Explaining in the Presence of Vocabulary Mismatch
Explainable Human-AI Interaction(2022)
摘要
All previous discussions on model-reconciliation explanations implicitly assume that the robot can communicate information about the model to the user. This suggests that the human and the robot share a common vocabulary that can be used to describe the model. However, this cannot be guaranteed unless the robots are using models that are specified by an expert. Since many of the modern AI systems rely on learned models, they may use representational schemes that would be inscrutable to most users. So in this chapter, we will focus on the question of generating model reconciliation explanations in the absence of shared vocabulary. Specifically, we will see how one could identify relevant model information in terms of concepts specified by a user. We will describe how the agent could use classifiers learned over such concepts to identify fragments of symbolic models specified in terms of such concepts that are sufficient to provide explanations. We will also discuss how one could measure the probability of the generated model fragments being true (especially when the learned classifiers may be noisy) and also how to identify cases where the user specified concepts may be insufficient.
更多查看译文
关键词
vocabulary mismatch
AI 理解论文
溯源树
样例
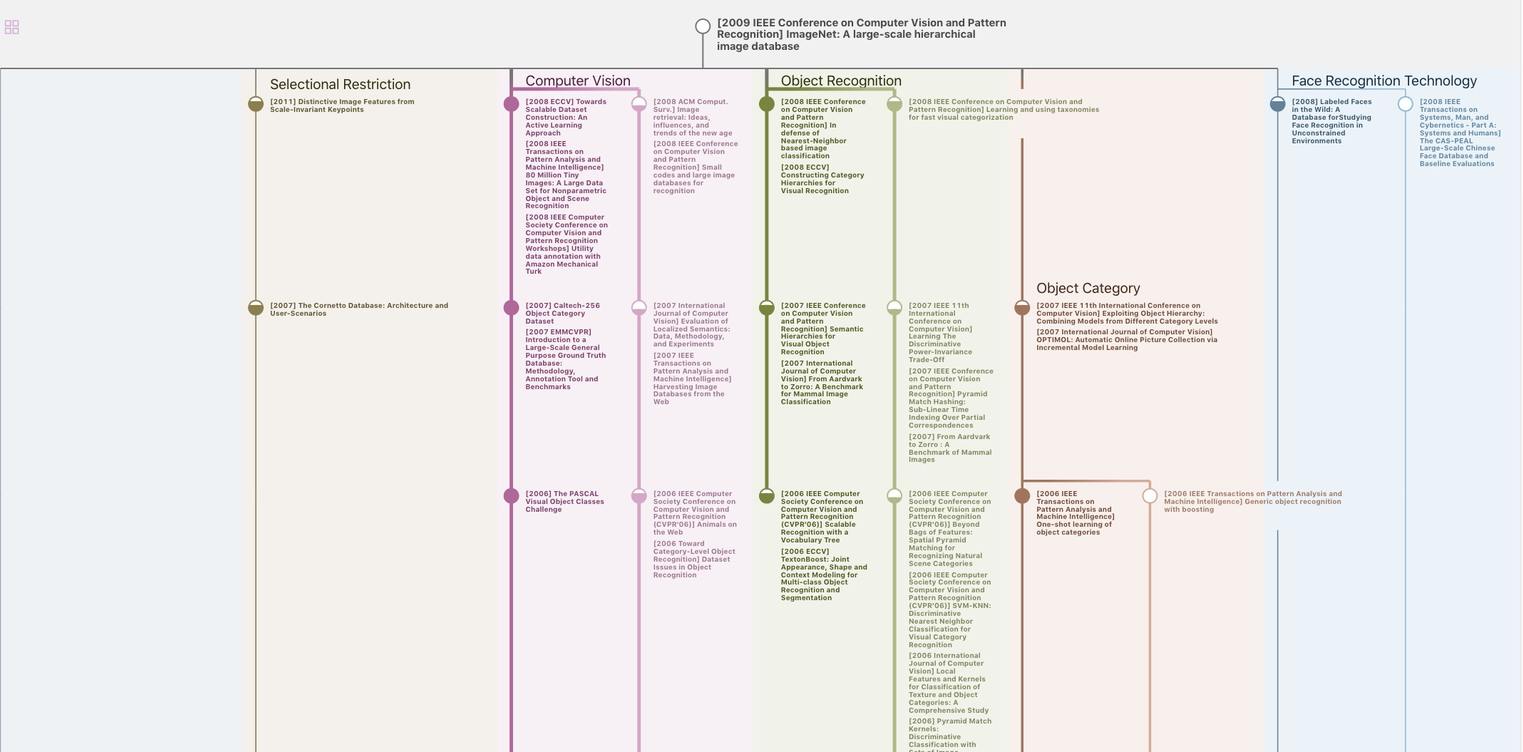
生成溯源树,研究论文发展脉络
Chat Paper
正在生成论文摘要