Multiparametric analysis in GBM plasma extracellular vesicles (Evs) and surface marker expression profile.
Journal of Clinical Oncology(2022)
摘要
2038 Background: Glioblastoma (GBM) is the most common malignant brain tumor with poor clinical prognosis. Management of GBM is hampered by the lack of an accurate test that can be used for differential diagnosis of tumor progression from inflammatory pseudoprogression. Plasma extracellular vesicles (EVs) has been shown to be a promising source for biomarker identification. In this project, we aimed to identify GBM plasma EV markers that could serve as the basis of a liquid biopsy. Methods: Sample preparation, assay controls and instrument calibration were performed following MIFlowCyt-EV guideline. Plasma samples were subjected to 2-step centrifugations to remove cell debris and platelets. 10ul of plasma sample was diluted with 90ul filtered PBS, then stained for EV surface markers including CD9, CD31, CD45, CD41a and CD11b, as well as actin phalloidin. Stained plasma samples were purified using IZON qEV1/70nm column, then EV fractions were analyzed using full spectrum Cytek Aurora flow cytometer. Clustering analysis was performed on EV events (CD9 +/ actin phalloidin -) using t-SNE and FlowSOM extensions from FlowJo plugins. Results: Compared to normal donors, GBM plasma EVs were bigger in size (higher SSC value) and expressed higher levels of CD9, CD31, CD45 and CD11b while ND plasma EVs had higher CD41a expression. t-SNE and FlowSOM analysis demonstrated that GBM plasma EVs had a unique surface marker expression profile compared to ND EVs. It also showed 10 EV sub-populations that differed in size as well as various surface marker expression levels. Four of these subpopulations were enriched in GBM EVs, while three of these were enriched in ND EVs. Conclusions: This multiparametric analysis revealed that GBM plasma EVs had a unique surface marker expression profile compared to ND plasma EVs. Further separation and molecular profiling analysis based on each sub population could reveal EV biomarkers that are unique to each sample population.
更多查看译文
AI 理解论文
溯源树
样例
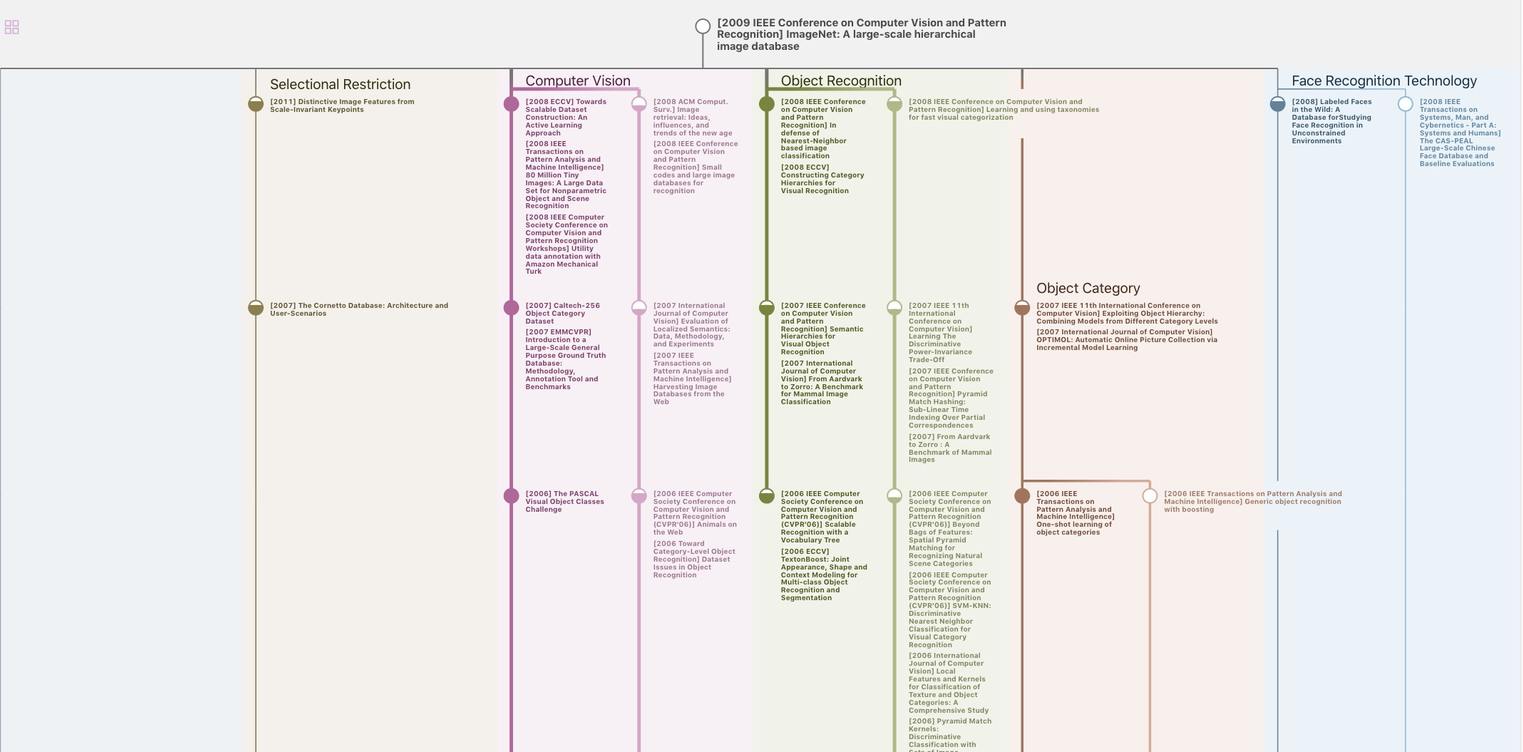
生成溯源树,研究论文发展脉络
Chat Paper
正在生成论文摘要