TEACHING NAILFOLD CAPILLAROSCOPY USING THE "FAST TRACK ALGORITHM" VIA AN ONLINE PLATFORM: THE ASIAN EXPERIENCE
Annals of the Rheumatic Diseases(2022)
摘要
BackgroundNailfold capillaroscopy (NFC) is being increasingly used in the early identification of systemic sclerosis (SSc) related disorders. Recent studies have shown that an optimised simple capillaroscopic definition of normal and abnormal capillary morphology is reliable and a multi-centre consensus ‘fast track algorithm’ was developed by the European League against Rheumatism (EULAR) Study Group on Microcirculation in Rheumatic Diseases (SG MC/RD) to allow trainees to identify scleroderma patterns from non-scleroderma patterns.ObjectivesTo validate the “fast track algorithm” taught via an online teaching platform to identify scleroderma patterns from non-scleroderma patterns on NFC.MethodsTrainees participated in the NFC teaching workshops conducted via an online platform by the same SSc expert (AL) over 3 separate sessions. In the first part of the workshop (training), all trainees attended a talk on NFC by the SSc expert (AL) whereby they were taught the fast track algorithm to identify and classify NFC image patterns using a training set of images. The “fast track algorithm” (Figure 1) consists of three easy rules: 1) Rule number 1: the presence of ≥ 7 capillaries (capillary density) AND the absence of giant capillaries (capillary dimension) allows the rater to call the capillaroscopic image a “non-scleroderma pattern (category 1)”; 2) Rule number 2: the presence of giant capillaries or the presence of an extremely lowered capillary density (≤ 3 capillaries) in combination with abnormal shapes (= “late” scleroderma pattern) allows the rater to call the capillaroscopic image a “scleroderma pattern (category 2)”; 3) Rule number 3: if the image does not meet rule number 1 or rule number 2 then the image is automatically classified as a “non-scleroderma pattern (category 1)”. In the second part of the workshop (evaluation), each trainee independently rated 45 NFC images (30 seconds for each