Semi-Supervised Cloud Detection in Satellite Images by Considering the Domain Shift Problem
REMOTE SENSING(2022)
摘要
In terms of semi-supervised cloud detection work, efforts are being made to learn a promising cloud detection model via a limited number of pixel-wise labeled images and a large number of unlabeled ones. However, remote sensing images obtained from the same satellite sensor often show a data distribution drift problem due to the different cloud shapes and land-cover types on the Earth's surface. Therefore, there are domain distribution gaps between labeled and unlabeled satellite images. To solve this problem, we take the domain shift problem into account for the semi-supervised learning (SSL) network. Feature-level and output-level domain adaptations are applied to reduce the domain distribution gaps between labeled and unlabeled images, thus improving predicted results accuracy of the SSL network. Experimental results on Landsat-8 OLI and GF-1 WFV multispectral images demonstrate that the proposed semi-supervised cloud detection network (SSCDnet) is able to achieve promising cloud detection performance when using a limited number of labeled samples and outperforms several state-of-the-art SSL methods.
更多查看译文
关键词
remote sensing imagery,cloud detection,semi-supervised learning,distribution drift,domain shift problem,domain adaptation
AI 理解论文
溯源树
样例
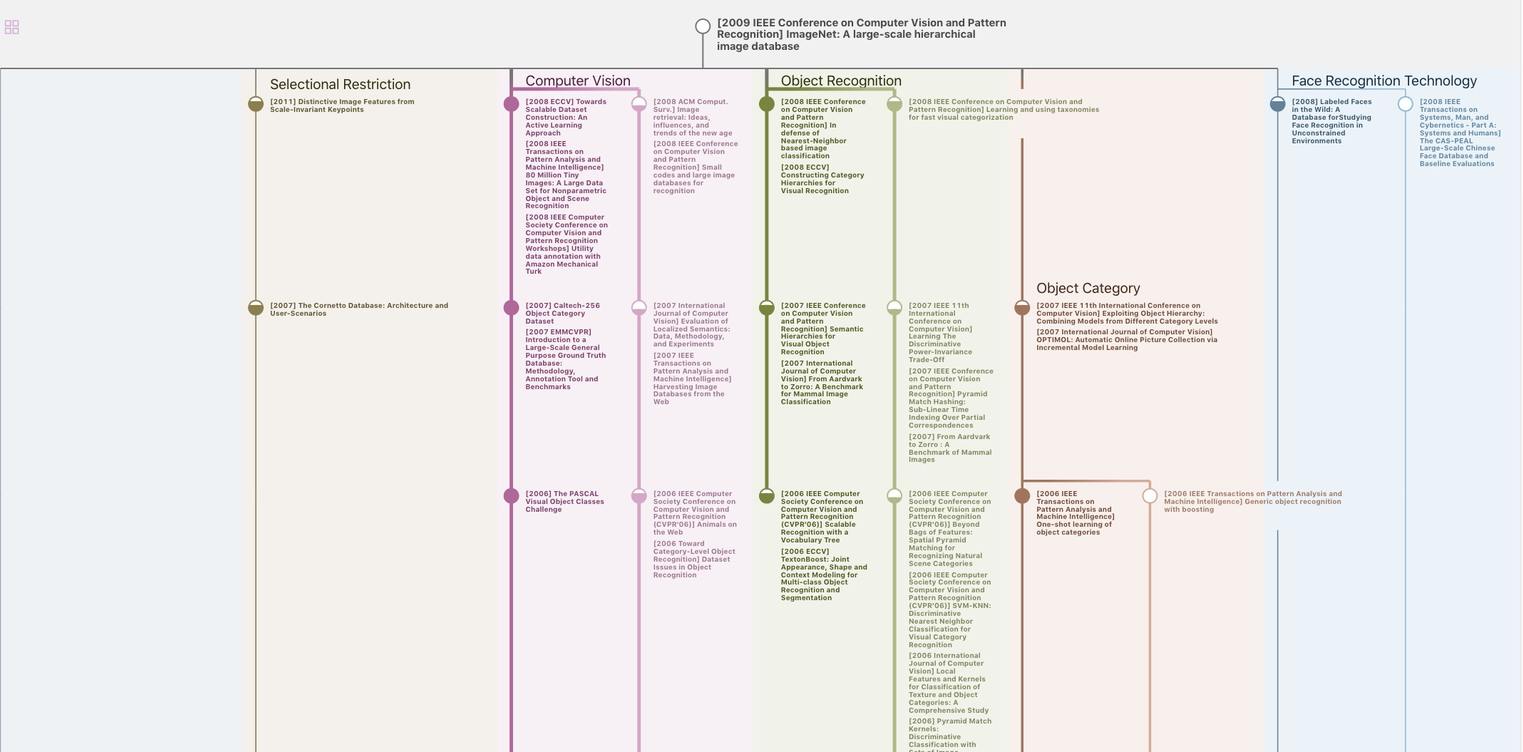
生成溯源树,研究论文发展脉络
Chat Paper
正在生成论文摘要