An Intrusion Detection System Based on Deep Belief Networks.
International Conference on Science of Cyber Security (SciSec)(2022)
摘要
The rapid growth of connected devices has led to the proliferation of novel cyber-security threats known as zero-day attacks. Traditional behaviour-based IDS rely on DNN to detect these attacks. The quality of the dataset used to train the DNN plays a critical role in the detection performance, with underrepresented samples causing poor performances. In this paper, we develop and evaluate the performance of DBN on detecting cyber-attacks within a network of connected devices. The CICIDS2017 dataset was used to train and evaluate the performance of our proposed DBN approach. Several class balancing techniques were applied and evaluated. Lastly, we compare our approach against a conventional MLP model and the existing state-of-the-art. Our proposed DBN approach shows competitive and promising results, with significant performance improvement on the detection of attacks underrepresented in the training dataset.
更多查看译文
关键词
Network Intrusion Detection System, Deep learning, Deep Belief Networks, Class balancing
AI 理解论文
溯源树
样例
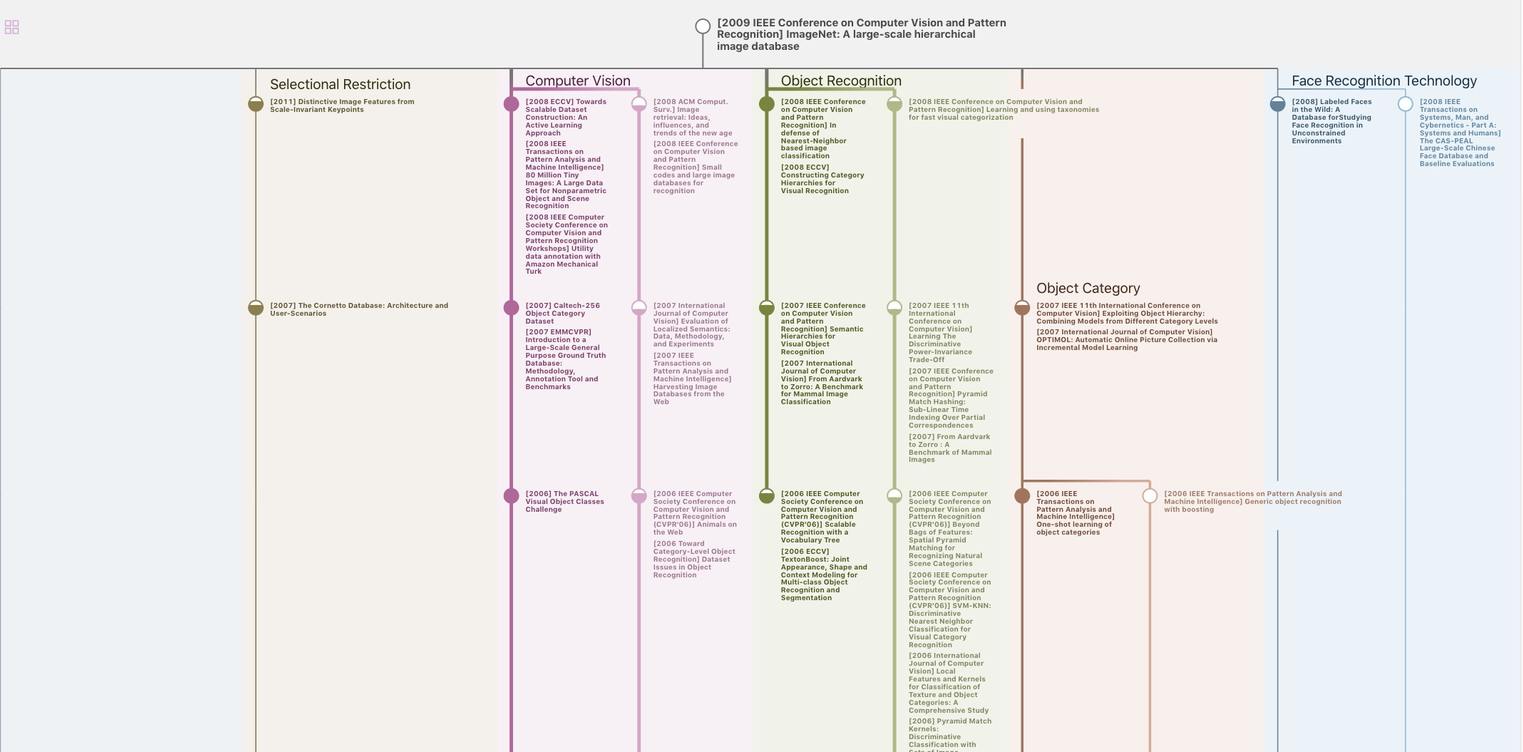
生成溯源树,研究论文发展脉络
Chat Paper
正在生成论文摘要