NFC image) in the same session, and submitted their answers in real time using an electronic form. The reading of the expert (AL) was considered the gold standard. AL is a SSc expert who had previously attended the EULAR workshop on NFC and has had more than 10 years of experience in NFC.Figure 1.Fast Track algorithmWithin 2-4 weeks of the initial rating exercise, trainees re-evaluated the same set of images for the intra-reader exercise under supervised conditions via the online platform.We computed the inter- and intra-rater agreement using mean [standard deviation (SD)] Cohen’s Kappa, with the following values representing the degrees of agreement: <0 –poor; 0-0.20 –slight; 0.21-0.40 –fair; 0.41-0.60 –moderate; 0.61-0.80 –substantial; and 0.81-1.00 –almost perfect agreement.ResultsNinety-eight trainees from Singapore, Malaysia, Indonesia, Hong Kong, Philippines, Taiwan, Myanmar and Brunei included rheumatologists, rheumatology trainees, pediatric rheumatologists, internists and nurses. Fifty-four participants were novices with no prior NFC experience, 38 participants were “moderately experienced” (< 5 years of NFC experience) and 1 participant was “experienced” with more than 5 years of NFC experience.The mean (SD) inter-rater kappa of the 98 trainees was 0.71 (0.13). For the 61 trainees who participated in the re-evaluation session, mean (SD) inter-rater and intra-rater kappa were 0.74 (0.16) and 0.71 (0.15), respectively.ConclusionThere was substantial inter- and intra-rater agreement. This is the first study in Asia examining NFC training using the EULAR SG MC/RD fast track algorithm and our results support the importance of standardized teaching for NFC.ReferencesNilFrom: Smith V, Vanhaecke A, Herrick AL, et al. EULAR Study Group on Microcirculation in Rheumatic Diseases. Fast track algorithm: How to differentiate a “scleroderma pattern” from a “non-scleroderm pattern”. Autoimmun Rev. 2019 Nov;18(11):102394.Disclosure of InterestsNone declared
更多查看译文
AI 理解论文
溯源树
样例
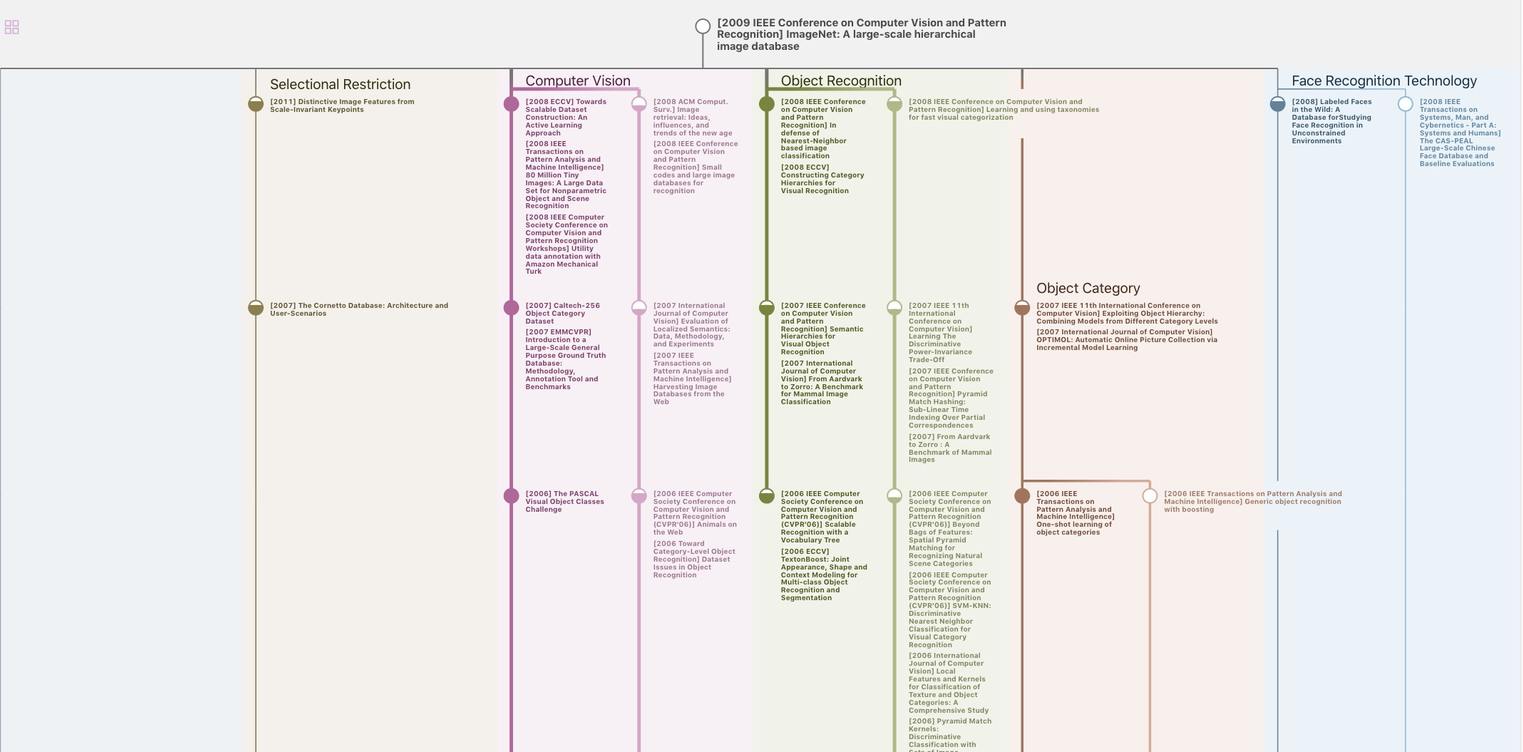
生成溯源树,研究论文发展脉络
Chat Paper
正在生成论文摘要