Artificial intelligence approach for modeling petroleum refinery catalytic desulfurization process
Neural Computing and Applications(2022)
摘要
Frequently, petroleum refineries create a variety of fuels as well as a vast range of chemicals for diverse applications. One of the most frequent procedures for purifying petroleum products from unwanted sulfur species and reducing SO 2 emissions is the hydrodesulfurization (HDS) process. However, HDS is still challenging since a variety of factors influence sulfur removal rates, including operating circumstances, feed compositions, catalyst activity, and so on. In actuality, reducing sulfur compounds comes at a high price, both environmentally and economically. In practice, it is necessary to forecast process yields and their implications for productivity, profitability, and environmental considerations. The study of such outcomes could serve as guidance for scholars and practitioners alike. Machine Learning (ML) algorithms have proven to be effective in solving various real-world problems in engineering and industrial fields, including the petroleum industry. This study presents a four-input support vector regression (SVR) model hybridized with a Bayesian optimization to predict three yields of the HDS process including outlet sulfur concentration, percentage of SO 2 emission, and percentage of biphenyl. The proposed models are used to identify the best laboratory configuration for better optimization of the HDS process. The obtained modeling results reveal that the proposed models are competent with a high degree of accuracy. The correlation coefficients during the testing of the three models were 99.1, 99.2, and 98.8% while the average experimental errors RMSE and MRAE were 0.022 and 0.097, respectively.
更多查看译文
关键词
Machine learning,Industry,Petrochemicals,Hydrodesulfurization,Multiobjective
AI 理解论文
溯源树
样例
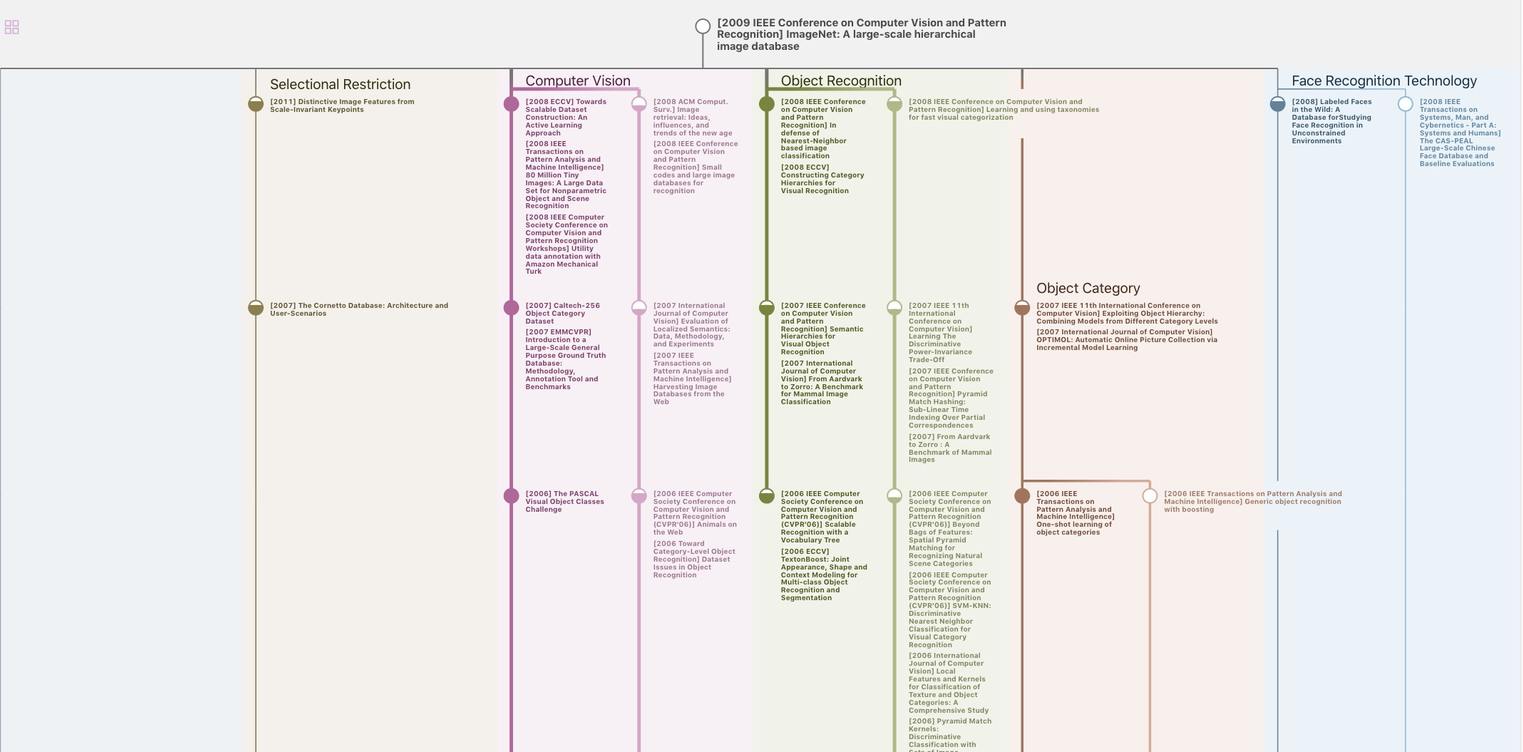
生成溯源树,研究论文发展脉络
Chat Paper
正在生成论文摘要