146 Ct radiomics in carotid artery atherosclerosis: a systematic evaluation of robustness, reproducibility and predictive performance for culprit lesions
Imaging(2022)
摘要
IntroductionRadiomics is the extraction and quantification of simple and complex patterns in medical images for diagnosis and prognosis prediction. However, clinical adoption may be limited by variability in imaging workflows. This study aimed to identify those radiomic features most immune to variability, with best potential for integration into practice.MethodsWe assessed the robustness to variability of 93 radiomic features, derived from the carotid CT angiograms of 41 patients with previous stroke and TIA. Morphological operations were then applied to regions-of-interest (involving a single CT slice, ROI) and/or volumes-of-interest (involving consecutive CT slices, VOI) drawn around the carotid artery to simulate intra- and inter-observer variability. These operations were conducted using a variety of real-world imaging settings: ± normalisation, ± resegmentation, different image quantisation and resampling methods. We sought to identify the optimal image settings (i.e. providing the highest proportion of radiomic features with excellent robustness and the most robust and non-redundant radiomics features) for the identification of culprit and non-culprit carotid arteries in symptomatic patients using several machine learning algorithms. We report the average area under the curve (AUC) from five-fold cross validation.ResultsThe proportion of radiomic features with excellent robustness varied depending on the image settings used. Not all the 93 radiomic features were robust against ROI/VOI morphological perturbations. Prior normalisation of CT imaging did not increase the proportion of robust radiomic features but using a multiple slice approach was superior to a single CT slice approach for producing robust features (67/93 vs. 61/93). Grey value range image resegmentation, which restricted radiomic feature calculations to Hounsfield units between 0 and 200 inclusive, reduced the proportion of poorly robust radiomic features. The optimal image quantisation method was a fixed bin width of 25 or 30. The top 10 non-redundant radiomic features and carotid calcium were then used as predictors to identify culprit carotid arteries in symptomatic patients. The ElasticNet model achieved the highest performance with a mean AUC (SD) of 0.73 (0.09) amongst the machine learning algorithms investigated: decision tree 0.58 (0.19), random forest 0.67. (0.08), LASSO 0.72 (0.09), neural network 0.60 (0.09) and XGBoost 0.56 (0.09). Using carotid calcification alone had a poor predictive performance with mean AUC (SD) of 0.44 (0.11). CONCLUSION/IMPLICATIONSHere, we highlight factors in the radiomics workflow that impact robustness. We identified the optimal image settings to minimise variability and finally we revealed a robust radiomic feature set that identified culprit carotid lesions in patients with stroke and transient ischaemic attack with good accuracy. The study provides key information for future carotid CT radiomic studies.Conflict of InterestNone to declare
更多查看译文
关键词
carotid artery atherosclerosis,ct radiomics
AI 理解论文
溯源树
样例
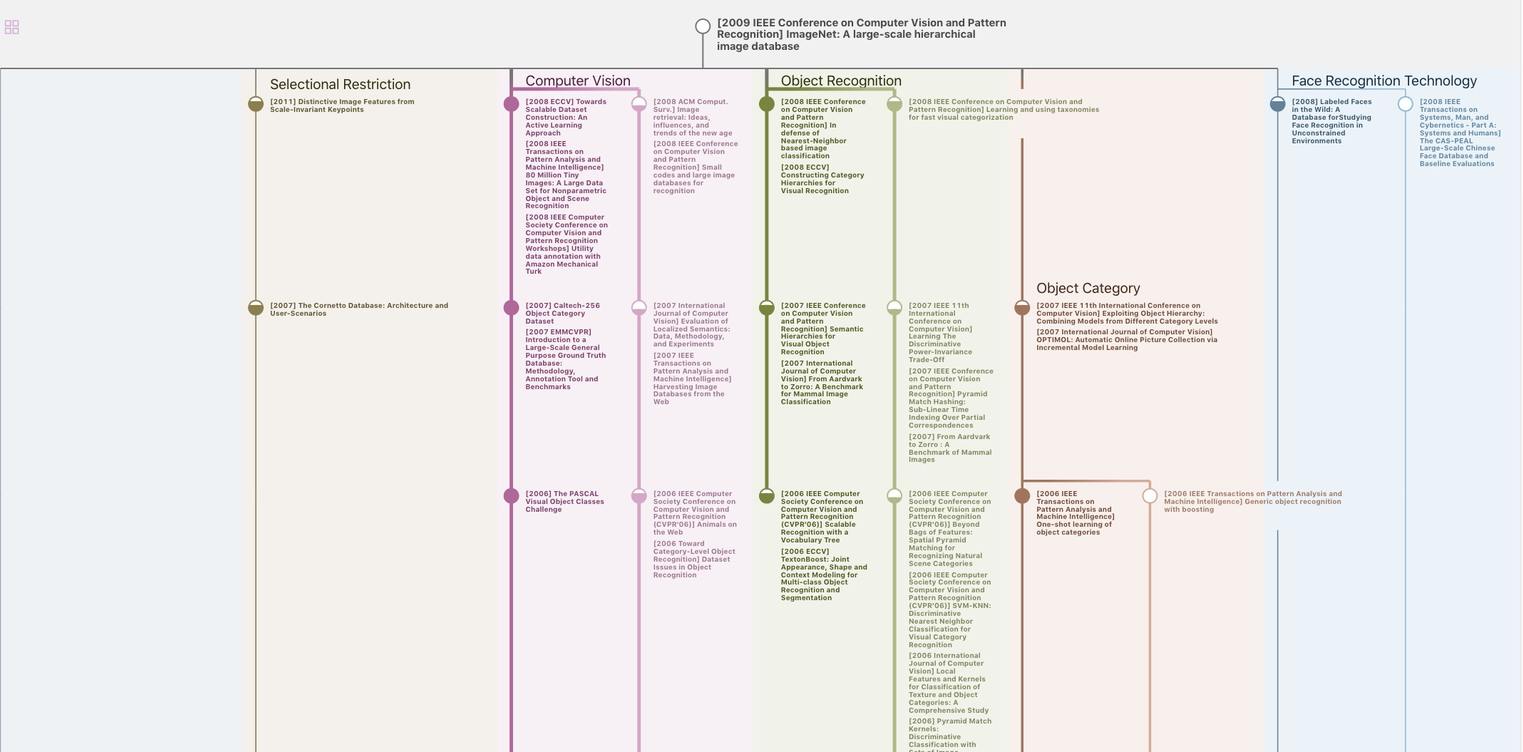
生成溯源树,研究论文发展脉络
Chat Paper
正在生成论文摘要