Knowledge Distillation in Quantum Neural Network Using Approximate Synthesis.
ASP-DAC(2023)
摘要
Recent assertions of a potential advantage of Quantum Neural Network (QNN) for specific Machine Learning (ML) tasks have sparked the curiosity of a sizable number of application researchers. The parameterized quantum circuit (PQC), a major building block of a QNN, consists of several layers of single-qubit rotations and multi-qubit entanglement operations. The optimum number of PQC layers for a particular ML task is generally unknown. A larger network often provides better performance in noiseless simulations. However, it may perform poorly on hardware compared to a shallower network. Because the amount of noise varies amongst quantum devices, the optimal depth of PQC can vary significantly. Additionally, the gates chosen for the PQC may be suitable for one type of hardware but not for another due to compilation overhead. This makes it difficult to generalize a QNN design to wide range of hardware and noise levels. An alternate approach is to build and train multiple QNN models targeted for each hardware which can be expensive. To circumvent these issues, we introduce the concept of knowledge distillation in QNN using approximate synthesis. The proposed approach will create a new QNN network with (i) a reduced number of layers or (ii) a different gate set without having to train it from scratch. Training the new network for a few epochs can compensate for the loss caused by approximation error. Through empirical analysis, we demonstrate ~71.4% reduction in circuit layers, and still achieve ~16.2% better accuracy under noise.
更多查看译文
关键词
quantum neural network,neural network,knowledge
AI 理解论文
溯源树
样例
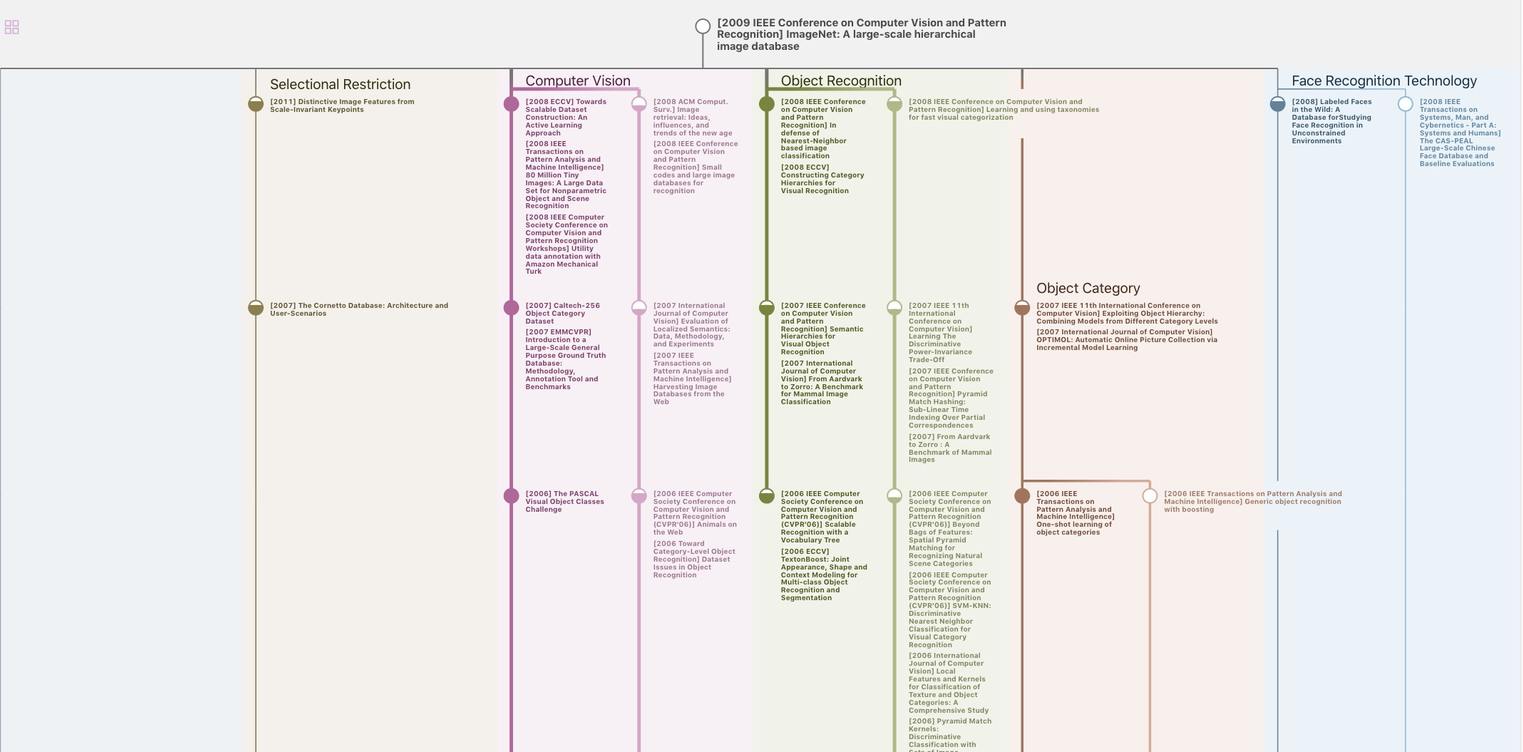
生成溯源树,研究论文发展脉络
Chat Paper
正在生成论文摘要