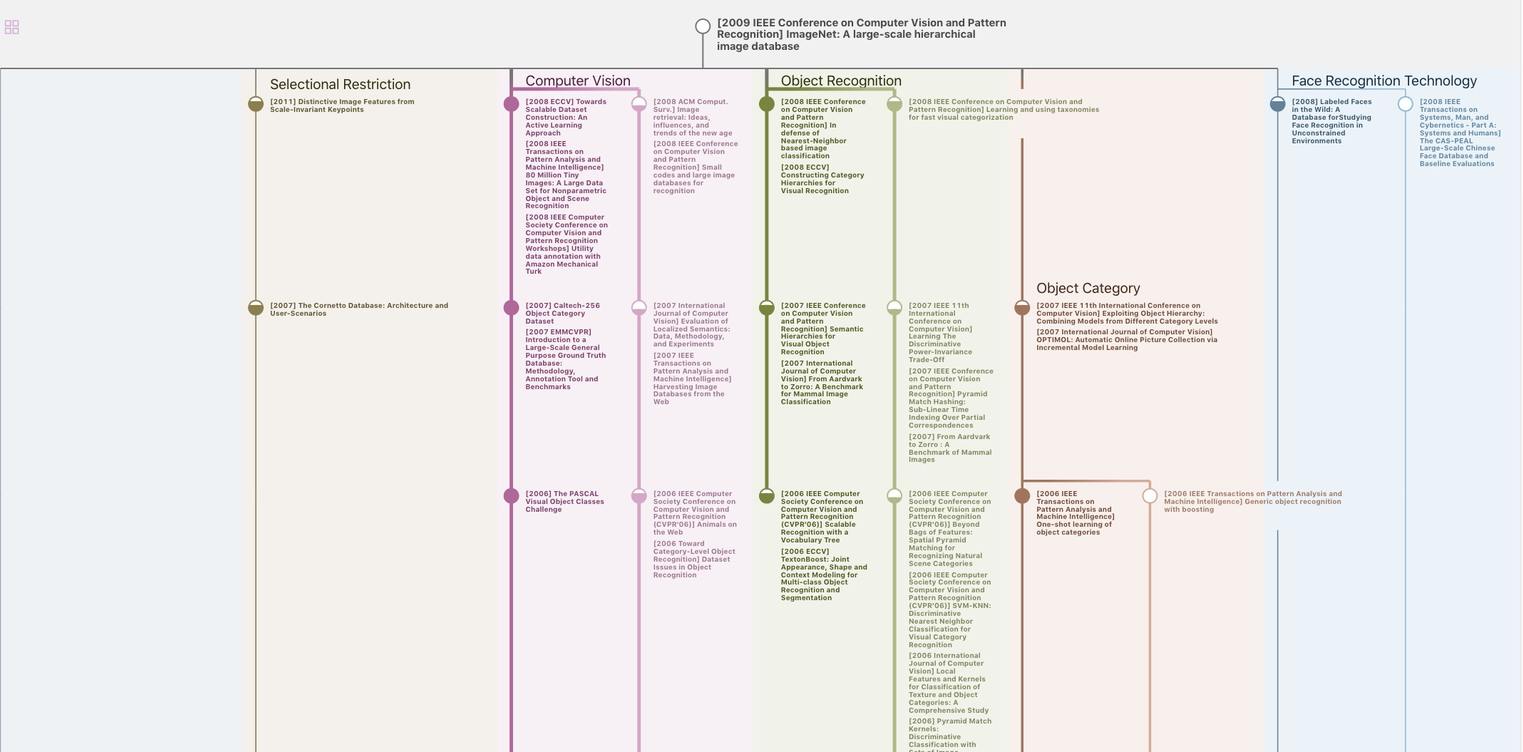
生成溯源树,研究论文发展脉络
Chat Paper
正在生成论文摘要