Abstract 459: Identification of the PDAC immunogenic subtype using deep learning with multi-scaled digital H&E images
Cancer Research(2022)
摘要
Abstract Background: Pancreatic ductal adenocarcinoma (PDAC) has proven a difficult cancer to treat. To improve treatment strategies, molecular subtypes have been identified, one being immunogenic with significant immune infiltrate and better prognosis. These subtypes have been determined by RNA profiling, which often exhibits technical challenges in a clinical setting. Here we present a deep learning-based method to identify PDAC immunogenic patients using H&E images. This method is robust to RNA quality issues and can elucidate unique morphological features not obtainable with RNA profiling to refine the selection of patients with this phenotype. Methods: Patients (n=265) were primarily stage II or IV, treated previously with gemcitabine+nab-paclitaxel or FOLFIRINOX. Ground truth for immunogenic subtype (n=105) was defined with RNASeq. Whole slide images were subdivided into non-overlapping, fixed-size tiles at 4 magnifications: 2.5x, 5x, 10x, 20x, followed by feature extraction using a ResNet50 convolutional neural network. Principal component (PC) analysis reduced dimensionality of extracted features in each tile. The dataset was split into 80% training, 20% testing. Posterior probabilities from a linear model were inputs to a support vector machine to predict outcome (2-step model). Progression- free survival (PFS) was evaluated using the Kaplan Meier method. Results: ImageNet-trained ResNet50 model extracted 2048 features from each tile and the first 75 PCs, explaining 87% variance, were input into the 2-step model; average AUC=0.77 (95% CI=0.70,0.83) across the 4 magnification datasets. A multi-scale ensemble approach combining these 4 magnifications improved the AUC to 0.80 (95% CI=0.66,0.93). Accuracy, sensitivity, and specificity were 0.79, 0.76, and 0.81, respectively. The predicted immunogenic subtype showed significantly improved PFS compared to the other 3 subtypes (HR 95%CI = 0.54 (0.36,0.81), p=0.003), while the RNASeq-derived immunogenic subtype had less differentiation (HR 95%CI = 0.64 (0.42,0.98), p=0.04). Conclusions: This study presents a deep learning 2-step model approach using tumor H&E images to identify PDAC immunogenic subtype, with improved prognostic potential to that identified by RNA profiling, suggesting possible application in clinical settings for patient stratification. Future work will expand the model to larger independent cohorts. Citation Format: Han Si, Steven Xu, Anantharaman Muthuswamy, Ryan Liang, Kate Sasser, Hisham K. Hamadeh, Suzana Couto, Brandon Higgs. Identification of the PDAC immunogenic subtype using deep learning with multi-scaled digital H&E images [abstract]. In: Proceedings of the American Association for Cancer Research Annual Meeting 2022; 2022 Apr 8-13. Philadelphia (PA): AACR; Cancer Res 2022;82(12_Suppl):Abstract nr 459.
更多查看译文
AI 理解论文
溯源树
样例
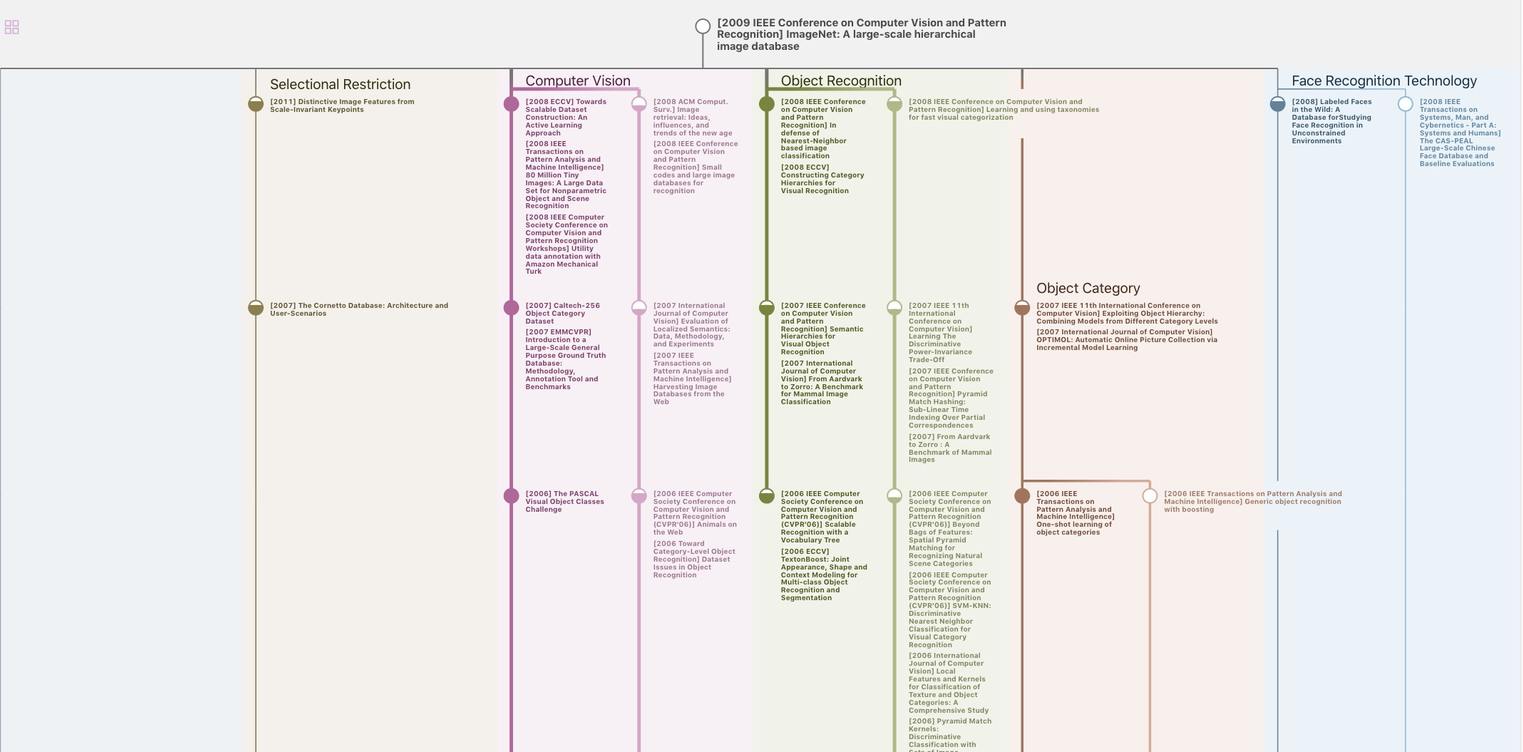
生成溯源树,研究论文发展脉络
Chat Paper
正在生成论文摘要