A New Sparse-Learning Model for Maximum Gap Reduction of Composite Fuselage Assembly
TECHNOMETRICS(2022)
摘要
Natural dimensional variabilities of incoming fuselages affect the assembly speed and quality of fuselage joins in composite fuselage assembly processes. Shape control is critical to ensure the quality of composite fuselage assembly. In current practice, the structures are adjusted to the design shape in terms of the l(2) loss for further assembly without considering the existing dimensional gap between two structures. Such practice has two limitations: (a) controlling each fuselage to the design shape may not be the optimal shape control strategy in terms of a pair of incoming fuselages with different incoming dimensions; (b) the maximum gap is the key concern during the fuselage assembly process, so the l(infinity) loss of gap after control ought to be considered. This article proposes an optimal shape control methodology via the l(infinity) loss for the composite fuselage assembly process by considering the existing dimensional gap between the incoming pair of fuselages. On the other hand, due to the limitation on the number of available actuators in practice, we face an important problem of finding the best locations for the actuators among many potential locations, which makes the problem a sparse estimation problem. We are the first to solve the optimal shape control in the fuselage assembly process using the l(infinity) model under the framework of sparse estimation, where we use the l(1) penalty to control the sparsity of the resulting estimator. From the statistical point of view, this can be formulated as the l(infinity) loss based linear regression, and under some standard assumptions, such as the restricted eigenvalue (RE) conditions, and the light-tailed noise, the nonasymptotic estimation error of the l(1) regularized l(infinity) linear model is derived, which meets the upper-bound in the existing literature. Compared to the current practice, the case study shows that our proposed method significantly reduces the maximum gap between two fuselages after shape adjustments.
更多查看译文
关键词
Assembly processes, Dimensional variations, Linear regression, Sparsity
AI 理解论文
溯源树
样例
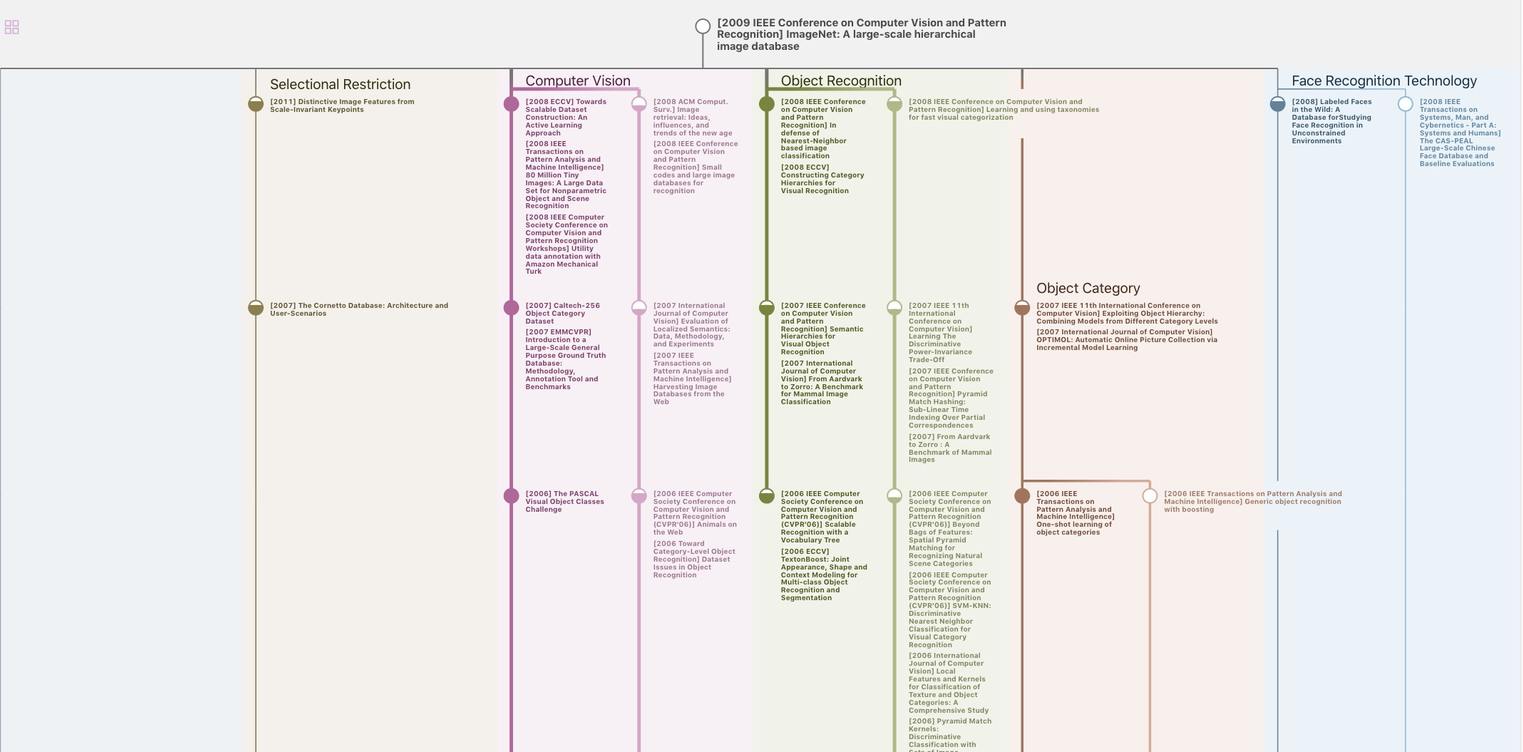
生成溯源树,研究论文发展脉络
Chat Paper
正在生成论文摘要