Optimal Survey Design for Forest Carbon Monitoring in Remote Regions Using Multi-Objective Mathematical Programming
FORESTS(2022)
摘要
Cost-effective monitoring of forest carbon resources is critical to the development of national policies and enforcement of international agreements aimed at reducing carbon emissions and mitigating the impacts of climate change. While carbon monitoring systems are often based on national forest inventories (NFI) utilizing a large sample of field plots, in remote regions the lack of transportation infrastructure often requires heavier reliance on remote sensing technologies, such as airborne lidar. The challenge motivating our research is that the efficacy of estimating carbon with lidar varies across the various carbon pools within forest ecosystems. Lidar measurements are typically highly correlated with aboveground tree carbon but are less strongly correlated with other carbon pools, such as down woody materials (DWM) and soil. Field measurements are essential to both (1) estimate soil and DWM carbon directly and (2) develop regression models to estimate tree carbon indirectly using lidar. With limited budgets and time, however, decision makers must find an optimal way to combine field measurements with lidar to minimize standard errors in carbon estimates for the various pools. We introduce a multi-objective binary programming formulation that quantifies the tradeoffs behind the competing objectives of minimizing standard errors for tree carbon, DWM carbon, and soil carbon. Using NFI and airborne lidar data from a remote boreal forest region of interior Alaska, we demonstrate the operational feasibility of the method and suggest that it is generalizable to other carbon sampling projects because of its generic mathematical structure.
更多查看译文
关键词
optimal sampling design,forest carbon,multi-objective binary programming,tradeoff analysis,lidar
AI 理解论文
溯源树
样例
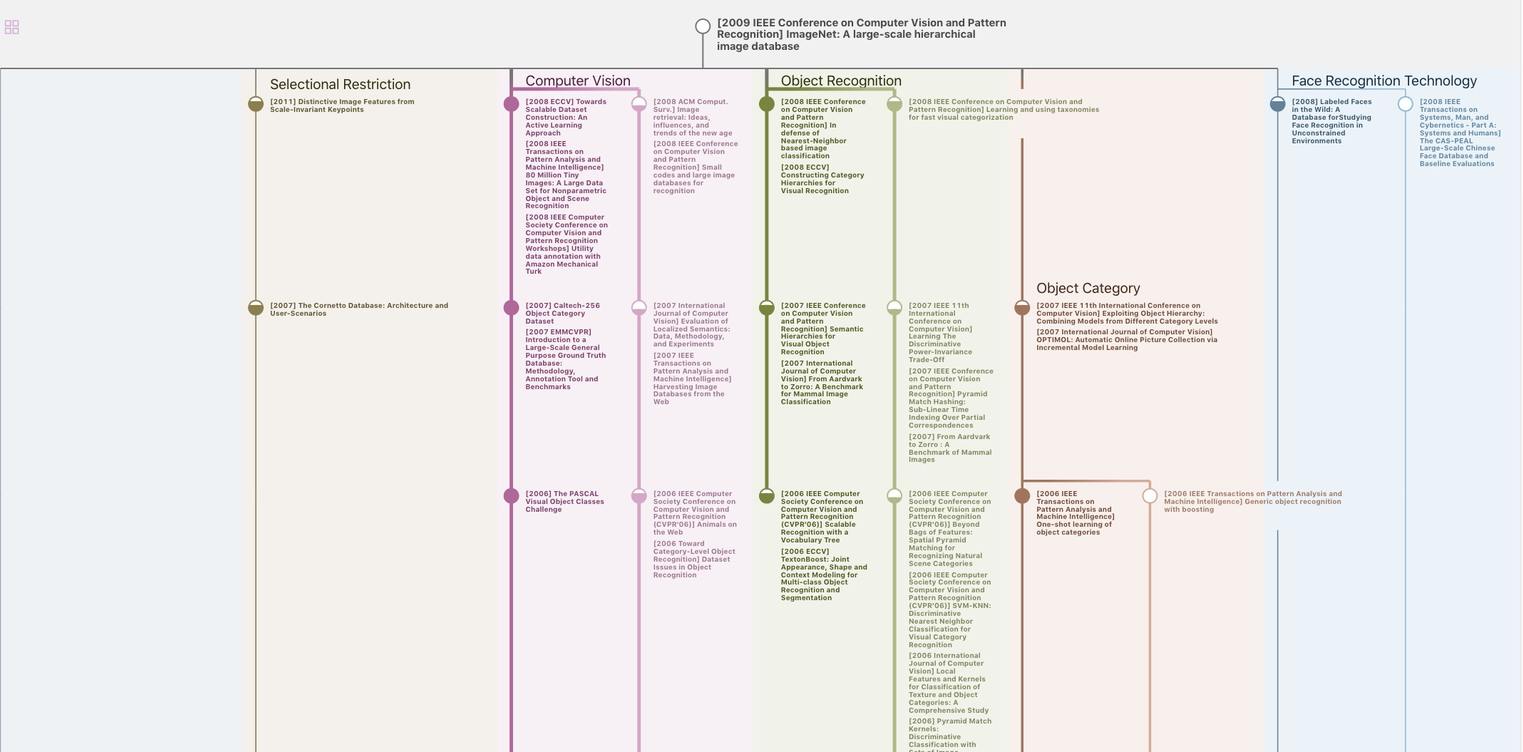
生成溯源树,研究论文发展脉络
Chat Paper
正在生成论文摘要