Topology Reconfiguration Using Neural Networks
Machine Learning for Solar Array Monitoring, Optimization, and Control(2020)
摘要
The production of PV energy is affected by external conditions such as partial shading, varying temperatures, and soiling of PV panels. Among these, partial shading causes a significant reduction in power. On the other hand, the energy production can also be affected if a PV array system has faulty modules [13] as discussed in Chapter 3. Partial shading although temporary causes voltage and current mismatch losses and can cause a significant reduction in the power supplied to the grid thereby limiting the performance of the PV array [92, 93]. The previous chapters (Chapter 3 and 4) dealt with fault detection and shading prediction. In addition to these tasks, another desirable feature in solar monitoring includes, reconfiguring the panel wiring to form different topologies or circuit connections to allow the PV array systems to produce increased power output under shading conditions. Reconfiguring the connections between the panels in a PV array is a powerful strategy to mitigate the impact of partial shading. Conventionally, utility-scale and roof-top PV arrays are connected in fixed topologies for example in Series-Parallel (SP) [13] where a fixed number of PV panels are connected in series and parallel to form an array. These fixed PV array system topologies were determined taking into consideration the weather condition for the entire year. This design does not provide the maximum power levels for a given day or season. A fixed topology is unable to bypass failed or under-performing modules, reducing the overall array output. To improve the array output power, reconfigurable systems that can change their topology need to be developed.
更多查看译文
关键词
neural networks
AI 理解论文
溯源树
样例
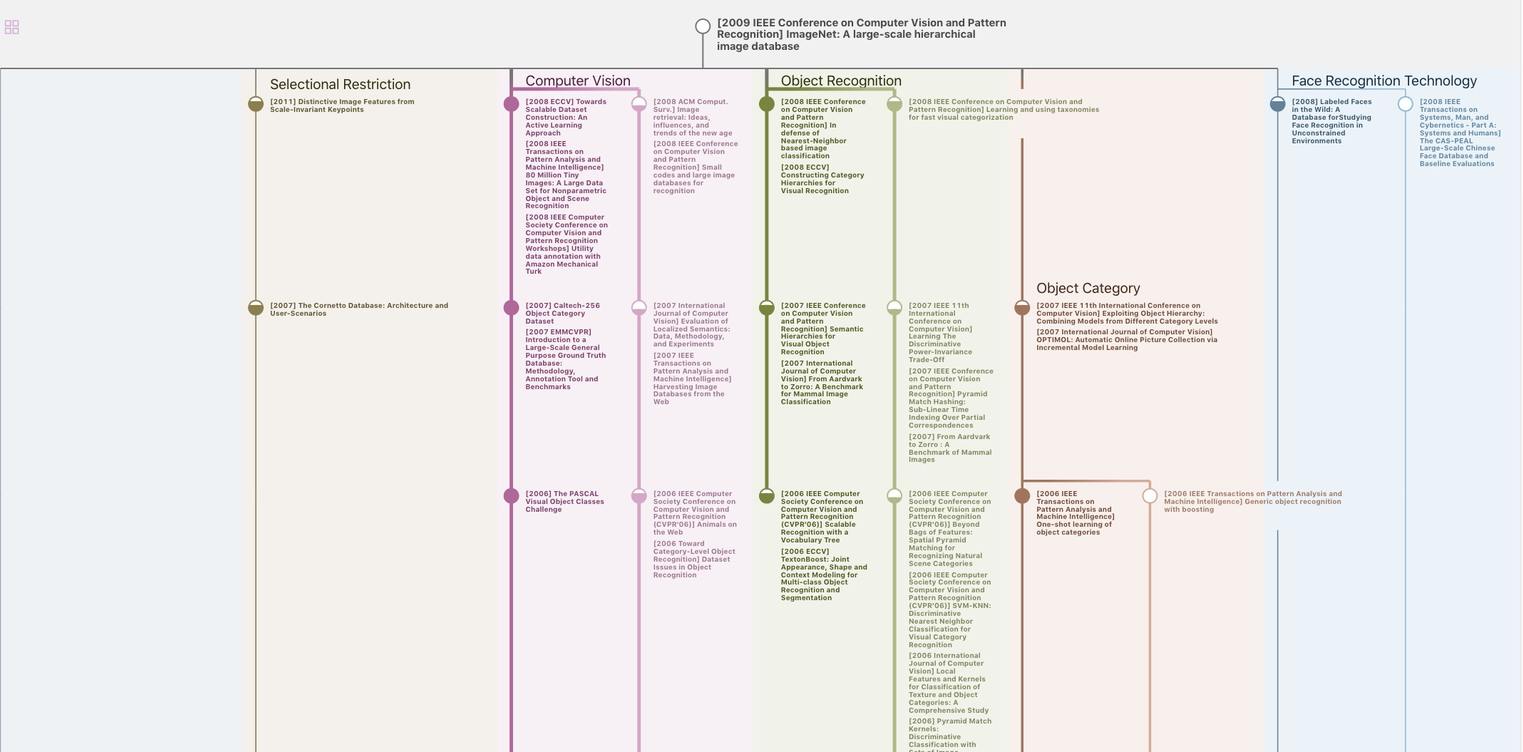
生成溯源树,研究论文发展脉络
Chat Paper
正在生成论文摘要