Deep Neural Networks for Direction of Arrival Estimation of Multiple Targets with Sparse Prior for Line-of-Sight Scenarios
IEEE TRANSACTIONS ON VEHICULAR TECHNOLOGY(2023)
Abstract
Received signal Direction of Arrival (DOA) estimation represents a significant problem with multiple applications, ranging from wireless communications to radars. This problem presents significant challenges, mainly given by a large number of closely located transmitters being difficultly separable. Currently available state of the art approaches fail in providing sufficient resolution to separate and recognize the DOA of closely located transmitters, unless using a large number of antennas and hence increasing the deployment and operation costs. In this paper, we present a deep learning framework for DOA estimation under Line-of-Sight scenarios, which able to distinguish a number of closely located sources higher than the number of receivers' antennas. We first propose a formulation that maps the received signal to a higher dimensional space that allows for better identification of signal sources. Secondly, we introduce a Deep Neural Network that learns the mapping from the receiver antenna space to the extended space to avoid relying on specific receiver antenna array structures. Thanks to our approach, we reduce the hardware complexity compared to state of the art solutions and allow reconfigurability of the receiver channels. Via extensive numerical simulations, we demonstrate the superiority of our proposed method compared to state-of-the-art deep learning-based DOA estimation methods, especially in demanding scenarios with low Signal-to-Noise Ratio and limited number of snapshots.
MoreTranslated text
Key words
Direction-of-arrival estimation,Antenna arrays,Estimation,Receiving antennas,Costs,Deep learning,Covariance matrices,DOA estimation,deep neural network,sparse representation,multiple targets
PDF
View via Publisher
AI Read Science
AI Summary
AI Summary is the key point extracted automatically understanding the full text of the paper, including the background, methods, results, conclusions, icons and other key content, so that you can get the outline of the paper at a glance.
Example
Background
Key content
Introduction
Methods
Results
Related work
Fund
Key content
- Pretraining has recently greatly promoted the development of natural language processing (NLP)
- We show that M6 outperforms the baselines in multimodal downstream tasks, and the large M6 with 10 parameters can reach a better performance
- We propose a method called M6 that is able to process information of multiple modalities and perform both single-modal and cross-modal understanding and generation
- The model is scaled to large model with 10 billion parameters with sophisticated deployment, and the 10 -parameter M6-large is the largest pretrained model in Chinese
- Experimental results show that our proposed M6 outperforms the baseline in a number of downstream tasks concerning both single modality and multiple modalities We will continue the pretraining of extremely large models by increasing data to explore the limit of its performance
Try using models to generate summary,it takes about 60s
Must-Reading Tree
Example
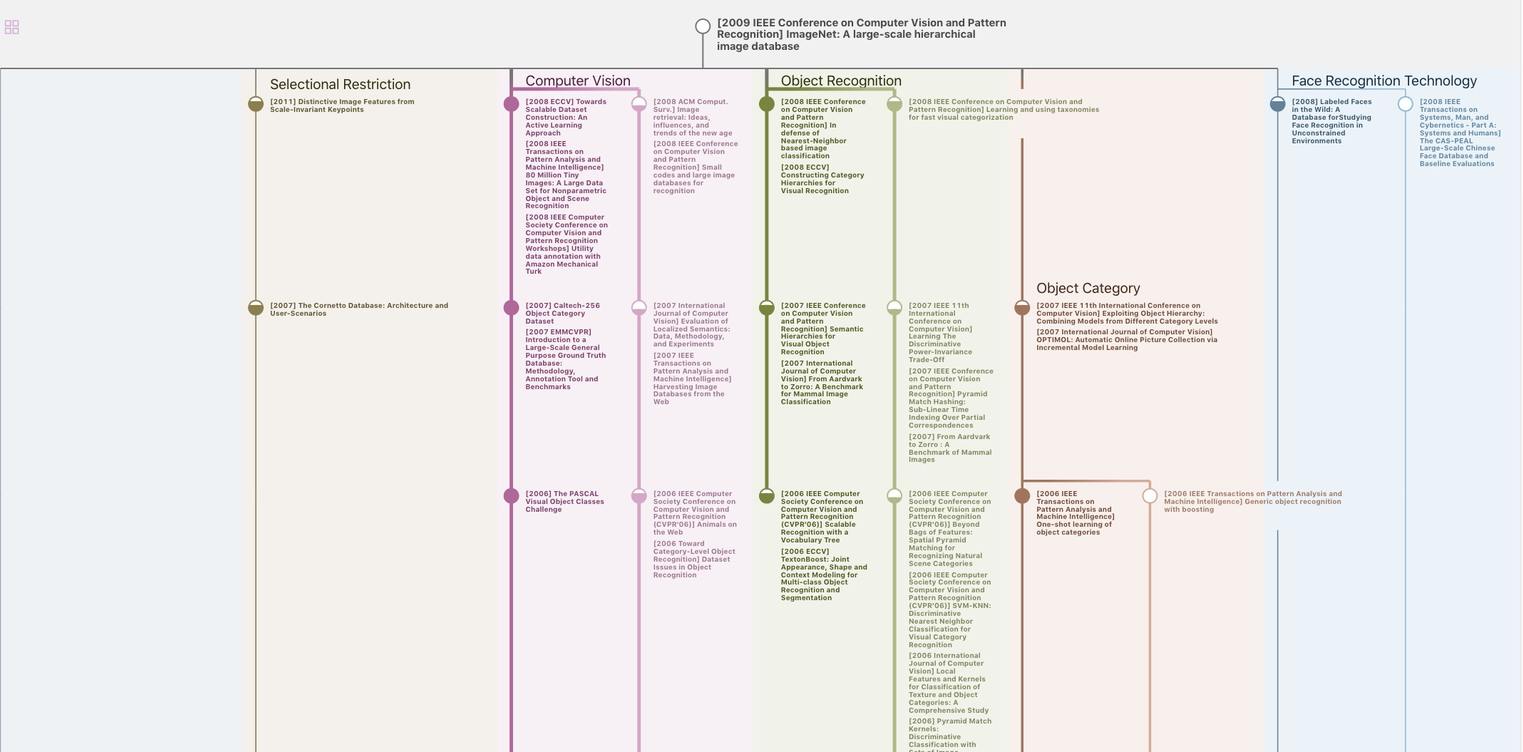
Generate MRT to find the research sequence of this paper
Related Papers
2013
被引用119 | 浏览
2005
被引用119 | 浏览
2007
被引用84 | 浏览
2010
被引用59 | 浏览
2017
被引用47 | 浏览
2019
被引用213 | 浏览
2018
被引用30 | 浏览
Direction-of-Arrival Estimation Based on Deep Neural Networks with Robustness to Array Imperfections
2018
被引用331 | 浏览
2019
被引用25 | 浏览
2021
被引用19 | 浏览
2021
被引用18 | 浏览
Data Disclaimer
The page data are from open Internet sources, cooperative publishers and automatic analysis results through AI technology. We do not make any commitments and guarantees for the validity, accuracy, correctness, reliability, completeness and timeliness of the page data. If you have any questions, please contact us by email: report@aminer.cn
Chat Paper
GPU is busy, summary generation fails
Rerequest