A Deep-Learning-Based Approach for Production Forecast and Reservoir Evaluation for Shale Gas Wells with Complex Fracture Networks
Day 4 Thu, June 09, 2022(2022)
摘要
Abstract This paper proposes a data-driven proxy model to effectively forecast the production of horizontal wells with complex fracture networks in shales. With the multilayer gated recurrent unit (GRU) cell, the proxy model is coupled with newly developed deep learning methods include attention mechanism (Att-GRU), skip connection, and cross-validation to deal with time series analysis (TSA) issue of multivariate operating and physical parameters. In the formulation, the input variables include time, variable bottom hole pressure (BHP), horizontal well length, fracture number, fracture half-length, and fracture conductivity and the output variable refers to the production corresponding to the forecast time. The sample data generated by the boundary element method (BEM) is used in the proxy model learning process. The shuffled cross-validation method is utilized to improve the model accuracy and generalization capability. Results depict that the Att-GRU can accurately forecast the production for shale gas wells with complex fracture networks at a given time and variable BHP while maintaining a high calculation efficiency. The operating and physical parameters analysis indicates that the Att-GRU has learned the underlying physical features of complex fracture networks and variable BHP. Case study from Marcellus shale shows that the proposed Att-GRU is robust in both production forecast and reservoir evaluation, and it is a potential proxy model for transient analysis.
更多查看译文
AI 理解论文
溯源树
样例
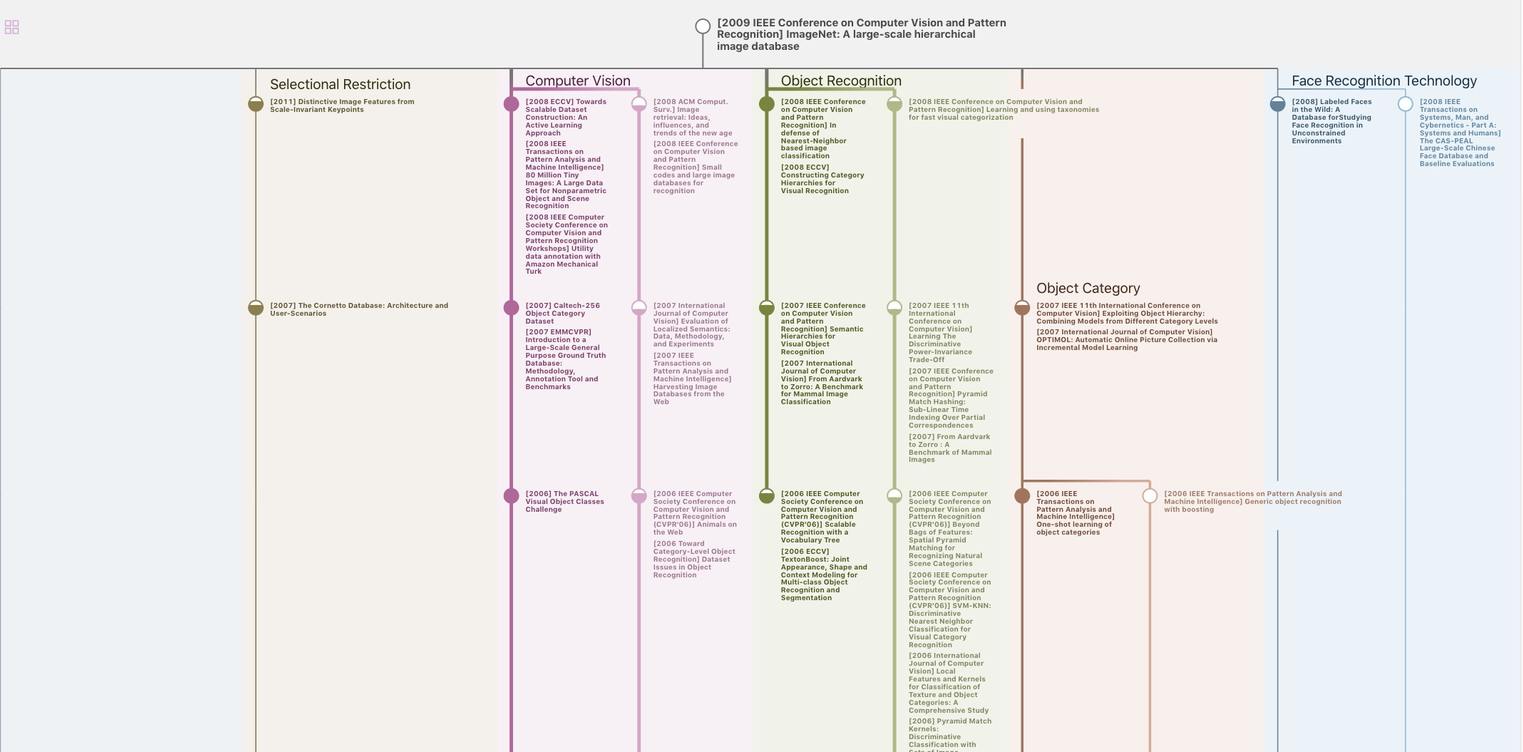
生成溯源树,研究论文发展脉络
Chat Paper
正在生成论文摘要