A survey on the interpretability of deep learning in medical diagnosis
Multim. Syst.(2022)
摘要
Deep learning has demonstrated remarkable performance in the medical domain, with accuracy that rivals or even exceeds that of human experts. However, it has a significant problem that these models are “black-box” structures, which means they are opaque, non-intuitive, and difficult for people to understand. This creates a barrier to the application of deep learning models in clinical practice due to lack of interpretability, trust, and transparency. To overcome this problem, several studies on interpretability have been proposed. Therefore, in this paper, we comprehensively review the interpretability of deep learning in medical diagnosis based on the current literature, including some common interpretability methods used in the medical domain, various applications with interpretability for disease diagnosis, prevalent evaluation metrics, and several disease datasets. In addition, the challenges of interpretability and future research directions are also discussed here. To the best of our knowledge, this is the first time that various applications of interpretability methods for disease diagnosis have been summarized.
更多查看译文
关键词
Deep learning,Interpretability methods,Applications,Disease diagnosis
AI 理解论文
溯源树
样例
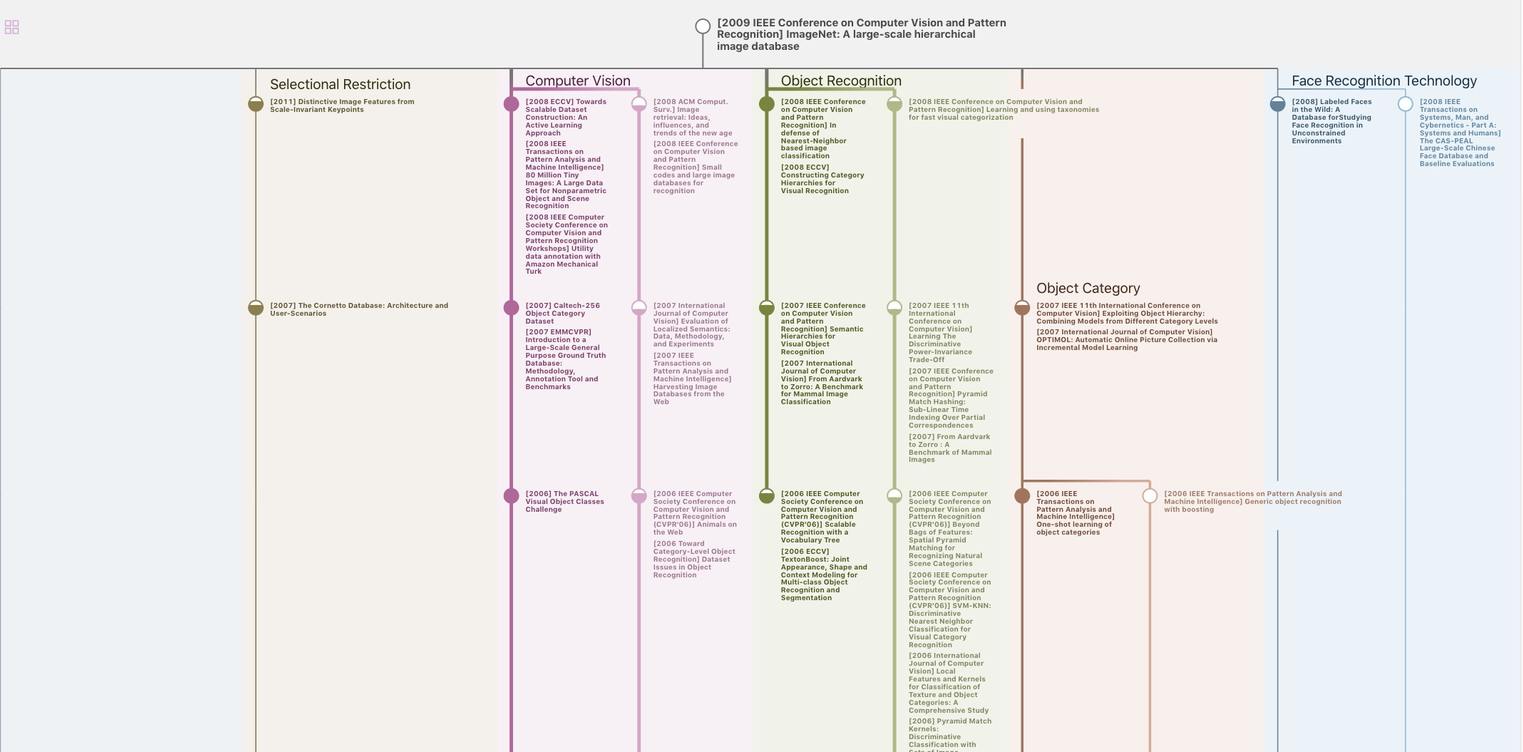
生成溯源树,研究论文发展脉络
Chat Paper
正在生成论文摘要