Travel Time Prediction Method Based on Spatial-Feature-based Hierarchical Clustering and Deep Multi-input Gated Recurrent Unit
ACM Transactions on Sensor Networks(2022)
摘要
Accurate travel time prediction (TTP) is a significant aspect in the intelligent transportation system (ITS). Travel times of certain road segments explicitly reflect the traffic conditions of those sections. Effective TTP of road segments is instrumental in route planning, traffic control, and traffic management. However, the accuracy of TTP is greatly affected by the intricate topological structure of traffic network and the dynamics of traffic flow over time. This paper develops a TTP method based on the spatial-feature-based hierarchical clustering (SFHC) and deep multi-input gated recurrent unit (DMGRU). The proposed two-stage method is capable of capturing the spatial-temporal features of traffic network. Specifically, the SFHC divides the road segments into several clusters having similar traffic features, and then the clustered data is fed into the DMGRU for TTP. Our experiments conducted on the practical dataset demonstrate that the designed prediction method can achieve the mean absolute percentage error (MAPE) of 3.3109% and mean absolute error (MAE) of 2.5658, which outperform various combinations of baseline clustering algorithms and prediction models.
更多查看译文
关键词
Travel time prediction,segmented regression analysis,hierarchical clustering,gated recurrent unit
AI 理解论文
溯源树
样例
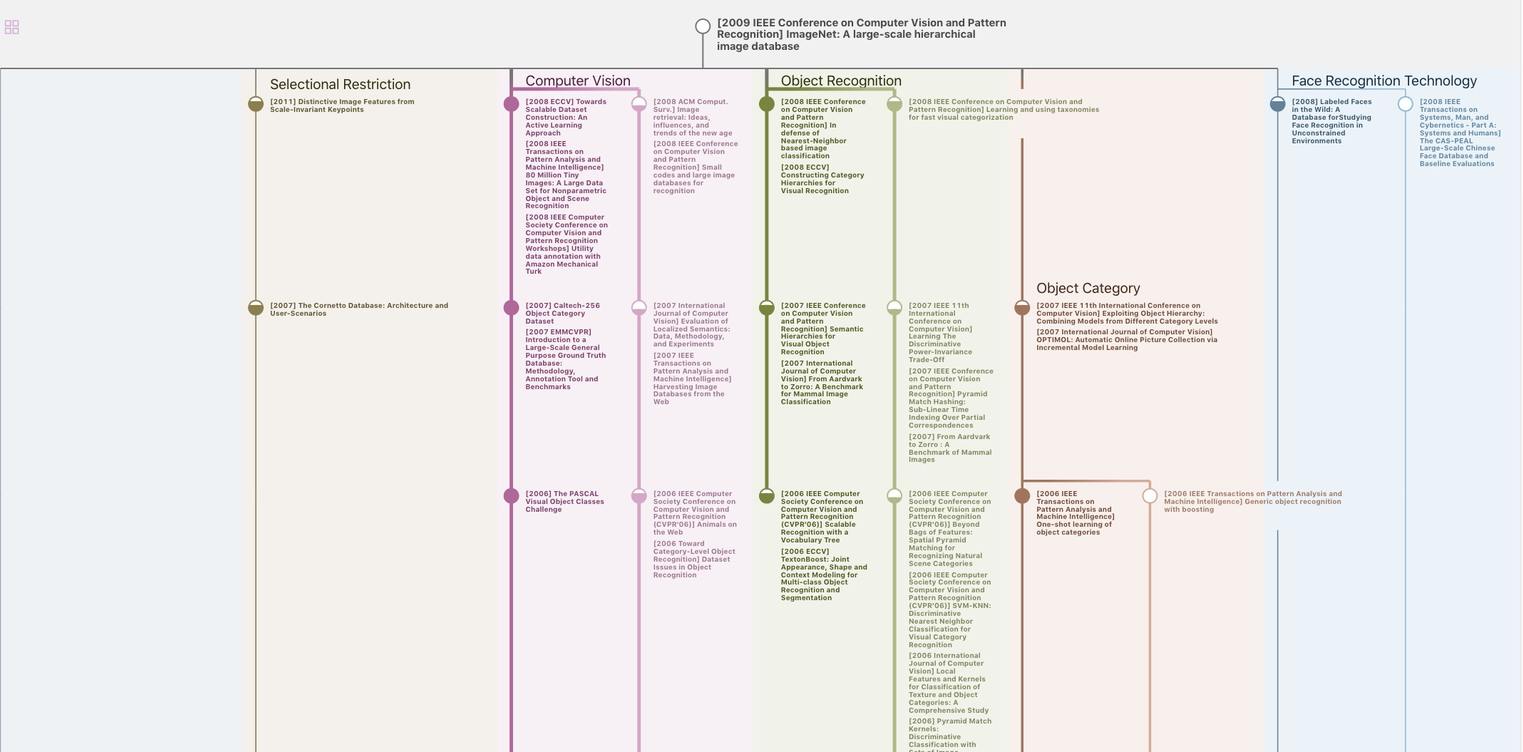
生成溯源树,研究论文发展脉络
Chat Paper
正在生成论文摘要