Hierarchical Symbolic Reasoning in Hyperbolic Space for Deep Discriminative Models
arxiv(2022)
摘要
Explanations for \emph{black-box} models help us understand model decisions as well as provide information on model biases and inconsistencies. Most of the current explainability techniques provide a single level of explanation, often in terms of feature importance scores or feature attention maps in input space. Our focus is on explaining deep discriminative models at \emph{multiple levels of abstraction}, from fine-grained to fully abstract explanations. We achieve this by using the natural properties of \emph{hyperbolic geometry} to more efficiently model a hierarchy of symbolic features and generate \emph{hierarchical symbolic rules} as part of our explanations. Specifically, for any given deep discriminative model, we distill the underpinning knowledge by discretisation of the continuous latent space using vector quantisation to form symbols, followed by a \emph{hyperbolic reasoning block} to induce an \emph{abstraction tree}. We traverse the tree to extract explanations in terms of symbolic rules and its corresponding visual semantics. We demonstrate the effectiveness of our method on the MNIST and AFHQ high-resolution animal faces dataset. Our framework is available at \url{https://github.com/koriavinash1/SymbolicInterpretability}.
更多查看译文
关键词
hierarchical symbolic reasoning,discriminative models,hyperbolic space
AI 理解论文
溯源树
样例
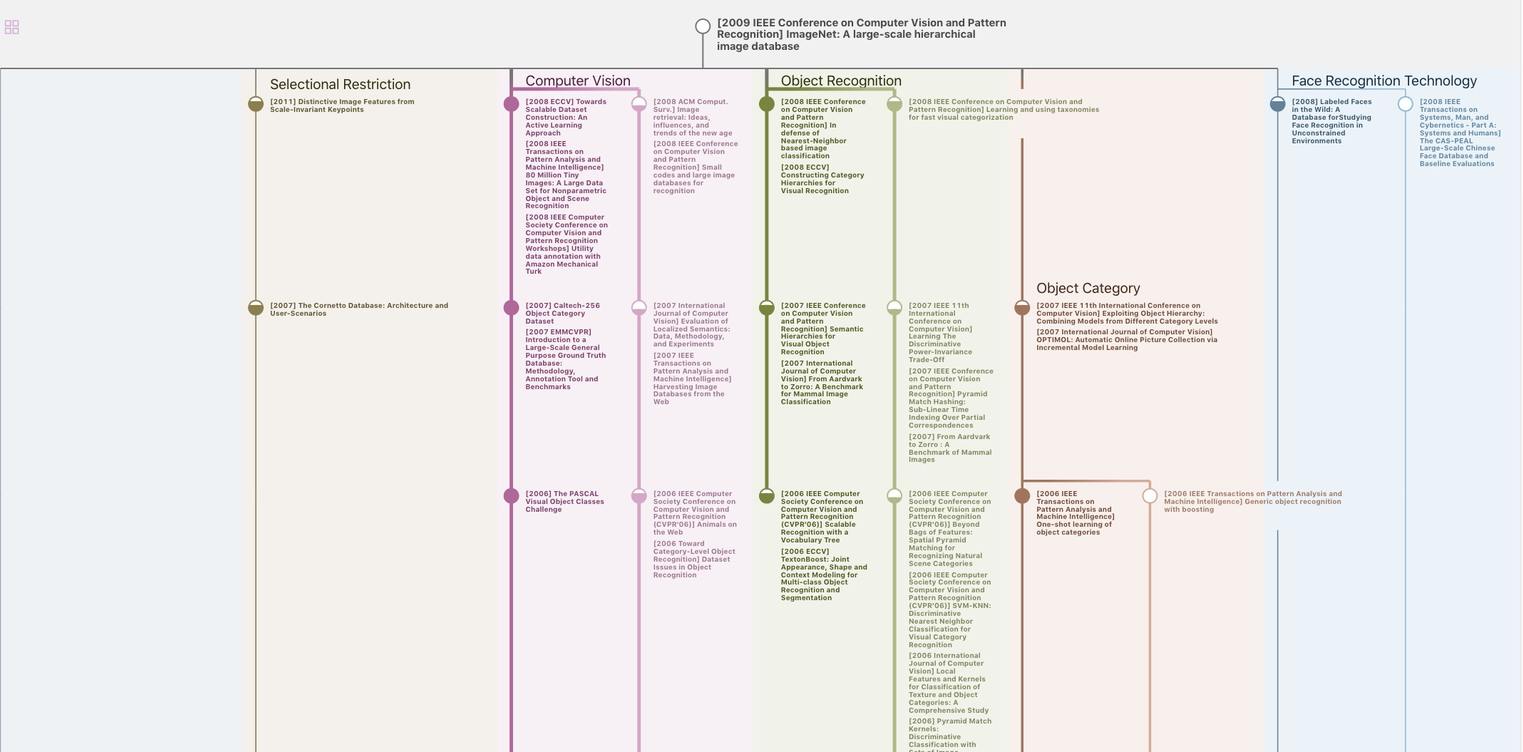
生成溯源树,研究论文发展脉络
Chat Paper
正在生成论文摘要